2023-11-27 更新
Sparsity-Driven EEG Channel Selection for Brain-Assisted Speech Enhancement
Authors:Jie Zhang, Qing-Tian Xu, Zhen-Hua Ling
Speech enhancement is widely used as a front-end to improve the speech quality in many audio systems, while it is still hard to extract the target speech in multi-talker conditions without prior information on the speaker identity. It was shown by auditory attention decoding that the attended speaker can be revealed by the electroencephalogram (EEG) of the listener implicitly. In this work, we therefore propose a novel end-to-end brain-assisted speech enhancement network (BASEN), which incorporates the listeners’ EEG signals and adopts a temporal convolutional network together with a convolutional multi-layer cross attention module to fuse EEG-audio features. Considering that an EEG cap with sparse channels exhibits multiple benefits and in practice many electrodes might contribute marginally, we further propose two channel selection methods, called residual Gumbel selection and convolutional regularization selection. They are dedicated to tackling the issues of training instability and duplicated channel selections, respectively. Experimental results on a public dataset show the superiority of the proposed baseline BASEN over existing approaches. The proposed channel selection methods can significantly reduce the amount of informative EEG channels with a negligible impact on the performance.
PDF arXiv admin note: text overlap with arXiv:2305.09994
点此查看论文截图
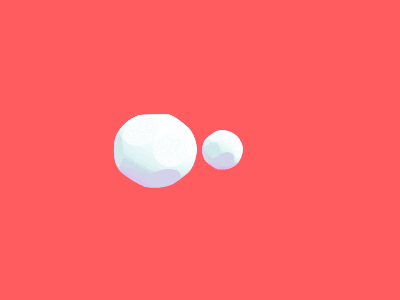
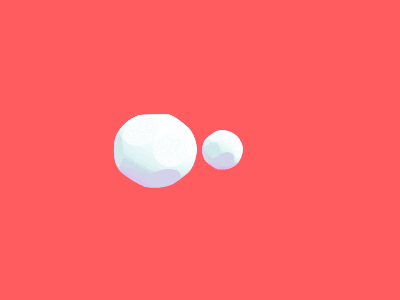
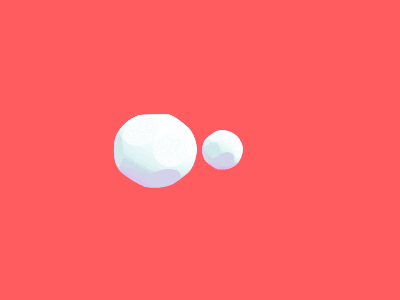
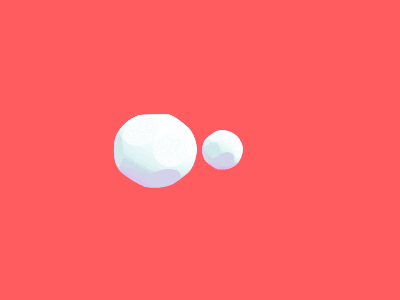
End-to-end Transfer Learning for Speaker-independent Cross-language Speech Emotion Recognition
Authors:Duowei Tang, Peter Kuppens, Luc Geurts, Toon van Waterschoot
Data-driven models achieve successful results in Speech Emotion Recognition (SER). However, these models, which are based on general acoustic features or end-to-end approaches, show poor performance when the testing set has a different language (i.e., the cross-language setting) than the training set or when they come from a different dataset (i.e., the cross-corpus setting). To alleviate this problem, this paper presents an end-to-end Deep Neural Network (DNN) model based on transfer learning for cross-language SER. We use the wav2vec 2.0 pre-trained model to transform audio time-domain waveforms from different languages, different speakers and different recording conditions into a feature space shared by multiple languages, thereby it reduces the language variabilities in the speech features. Next, we propose a new Deep-Within-Class Co-variance Normalisation (Deep-WCCN) layer that can be inserted into the DNN model and it aims to reduce other variabilities including speaker variability, channel variability and so on. The whole model is fine-tuned in an end-to-end manner on a combined loss and is validated on datasets from three languages (i.e., English, German, Chinese). Experiment results show that our proposed method not only outperforms the baseline model that is based on common acoustic feature sets for SER in the within-language setting, but also significantly outperforms the baseline model for cross-language setting. In addition, we also experimentally validate the effectiveness of Deep-WCCN, which can further improve the model performance. Finally, to comparing the results in the recent literatures that use the same testing datasets, our proposed model shows significantly better performance than other state-of-the-art models in cross-language SER.
