2023-08-26 更新
Joint one-sided synthetic unpaired image translation and segmentation for colorectal cancer prevention
Authors:Enric Moreu, Eric Arazo, Kevin McGuinness, Noel E. O’Connor
Deep learning has shown excellent performance in analysing medical images. However, datasets are difficult to obtain due privacy issues, standardization problems, and lack of annotations. We address these problems by producing realistic synthetic images using a combination of 3D technologies and generative adversarial networks. We propose CUT-seg, a joint training where a segmentation model and a generative model are jointly trained to produce realistic images while learning to segment polyps. We take advantage of recent one-sided translation models because they use significantly less memory, allowing us to add a segmentation model in the training loop. CUT-seg performs better, is computationally less expensive, and requires less real images than other memory-intensive image translation approaches that require two stage training. Promising results are achieved on five real polyp segmentation datasets using only one real image and zero real annotations. As a part of this study we release Synth-Colon, an entirely synthetic dataset that includes 20000 realistic colon images and additional details about depth and 3D geometry: https://enric1994.github.io/synth-colon
PDF arXiv admin note: substantial text overlap with arXiv:2202.08680
点此查看论文截图
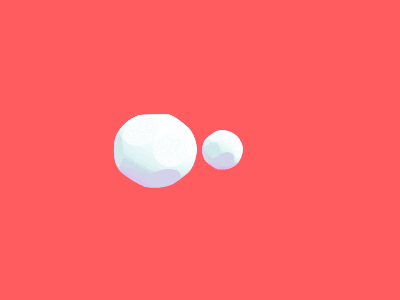
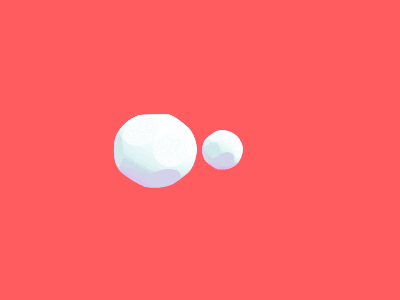
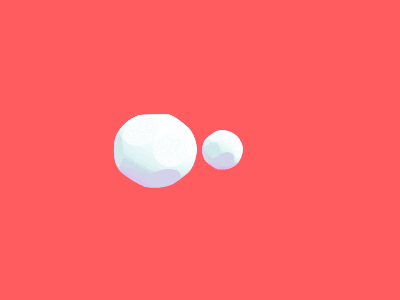
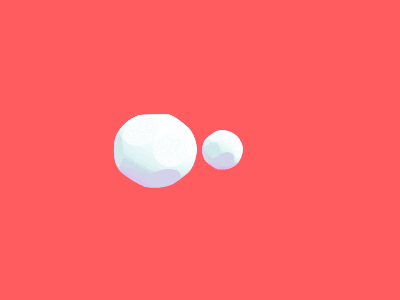
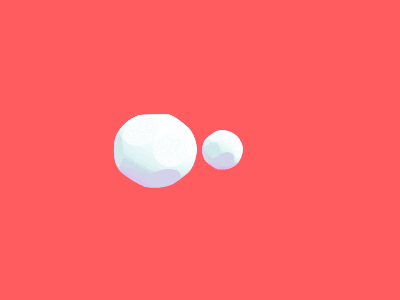
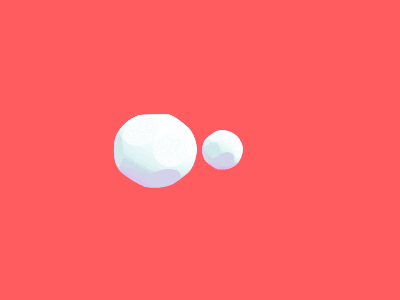
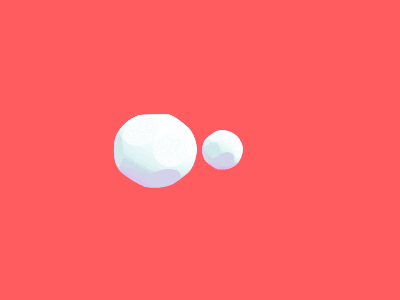
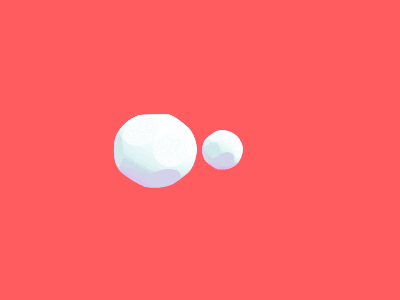
General Image-to-Image Translation with One-Shot Image Guidance
Authors:Bin Cheng, Zuhao Liu, Yunbo Peng, Yue Lin
Large-scale text-to-image models pre-trained on massive text-image pairs show excellent performance in image synthesis recently. However, image can provide more intuitive visual concepts than plain text. People may ask: how can we integrate the desired visual concept into an existing image, such as our portrait? Current methods are inadequate in meeting this demand as they lack the ability to preserve content or translate visual concepts effectively. Inspired by this, we propose a novel framework named visual concept translator (VCT) with the ability to preserve content in the source image and translate the visual concepts guided by a single reference image. The proposed VCT contains a content-concept inversion (CCI) process to extract contents and concepts, and a content-concept fusion (CCF) process to gather the extracted information to obtain the target image. Given only one reference image, the proposed VCT can complete a wide range of general image-to-image translation tasks with excellent results. Extensive experiments are conducted to prove the superiority and effectiveness of the proposed methods. Codes are available at https://github.com/CrystalNeuro/visual-concept-translator.
