2024-03-31 更新
TriAug: Out-of-Distribution Detection for Imbalanced Breast Lesion in Ultrasound
Authors:Yinyu Ye, Shijing Chen, Dong Ni, Ruobing Huang
Different diseases, such as histological subtypes of breast lesions, have severely varying incidence rates. Even trained with substantial amount of in-distribution (ID) data, models often encounter out-of-distribution (OOD) samples belonging to unseen classes in clinical reality. To address this, we propose a novel framework built upon a long-tailed OOD detection task for breast ultrasound images. It is equipped with a triplet state augmentation (TriAug) which improves ID classification accuracy while maintaining a promising OOD detection performance. Meanwhile, we designed a balanced sphere loss to handle the class imbalanced problem. Experimental results show that the model outperforms state-of-art OOD approaches both in ID classification (F1-score=42.12%) and OOD detection (AUROC=78.06%).
PDF
点此查看论文截图
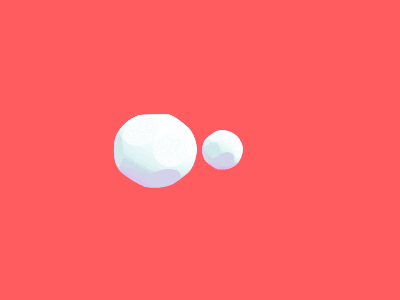
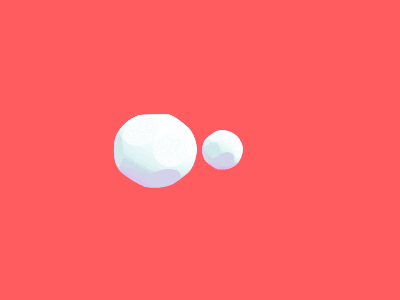
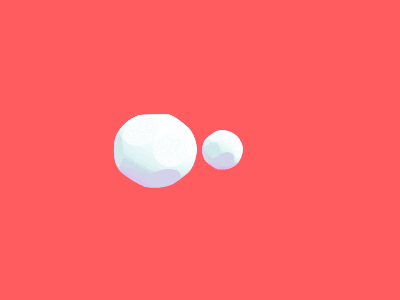
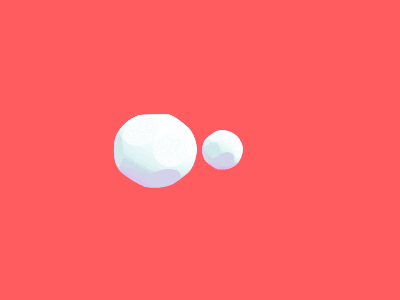
Towards Out-of-Distribution Detection for breast cancer classification in Point-of-Care Ultrasound Imaging
Authors:Jennie Karlsson, Marisa Wodrich, Niels Christian Overgaard, Freja Sahlin, Kristina Lång, Anders Heyden, Ida Arvidsson
Deep learning has shown to have great potential in medical applications. In critical domains as such, it is of high interest to have trustworthy algorithms which are able to tell when reliable assessments cannot be guaranteed. Detecting out-of-distribution (OOD) samples is a crucial step towards building a safe classifier. Following a previous study, showing that it is possible to classify breast cancer in point-of-care ultrasound images, this study investigates OOD detection using three different methods: softmax, energy score and deep ensembles. All methods are tested on three different OOD data sets. The results show that the energy score method outperforms the softmax method, performing well on two of the data sets. The ensemble method is the most robust, performing the best at detecting OOD samples for all three OOD data sets.
PDF
点此查看论文截图
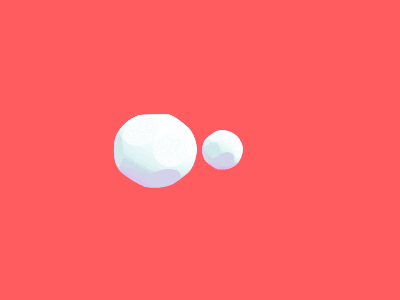
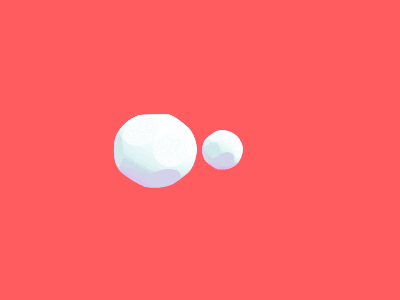
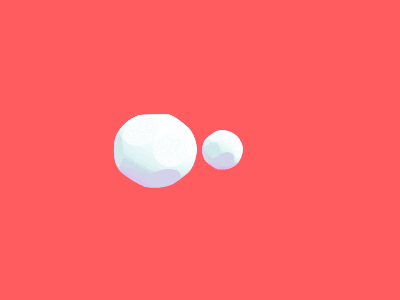
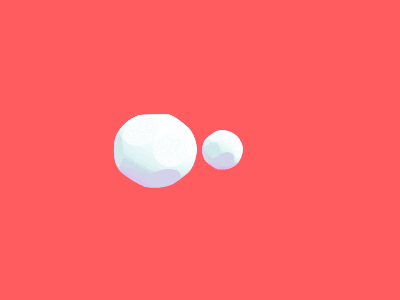
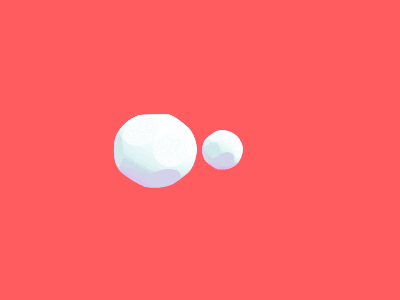
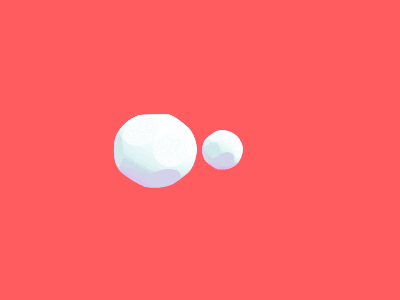
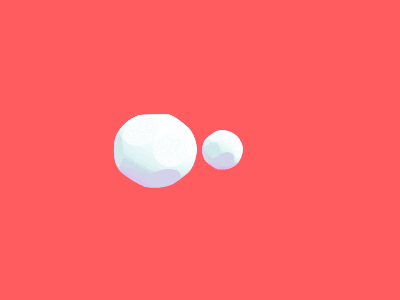