2023-08-29 更新
A Systematic Study on Quantifying Bias in GAN-Augmented Data
Authors:Denis Liu
Generative adversarial networks (GANs) have recently become a popular data augmentation technique used by machine learning practitioners. However, they have been shown to suffer from the so-called mode collapse failure mode, which makes them vulnerable to exacerbating biases on already skewed datasets, resulting in the generated data distribution being less diverse than the training distribution. To this end, we address the problem of quantifying the extent to which mode collapse occurs. This study is a systematic effort focused on the evaluation of state-of-the-art metrics that can potentially quantify biases in GAN-augmented data. We show that, while several such methods are available, there is no single metric that quantifies bias exacerbation reliably over the span of different image domains.
PDF
点此查看论文截图
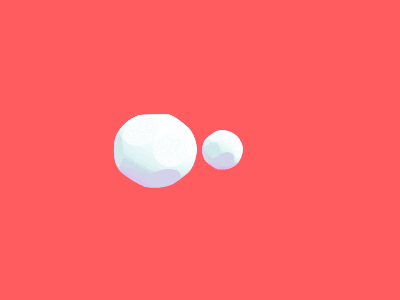
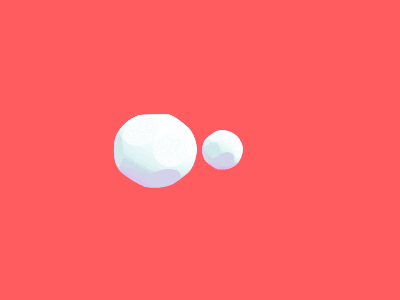
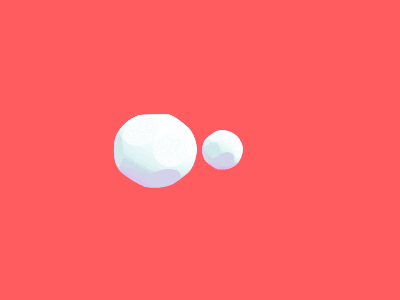