PDF accepted by ICCV 2023
点此查看论文截图
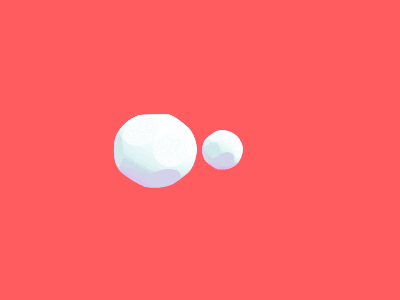
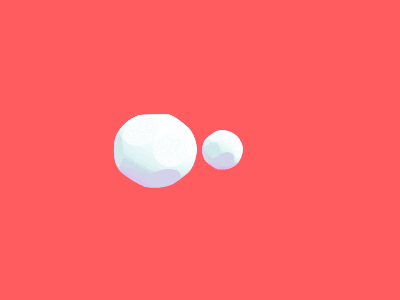
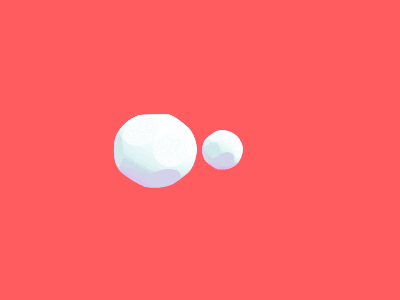
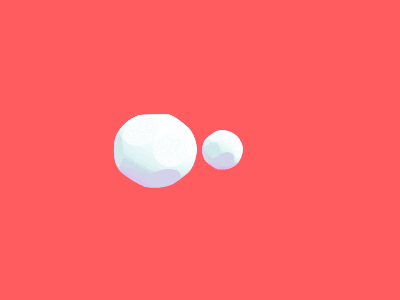
UCDFormer: Unsupervised Change Detection Using a Transformer-driven Image Translation
Authors:Qingsong Xu, Yilei Shi, Jianhua Guo, Chaojun Ouyang, Xiao Xiang Zhu
Change detection (CD) by comparing two bi-temporal images is a crucial task in remote sensing. With the advantages of requiring no cumbersome labeled change information, unsupervised CD has attracted extensive attention in the community. However, existing unsupervised CD approaches rarely consider the seasonal and style differences incurred by the illumination and atmospheric conditions in multi-temporal images. To this end, we propose a change detection with domain shift setting for remote sensing images. Furthermore, we present a novel unsupervised CD method using a light-weight transformer, called UCDFormer. Specifically, a transformer-driven image translation composed of a light-weight transformer and a domain-specific affinity weight is first proposed to mitigate domain shift between two images with real-time efficiency. After image translation, we can generate the difference map between the translated before-event image and the original after-event image. Then, a novel reliable pixel extraction module is proposed to select significantly changed/unchanged pixel positions by fusing the pseudo change maps of fuzzy c-means clustering and adaptive threshold. Finally, a binary change map is obtained based on these selected pixel pairs and a binary classifier. Experimental results on different unsupervised CD tasks with seasonal and style changes demonstrate the effectiveness of the proposed UCDFormer. For example, compared with several other related methods, UCDFormer improves performance on the Kappa coefficient by more than 12\%. In addition, UCDFormer achieves excellent performance for earthquake-induced landslide detection when considering large-scale applications. The code is available at \url{https://github.com/zhu-xlab/UCDFormer}
PDF 16 pages, 7 figures, IEEE Transactions on Geoscience and Remote Sensing
点此查看论文截图
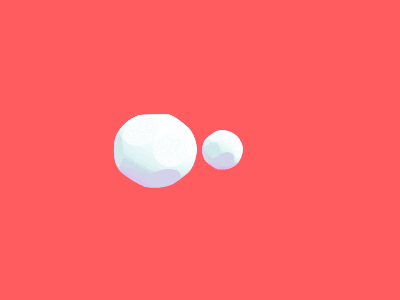
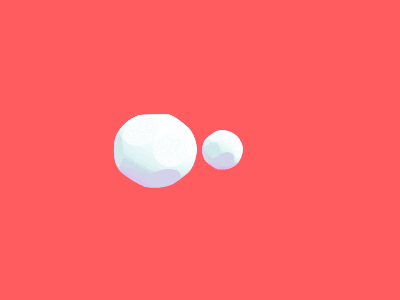
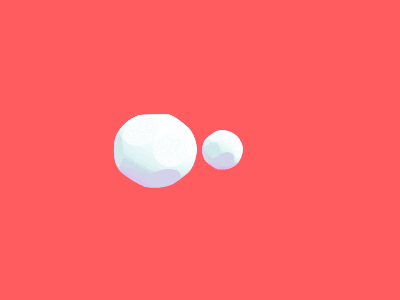
SDDM: Score-Decomposed Diffusion Models on Manifolds for Unpaired Image-to-Image Translation
Authors:Shikun Sun, Longhui Wei, Junliang Xing, Jia Jia, Qi Tian
Recent score-based diffusion models (SBDMs) show promising results in unpaired image-to-image translation (I2I). However, existing methods, either energy-based or statistically-based, provide no explicit form of the interfered intermediate generative distributions. This work presents a new score-decomposed diffusion model (SDDM) on manifolds to explicitly optimize the tangled distributions during image generation. SDDM derives manifolds to make the distributions of adjacent time steps separable and decompose the score function or energy guidance into an image denoising" part and a content
refinement” part. To refine the image in the same noise level, we equalize the refinement parts of the score function and energy guidance, which permits multi-objective optimization on the manifold. We also leverage the block adaptive instance normalization module to construct manifolds with lower dimensions but still concentrated with the perturbed reference image. SDDM outperforms existing SBDM-based methods with much fewer diffusion steps on several I2I benchmarks.
