2023-09-02 更新
MixNet: Toward Accurate Detection of Challenging Scene Text in the Wild
Authors:Yu-Xiang Zeng, Jun-Wei Hsieh, Xin Li, Ming-Ching Chang
Detecting small scene text instances in the wild is particularly challenging, where the influence of irregular positions and nonideal lighting often leads to detection errors. We present MixNet, a hybrid architecture that combines the strengths of CNNs and Transformers, capable of accurately detecting small text from challenging natural scenes, regardless of the orientations, styles, and lighting conditions. MixNet incorporates two key modules: (1) the Feature Shuffle Network (FSNet) to serve as the backbone and (2) the Central Transformer Block (CTBlock) to exploit the 1D manifold constraint of the scene text. We first introduce a novel feature shuffling strategy in FSNet to facilitate the exchange of features across multiple scales, generating high-resolution features superior to popular ResNet and HRNet. The FSNet backbone has achieved significant improvements over many existing text detection methods, including PAN, DB, and FAST. Then we design a complementary CTBlock to leverage center line based features similar to the medial axis of text regions and show that it can outperform contour-based approaches in challenging cases when small scene texts appear closely. Extensive experimental results show that MixNet, which mixes FSNet with CTBlock, achieves state-of-the-art results on multiple scene text detection datasets.
PDF
点此查看论文截图
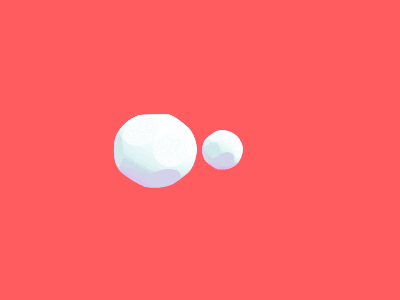
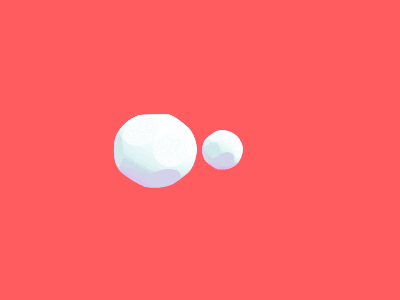
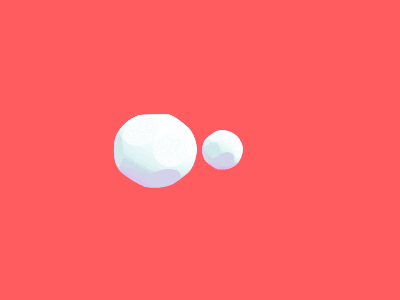
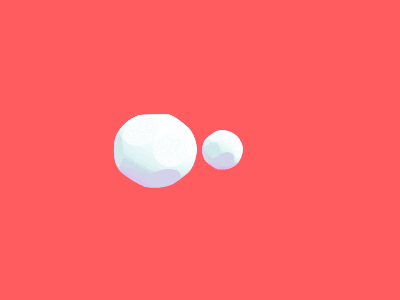
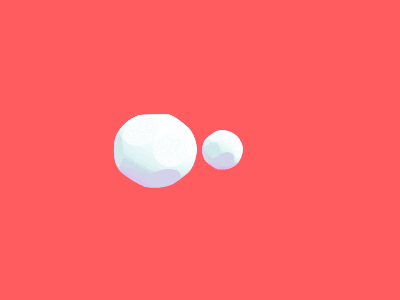
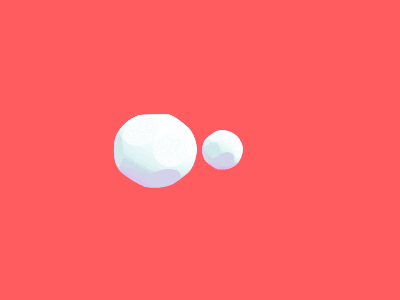
PBFormer: Capturing Complex Scene Text Shape with Polynomial Band Transformer
Authors:Ruijin Liu, Ning Lu, Dapeng Chen, Cheng Li, Zejian Yuan, Wei Peng
We present PBFormer, an efficient yet powerful scene text detector that unifies the transformer with a novel text shape representation Polynomial Band (PB). The representation has four polynomial curves to fit a text’s top, bottom, left, and right sides, which can capture a text with a complex shape by varying polynomial coefficients. PB has appealing features compared with conventional representations: 1) It can model different curvatures with a fixed number of parameters, while polygon-points-based methods need to utilize a different number of points. 2) It can distinguish adjacent or overlapping texts as they have apparent different curve coefficients, while segmentation-based or points-based methods suffer from adhesive spatial positions. PBFormer combines the PB with the transformer, which can directly generate smooth text contours sampled from predicted curves without interpolation. A parameter-free cross-scale pixel attention (CPA) module is employed to highlight the feature map of a suitable scale while suppressing the other feature maps. The simple operation can help detect small-scale texts and is compatible with the one-stage DETR framework, where no postprocessing exists for NMS. Furthermore, PBFormer is trained with a shape-contained loss, which not only enforces the piecewise alignment between the ground truth and the predicted curves but also makes curves’ positions and shapes consistent with each other. Without bells and whistles about text pre-training, our method is superior to the previous state-of-the-art text detectors on the arbitrary-shaped text datasets.