PDF 15 pages, 6 figures, 4 tables
点此查看论文截图
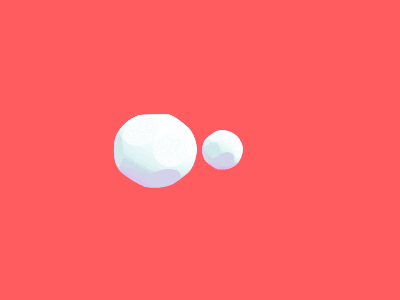
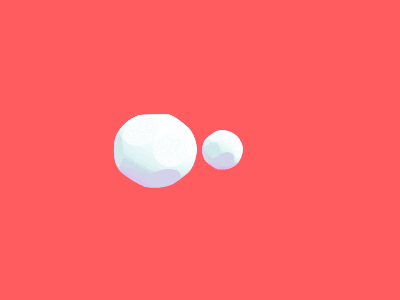
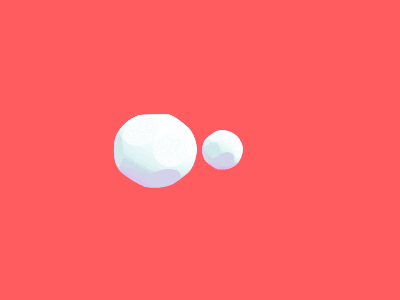
SER_AMPEL: A multi-source dataset for SER of Italian older adults
Authors:Alessandra Grossi, Francesca Gasparini
In this paper, SER_AMPEL, a multi-source dataset for speech emotion recognition (SER) is presented. The peculiarity of the dataset is that it is collected with the aim of providing a reference for speech emotion recognition in case of Italian older adults. The dataset is collected following different protocols, in particular considering acted conversations, extracted from movies and TV series, and recording natural conversations where the emotions are elicited by proper questions. The evidence of the need for such a dataset emerges from the analysis of the state of the art. Preliminary considerations on the critical issues of SER are reported analyzing the classification results on a subset of the proposed dataset.
PDF 11 pages, 1 Figure, 7 Tables, submitted to ForItAAL 2023 (12{\deg} Forum Italiano Ambient Assisted Living)
点此查看论文截图
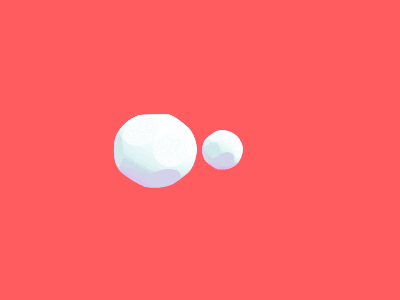
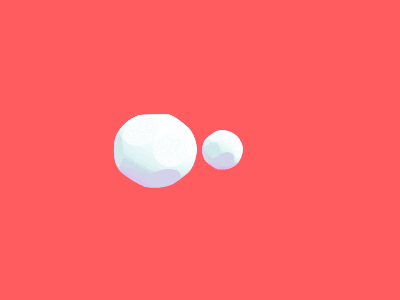
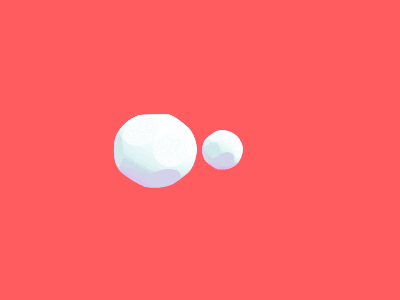
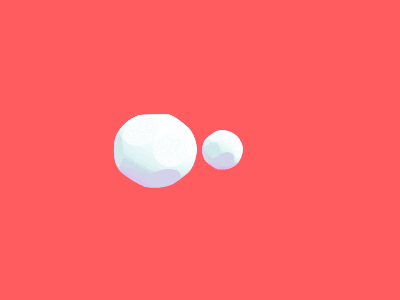
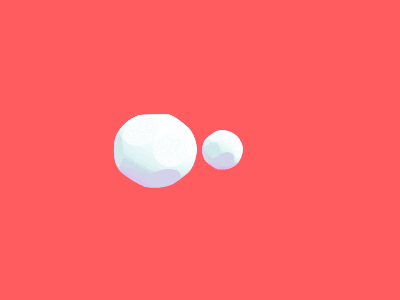