PDF
点此查看论文截图
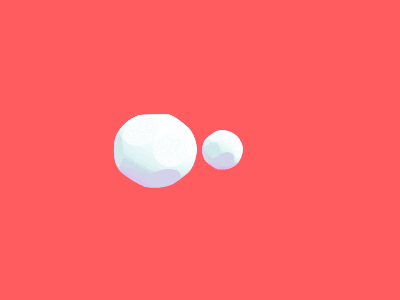
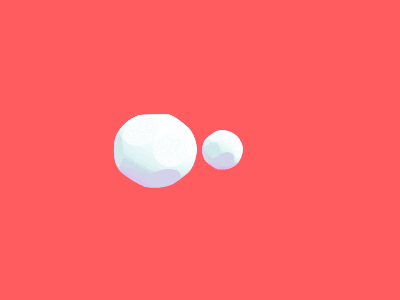
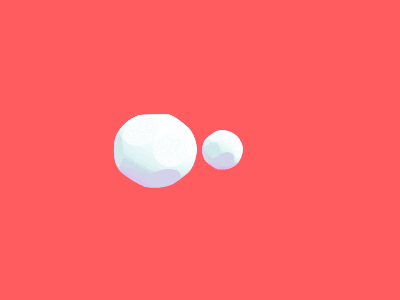
Cooperative Colorization: Exploring Latent Cross-Domain Priors for NIR Image Spectrum Translation
Authors:Xingxing Yang, Jie Chen, Zaifeng Yang
Near-infrared (NIR) image spectrum translation is a challenging problem with many promising applications. Existing methods struggle with the mapping ambiguity between the NIR and the RGB domains, and generalize poorly due to the limitations of models’ learning capabilities and the unavailability of sufficient NIR-RGB image pairs for training. To address these challenges, we propose a cooperative learning paradigm that colorizes NIR images in parallel with another proxy grayscale colorization task by exploring latent cross-domain priors (i.e., latent spectrum context priors and task domain priors), dubbed CoColor. The complementary statistical and semantic spectrum information from these two task domains — in the forms of pre-trained colorization networks — are brought in as task domain priors. A bilateral domain translation module is subsequently designed, in which intermittent NIR images are generated from grayscale and colorized in parallel with authentic NIR images; and vice versa for the grayscale images. These intermittent transformations act as latent spectrum context priors for efficient domain knowledge exchange. We progressively fine-tune and fuse these modules with a series of pixel-level and feature-level consistency constraints. Experiments show that our proposed cooperative learning framework produces satisfactory spectrum translation outputs with diverse colors and rich textures, and outperforms state-of-the-art counterparts by 3.95dB and 4.66dB in terms of PNSR for the NIR and grayscale colorization tasks, respectively.
PDF Accepted by ACMMM 2023
点此查看论文截图
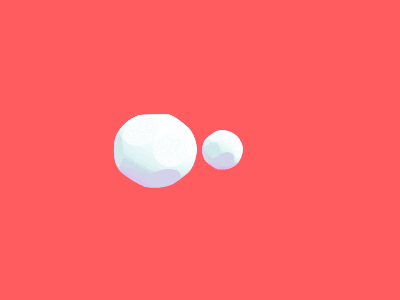
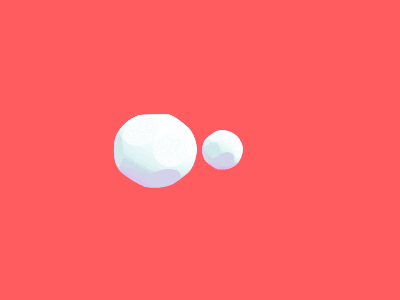
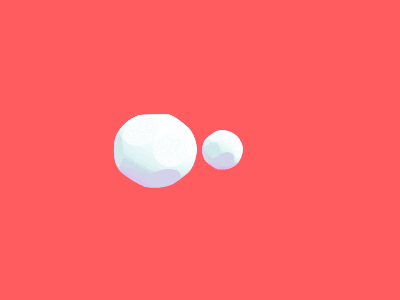
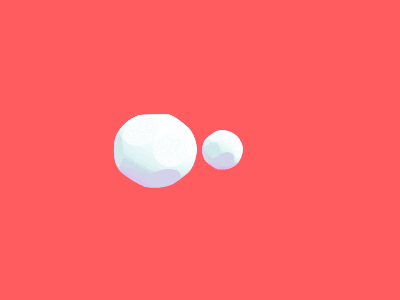
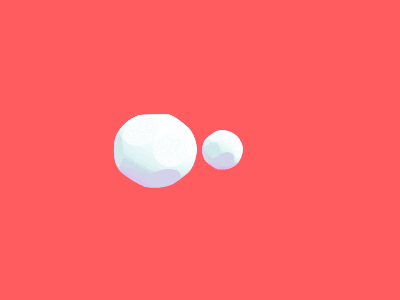
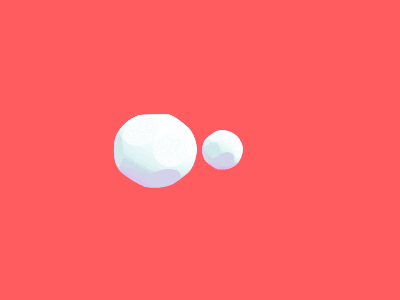
GaFET: Learning Geometry-aware Facial Expression Translation from In-The-Wild Images
Authors:Tianxiang Ma, Bingchuan Li, Qian He, Jing Dong, Tieniu Tan
While current face animation methods can manipulate expressions individually, they suffer from several limitations. The expressions manipulated by some motion-based facial reenactment models are crude. Other ideas modeled with facial action units cannot generalize to arbitrary expressions not covered by annotations. In this paper, we introduce a novel Geometry-aware Facial Expression Translation (GaFET) framework, which is based on parametric 3D facial representations and can stably decoupled expression. Among them, a Multi-level Feature Aligned Transformer is proposed to complement non-geometric facial detail features while addressing the alignment challenge of spatial features. Further, we design a De-expression model based on StyleGAN, in order to reduce the learning difficulty of GaFET in unpaired “in-the-wild” images. Extensive qualitative and quantitative experiments demonstrate that we achieve higher-quality and more accurate facial expression transfer results compared to state-of-the-art methods, and demonstrate applicability of various poses and complex textures. Besides, videos or annotated training data are omitted, making our method easier to use and generalize.