PDF 9 pages, 8 figures, accepted by ACM MM 2023
点此查看论文截图
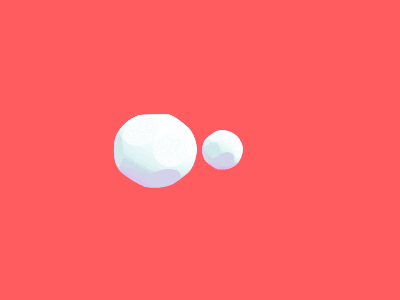
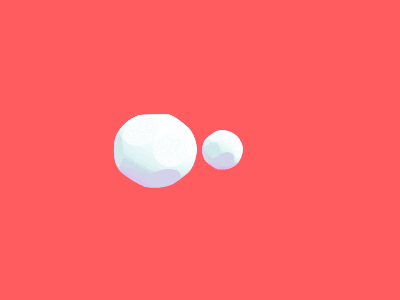
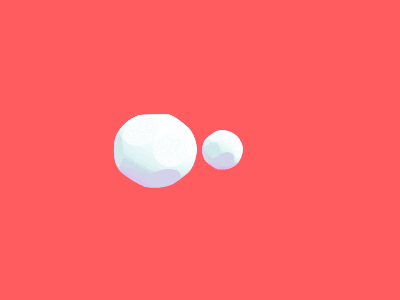
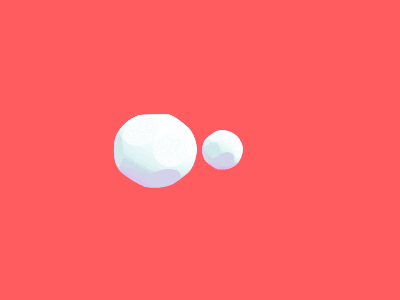
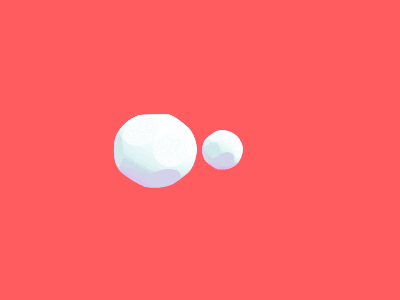
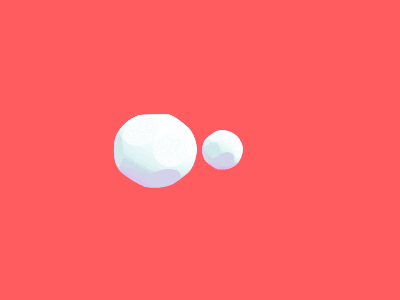
Text2Scene: Text-driven Indoor Scene Stylization with Part-aware Details
Authors:Inwoo Hwang, Hyeonwoo Kim, Young Min Kim
We propose Text2Scene, a method to automatically create realistic textures for virtual scenes composed of multiple objects. Guided by a reference image and text descriptions, our pipeline adds detailed texture on labeled 3D geometries in the room such that the generated colors respect the hierarchical structure or semantic parts that are often composed of similar materials. Instead of applying flat stylization on the entire scene at a single step, we obtain weak semantic cues from geometric segmentation, which are further clarified by assigning initial colors to segmented parts. Then we add texture details for individual objects such that their projections on image space exhibit feature embedding aligned with the embedding of the input. The decomposition makes the entire pipeline tractable to a moderate amount of computation resources and memory. As our framework utilizes the existing resources of image and text embedding, it does not require dedicated datasets with high-quality textures designed by skillful artists. To the best of our knowledge, it is the first practical and scalable approach that can create detailed and realistic textures of the desired style that maintain structural context for scenes with multiple objects.
PDF Accepted to CVPR 2023
点此查看论文截图
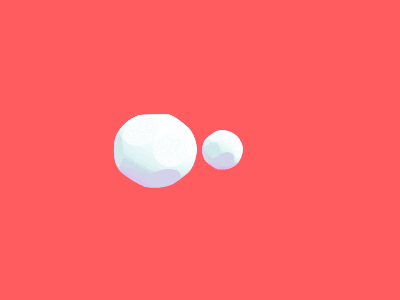
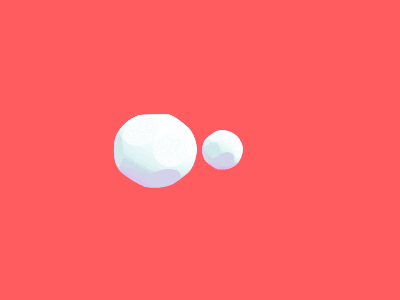
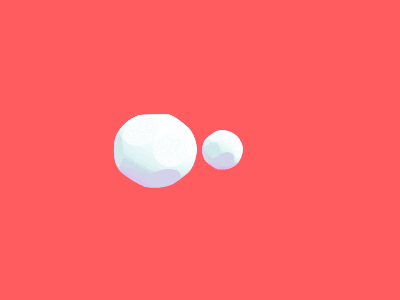
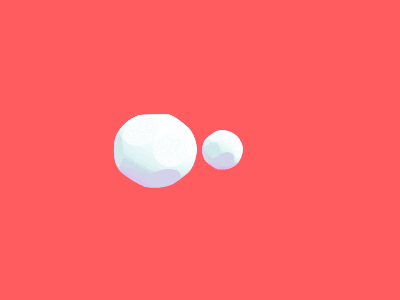
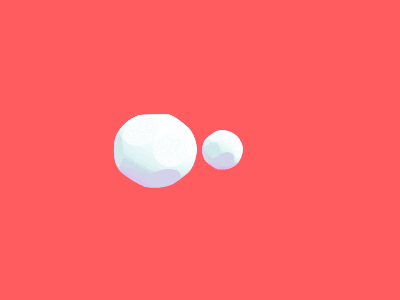