2024-01-18 更新
Contrastive Learning with Negative Sampling Correction
Authors:Lu Wang, Chao Du, Pu Zhao, Chuan Luo, Zhangchi Zhu, Bo Qiao, Wei Zhang, Qingwei Lin, Saravan Rajmohan, Dongmei Zhang, Qi Zhang
As one of the most effective self-supervised representation learning methods, contrastive learning (CL) relies on multiple negative pairs to contrast against each positive pair. In the standard practice of contrastive learning, data augmentation methods are utilized to generate both positive and negative pairs. While existing works have been focusing on improving the positive sampling, the negative sampling process is often overlooked. In fact, the generated negative samples are often polluted by positive samples, which leads to a biased loss and performance degradation. To correct the negative sampling bias, we propose a novel contrastive learning method named Positive-Unlabeled Contrastive Learning (PUCL). PUCL treats the generated negative samples as unlabeled samples and uses information from positive samples to correct bias in contrastive loss. We prove that the corrected loss used in PUCL only incurs a negligible bias compared to the unbiased contrastive loss. PUCL can be applied to general contrastive learning problems and outperforms state-of-the-art methods on various image and graph classification tasks. The code of PUCL is in the supplementary file.
PDF 9 pages, 3 figures
点此查看论文截图
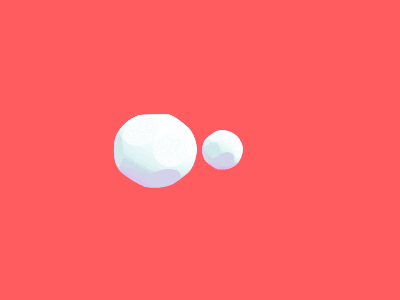
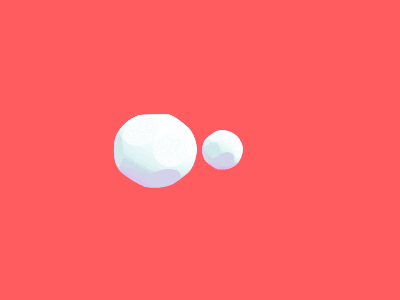
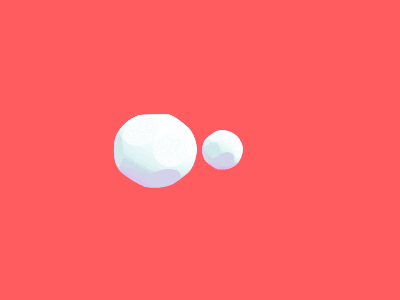
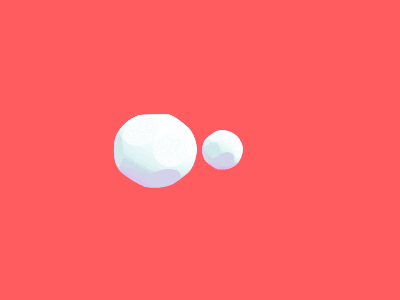
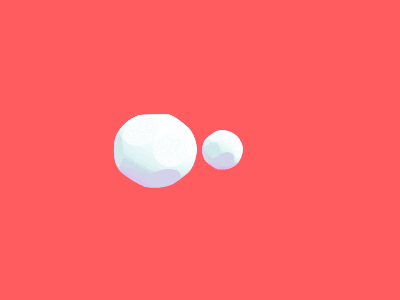
CrossVideo: Self-supervised Cross-modal Contrastive Learning for Point Cloud Video Understanding
Authors:Yunze Liu, Changxi Chen, Zifan Wang, Li Yi
This paper introduces a novel approach named CrossVideo, which aims to enhance self-supervised cross-modal contrastive learning in the field of point cloud video understanding. Traditional supervised learning methods encounter limitations due to data scarcity and challenges in label acquisition. To address these issues, we propose a self-supervised learning method that leverages the cross-modal relationship between point cloud videos and image videos to acquire meaningful feature representations. Intra-modal and cross-modal contrastive learning techniques are employed to facilitate effective comprehension of point cloud video. We also propose a multi-level contrastive approach for both modalities. Through extensive experiments, we demonstrate that our method significantly surpasses previous state-of-the-art approaches, and we conduct comprehensive ablation studies to validate the effectiveness of our proposed designs.
PDF
点此查看论文截图
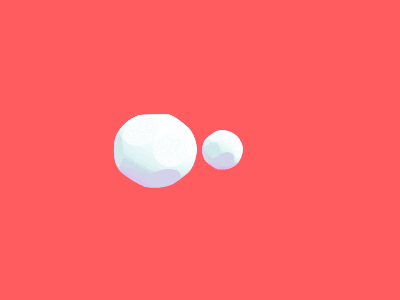
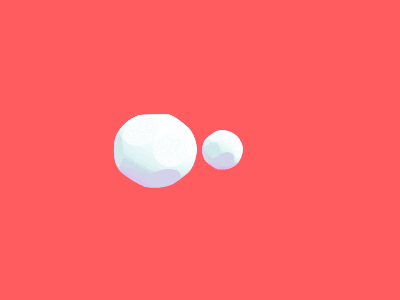
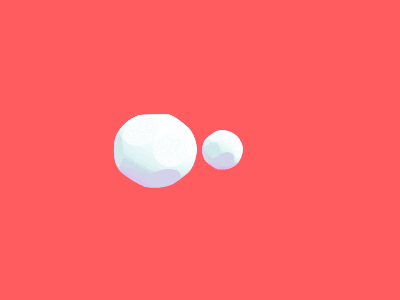
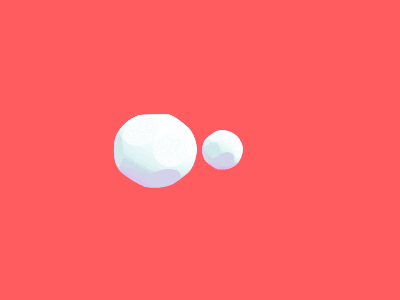
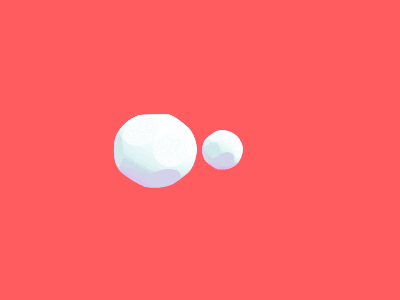
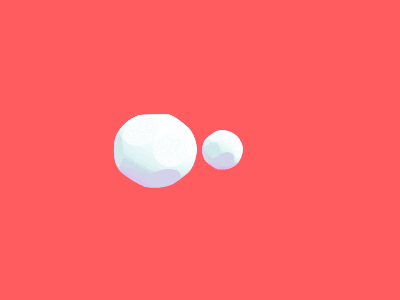
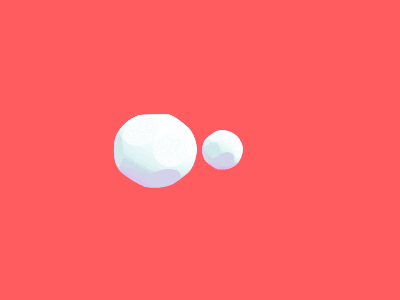