PDF Accepted by ICCV2023
点此查看论文截图
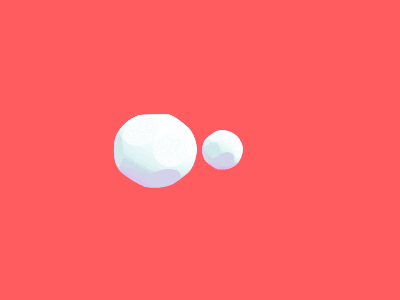
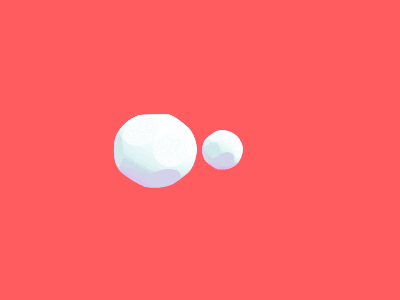
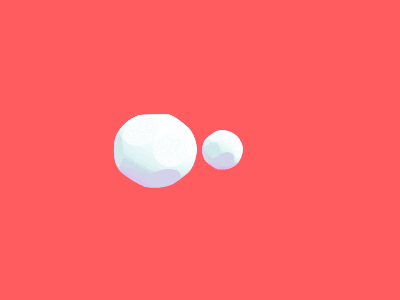
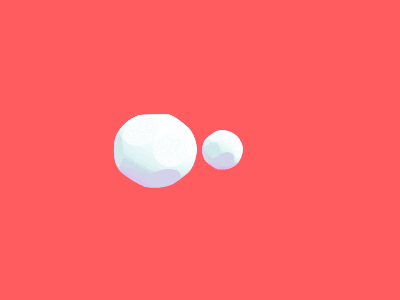
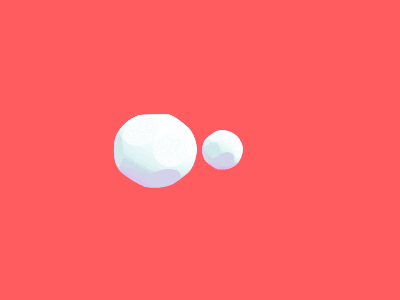
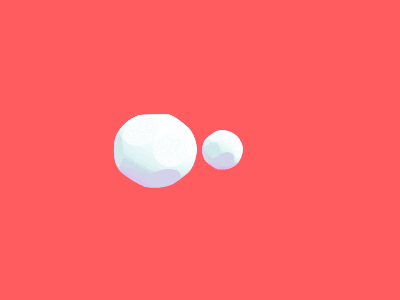
LadleNet: Translating Thermal Infrared Images to Visible Light Images Using A Scalable Two-stage U-Net
Authors:Tonghui Zou
The translation of thermal infrared (TIR) images to visible light (VI) images presents a challenging task with potential applications spanning various domains such as TIR-VI image registration and fusion. Leveraging supplementary information derived from TIR image conversions can significantly enhance model performance and generalization across these applications. However, prevailing issues within this field include suboptimal image fidelity and limited model scalability. In this paper, we introduce an algorithm, LadleNet, based on the U-Net architecture. LadleNet employs a two-stage U-Net concatenation structure, augmented with skip connections and refined feature aggregation techniques, resulting in a substantial enhancement in model performance. Comprising ‘Handle’ and ‘Bowl’ modules, LadleNet’s Handle module facilitates the construction of an abstract semantic space, while the Bowl module decodes this semantic space to yield mapped VI images. The Handle module exhibits extensibility by allowing the substitution of its network architecture with semantic segmentation networks, thereby establishing more abstract semantic spaces to bolster model performance. Consequently, we propose LadleNet+, which replaces LadleNet’s Handle module with the pre-trained DeepLabv3+ network, thereby endowing the model with enhanced semantic space construction capabilities. The proposed method is evaluated and tested on the KAIST dataset, accompanied by quantitative and qualitative analyses. Compared to existing methodologies, our approach achieves state-of-the-art performance in terms of image clarity and perceptual quality. The source code will be made available at https://github.com/Ach-1914/LadleNet/tree/main/.
PDF
点此查看论文截图
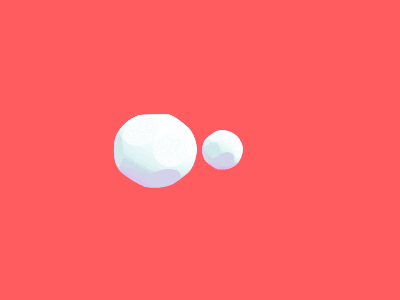
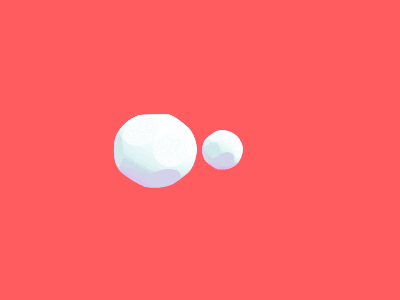
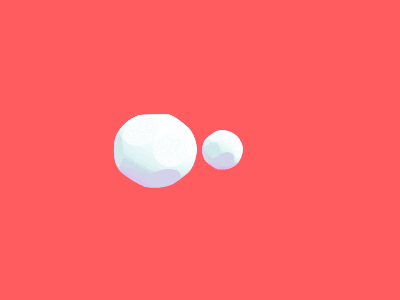
Hierarchy Flow For High-Fidelity Image-to-Image Translation
Authors:Weichen Fan, Jinghuan Chen, Ziwei Liu
Image-to-image (I2I) translation comprises a wide spectrum of tasks. Here we divide this problem into three levels: strong-fidelity translation, normal-fidelity translation, and weak-fidelity translation, indicating the extent to which the content of the original image is preserved. Although existing methods achieve good performance in weak-fidelity translation, they fail to fully preserve the content in both strong- and normal-fidelity tasks, e.g. sim2real, style transfer and low-level vision. In this work, we propose Hierarchy Flow, a novel flow-based model to achieve better content preservation during translation. Specifically, 1) we first unveil the drawbacks of standard flow-based models when applied to I2I translation. 2) Next, we propose a new design, namely hierarchical coupling for reversible feature transformation and multi-scale modeling, to constitute Hierarchy Flow. 3) Finally, we present a dedicated aligned-style loss for a better trade-off between content preservation and stylization during translation. Extensive experiments on a wide range of I2I translation benchmarks demonstrate that our approach achieves state-of-the-art performance, with convincing advantages in both strong- and normal-fidelity tasks. Code and models will be at https://github.com/WeichenFan/HierarchyFlow.
PDF arXiv admin note: text overlap with arXiv:2207.01909
点此查看论文截图
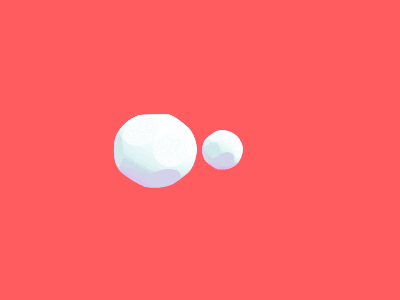
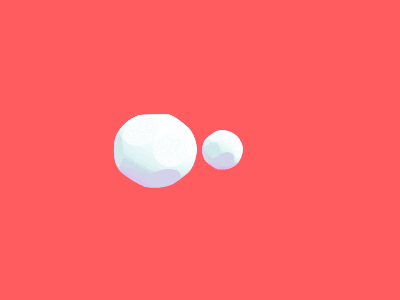
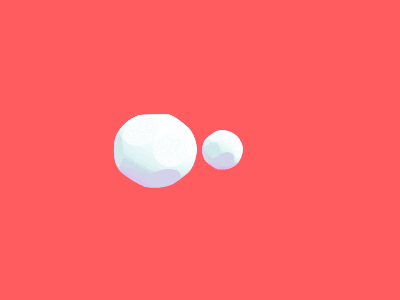
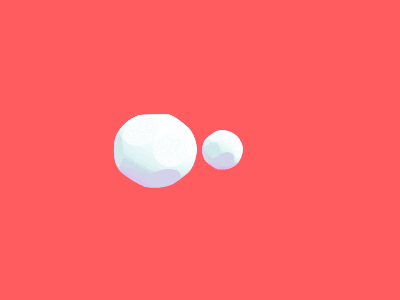
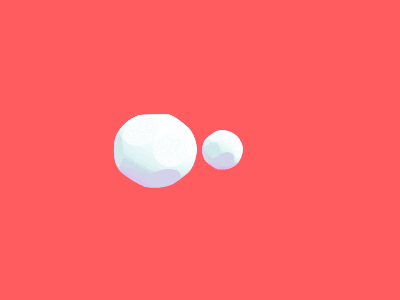
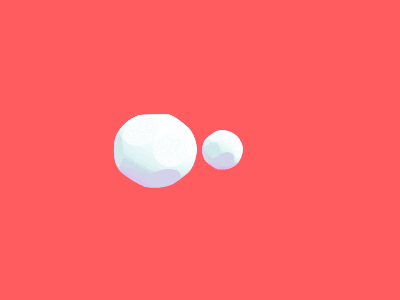
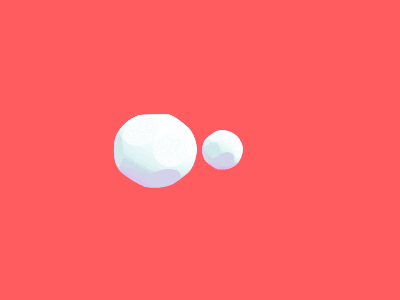
Jurassic World Remake: Bringing Ancient Fossils Back to Life via Zero-Shot Long Image-to-Image Translation
Authors:Alexander Martin, Haitian Zheng, Jie An, Jiebo Luo
With a strong understanding of the target domain from natural language, we produce promising results in translating across large domain gaps and bringing skeletons back to life. In this work, we use text-guided latent diffusion models for zero-shot image-to-image translation (I2I) across large domain gaps (longI2I), where large amounts of new visual features and new geometry need to be generated to enter the target domain. Being able to perform translations across large domain gaps has a wide variety of real-world applications in criminology, astrology, environmental conservation, and paleontology. In this work, we introduce a new task Skull2Animal for translating between skulls and living animals. On this task, we find that unguided Generative Adversarial Networks (GANs) are not capable of translating across large domain gaps. Instead of these traditional I2I methods, we explore the use of guided diffusion and image editing models and provide a new benchmark model, Revive-2I, capable of performing zero-shot I2I via text-prompting latent diffusion models. We find that guidance is necessary for longI2I because, to bridge the large domain gap, prior knowledge about the target domain is needed. In addition, we find that prompting provides the best and most scalable information about the target domain as classifier-guided diffusion models require retraining for specific use cases and lack stronger constraints on the target domain because of the wide variety of images they are trained on.
PDF 9 pages, 10 figures, ACM Multimedia 2023
点此查看论文截图
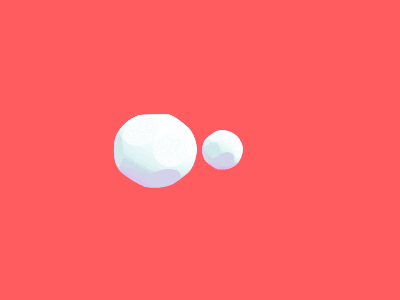
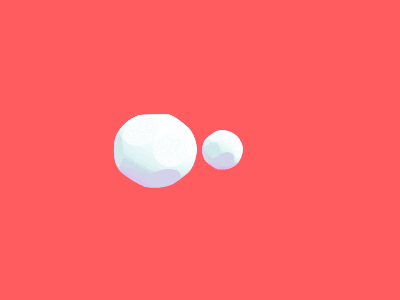
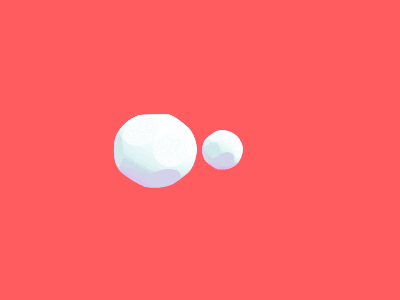
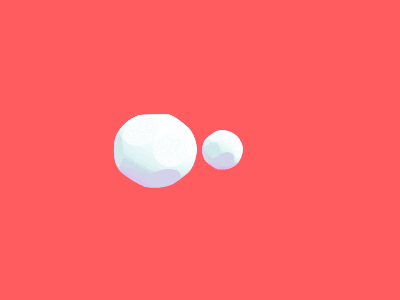
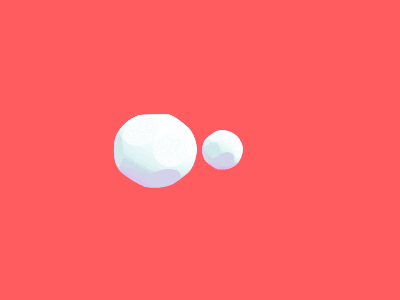
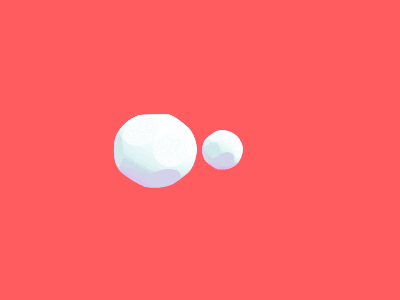
CoDeF: Content Deformation Fields for Temporally Consistent Video Processing
Authors:Hao Ouyang, Qiuyu Wang, Yuxi Xiao, Qingyan Bai, Juntao Zhang, Kecheng Zheng, Xiaowei Zhou, Qifeng Chen, Yujun Shen
We present the content deformation field CoDeF as a new type of video representation, which consists of a canonical content field aggregating the static contents in the entire video and a temporal deformation field recording the transformations from the canonical image (i.e., rendered from the canonical content field) to each individual frame along the time axis.Given a target video, these two fields are jointly optimized to reconstruct it through a carefully tailored rendering pipeline.We advisedly introduce some regularizations into the optimization process, urging the canonical content field to inherit semantics (e.g., the object shape) from the video.With such a design, CoDeF naturally supports lifting image algorithms for video processing, in the sense that one can apply an image algorithm to the canonical image and effortlessly propagate the outcomes to the entire video with the aid of the temporal deformation field.We experimentally show that CoDeF is able to lift image-to-image translation to video-to-video translation and lift keypoint detection to keypoint tracking without any training.More importantly, thanks to our lifting strategy that deploys the algorithms on only one image, we achieve superior cross-frame consistency in processed videos compared to existing video-to-video translation approaches, and even manage to track non-rigid objects like water and smog.Project page can be found at https://qiuyu96.github.io/CoDeF/.
PDF Project Webpage: https://qiuyu96.github.io/CoDeF/, Code: https://github.com/qiuyu96/CoDeF
点此查看论文截图
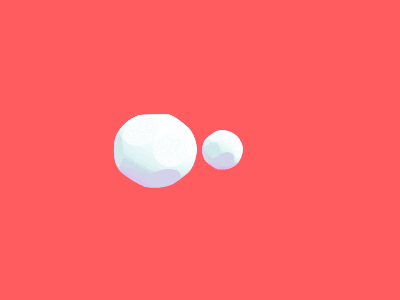
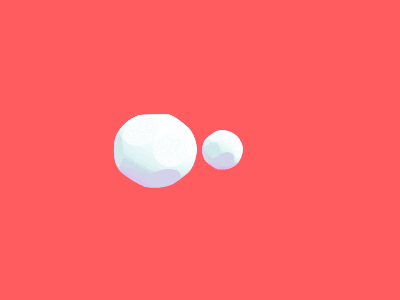
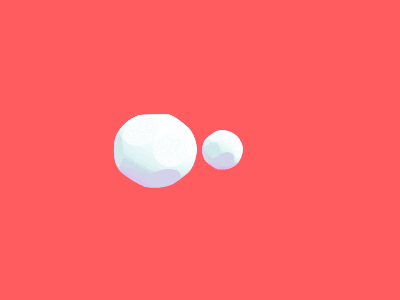
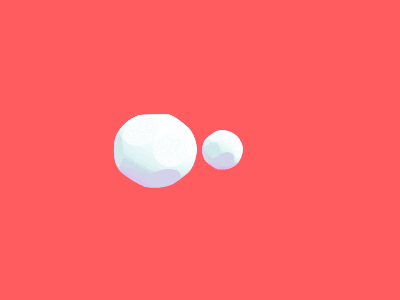
High Performance Computing Applied to Logistic Regression: A CPU and GPU Implementation Comparison
Authors:Nechba Mohammed, Mouhajir Mohamed, Sedjari Yassine
We present a versatile GPU-based parallel version of Logistic Regression (LR), aiming to address the increasing demand for faster algorithms in binary classification due to large data sets. Our implementation is a direct translation of the parallel Gradient Descent Logistic Regression algorithm proposed by X. Zou et al. [12]. Our experiments demonstrate that our GPU-based LR outperforms existing CPU-based implementations in terms of execution time while maintaining comparable f1 score. The significant acceleration of processing large datasets makes our method particularly advantageous for real-time prediction applications like image recognition, spam detection, and fraud detection. Our algorithm is implemented in a ready-to-use Python library available at : https://github.com/NechbaMohammed/SwiftLogisticReg
PDF
点此查看论文截图
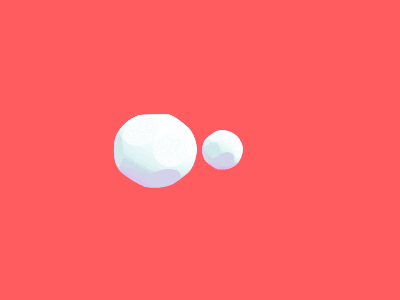
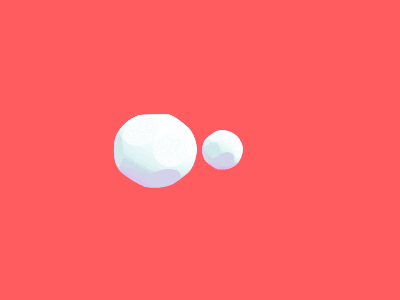
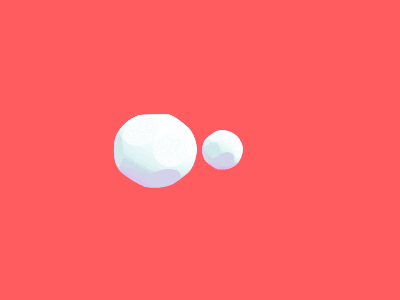
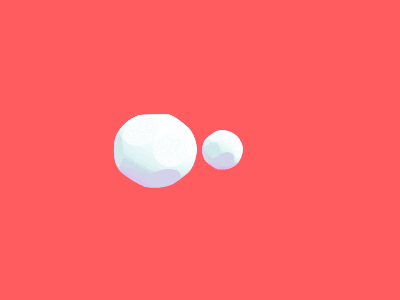
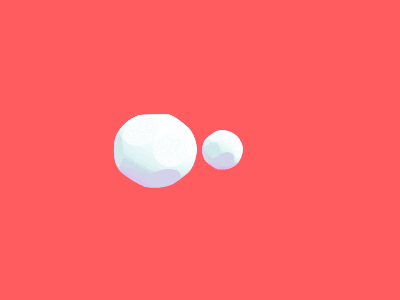
MeDM: Mediating Image Diffusion Models for Video-to-Video Translation with Temporal Correspondence Guidance
Authors:Ernie Chu, Tzuhsuan Huang, Shuo-Yen Lin, Jun-Cheng Chen
This study introduces an efficient and effective method, MeDM, that utilizes pre-trained image Diffusion Models for video-to-video translation with consistent temporal flow. The proposed framework can render videos from scene position information, such as a normal G-buffer, or perform text-guided editing on videos captured in real-world scenarios. We employ explicit optical flows to construct a practical coding that enforces physical constraints on generated frames and mediates independent frame-wise scores. By leveraging this coding, maintaining temporal consistency in the generated videos can be framed as an optimization problem with a closed-form solution. To ensure compatibility with Stable Diffusion, we also suggest a workaround for modifying observed-space scores in latent-space Diffusion Models. Notably, MeDM does not require fine-tuning or test-time optimization of the Diffusion Models. Through extensive qualitative, quantitative, and subjective experiments on various benchmarks, the study demonstrates the effectiveness and superiority of the proposed approach.
PDF
点此查看论文截图
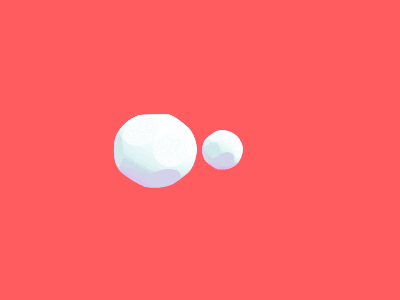
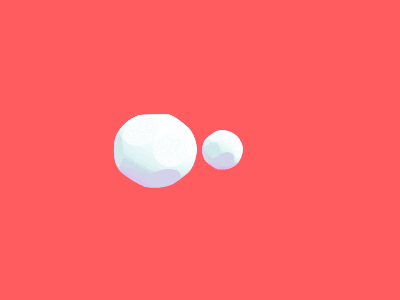
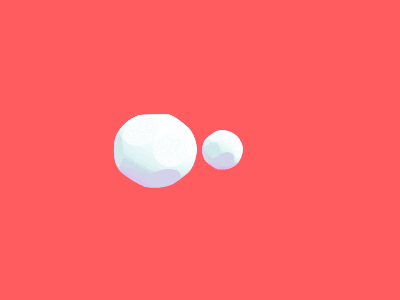
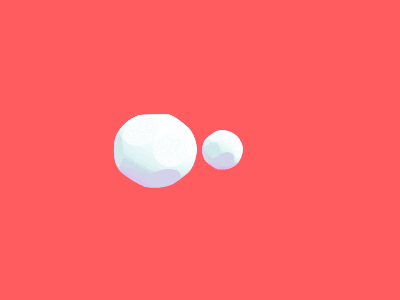
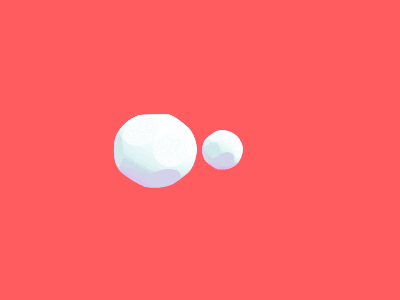
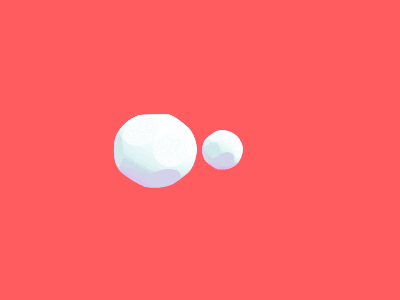
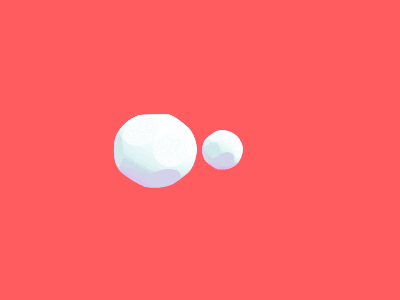
Diffusion-based Image Translation with Label Guidance for Domain Adaptive Semantic Segmentation
Authors:Duo Peng, Ping Hu, Qiuhong Ke, Jun Liu
Translating images from a source domain to a target domain for learning target models is one of the most common strategies in domain adaptive semantic segmentation (DASS). However, existing methods still struggle to preserve semantically-consistent local details between the original and translated images. In this work, we present an innovative approach that addresses this challenge by using source-domain labels as explicit guidance during image translation. Concretely, we formulate cross-domain image translation as a denoising diffusion process and utilize a novel Semantic Gradient Guidance (SGG) method to constrain the translation process, conditioning it on the pixel-wise source labels. Additionally, a Progressive Translation Learning (PTL) strategy is devised to enable the SGG method to work reliably across domains with large gaps. Extensive experiments demonstrate the superiority of our approach over state-of-the-art methods.
PDF Accepted to ICCV2023
点此查看论文截图
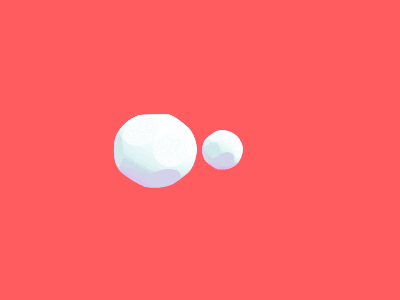
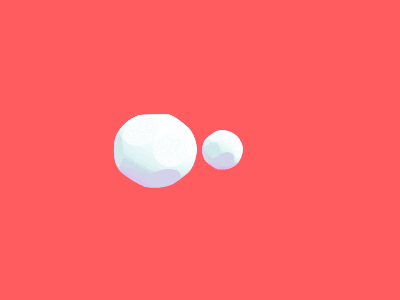
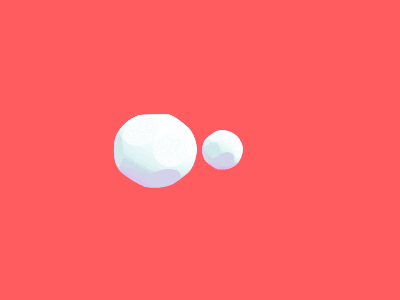
With a Little Help from your own Past: Prototypical Memory Networks for Image Captioning
Authors:Manuele Barraco, Sara Sarto, Marcella Cornia, Lorenzo Baraldi, Rita Cucchiara
Image captioning, like many tasks involving vision and language, currently relies on Transformer-based architectures for extracting the semantics in an image and translating it into linguistically coherent descriptions. Although successful, the attention operator only considers a weighted summation of projections of the current input sample, therefore ignoring the relevant semantic information which can come from the joint observation of other samples. In this paper, we devise a network which can perform attention over activations obtained while processing other training samples, through a prototypical memory model. Our memory models the distribution of past keys and values through the definition of prototype vectors which are both discriminative and compact. Experimentally, we assess the performance of the proposed model on the COCO dataset, in comparison with carefully designed baselines and state-of-the-art approaches, and by investigating the role of each of the proposed components. We demonstrate that our proposal can increase the performance of an encoder-decoder Transformer by 3.7 CIDEr points both when training in cross-entropy only and when fine-tuning with self-critical sequence training. Source code and trained models are available at: https://github.com/aimagelab/PMA-Net.
PDF ICCV 2023
点此查看论文截图
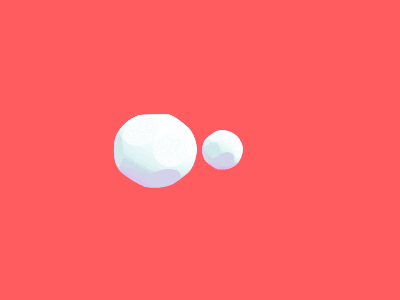
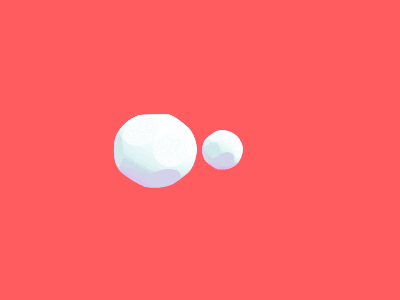
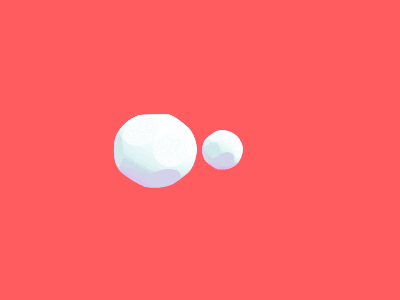
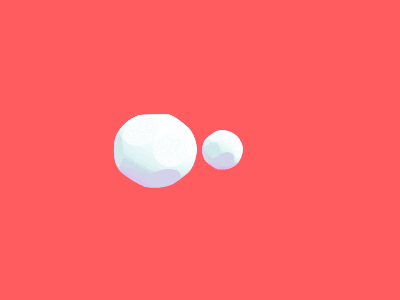
Scenimefy: Learning to Craft Anime Scene via Semi-Supervised Image-to-Image Translation
Authors:Yuxin Jiang, Liming Jiang, Shuai Yang, Chen Change Loy
Automatic high-quality rendering of anime scenes from complex real-world images is of significant practical value. The challenges of this task lie in the complexity of the scenes, the unique features of anime style, and the lack of high-quality datasets to bridge the domain gap. Despite promising attempts, previous efforts are still incompetent in achieving satisfactory results with consistent semantic preservation, evident stylization, and fine details. In this study, we propose Scenimefy, a novel semi-supervised image-to-image translation framework that addresses these challenges. Our approach guides the learning with structure-consistent pseudo paired data, simplifying the pure unsupervised setting. The pseudo data are derived uniquely from a semantic-constrained StyleGAN leveraging rich model priors like CLIP. We further apply segmentation-guided data selection to obtain high-quality pseudo supervision. A patch-wise contrastive style loss is introduced to improve stylization and fine details. Besides, we contribute a high-resolution anime scene dataset to facilitate future research. Our extensive experiments demonstrate the superiority of our method over state-of-the-art baselines in terms of both perceptual quality and quantitative performance.
PDF ICCV 2023. The first two authors contributed equally. Code: https://github.com/Yuxinn-J/Scenimefy Project page: https://yuxinn-j.github.io/projects/Scenimefy.html
点此查看论文截图
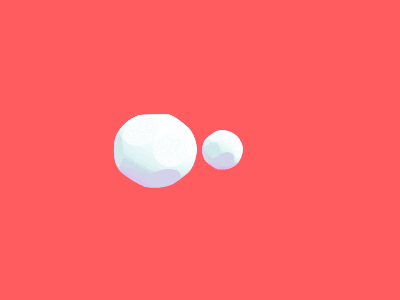
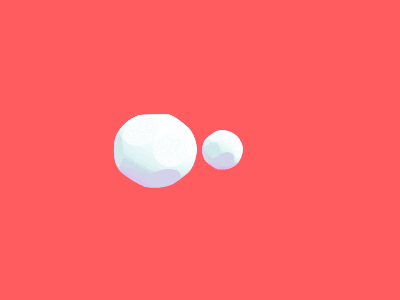
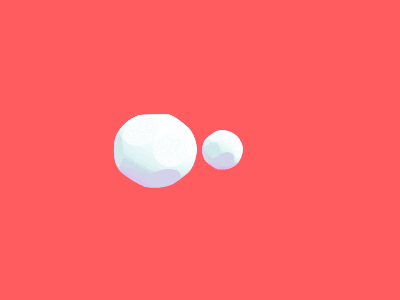
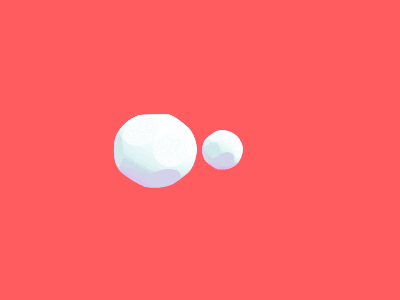
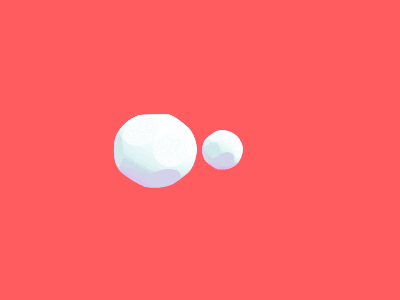
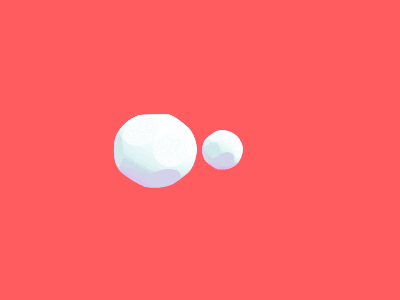