2023-07-01 更新
Inter-Instance Similarity Modeling for Contrastive Learning
Authors:Chengchao Shen, Dawei Liu, Hao Tang, Zhe Qu, Jianxin Wang
The existing contrastive learning methods widely adopt one-hot instance discrimination as pretext task for self-supervised learning, which inevitably neglects rich inter-instance similarities among natural images, then leading to potential representation degeneration. In this paper, we propose a novel image mix method, PatchMix, for contrastive learning in Vision Transformer (ViT), to model inter-instance similarities among images. Following the nature of ViT, we randomly mix multiple images from mini-batch in patch level to construct mixed image patch sequences for ViT. Compared to the existing sample mix methods, our PatchMix can flexibly and efficiently mix more than two images and simulate more complicated similarity relations among natural images. In this manner, our contrastive framework can significantly reduce the gap between contrastive objective and ground truth in reality. Experimental results demonstrate that our proposed method significantly outperforms the previous state-of-the-art on both ImageNet-1K and CIFAR datasets, e.g., 3.0% linear accuracy improvement on ImageNet-1K and 8.7% kNN accuracy improvement on CIFAR100. Moreover, our method achieves the leading transfer performance on downstream tasks, object detection and instance segmentation on COCO dataset. The code is available at https://github.com/visresearch/patchmix
PDF
点此查看论文截图
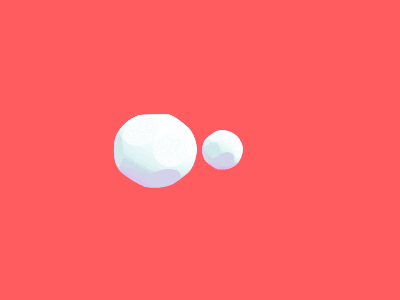
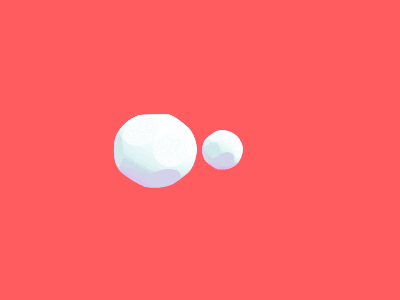
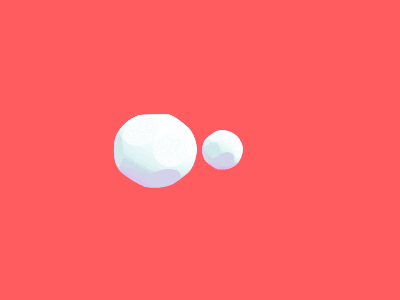
Bridging the Gap: Neural Collapse Inspired Prompt Tuning for Generalization under Class Imbalance
Authors:Didi Zhu, Yinchuan Li, Min Zhang, Junkun Yuan, Jiashuo Liu, Zexi Li, Kun Kuang, Chao Wu
Large-scale vision-language (V-L) models have demonstrated remarkable generalization capabilities for downstream tasks through prompt tuning. However, their performance suffers significantly in the presence of class imbalance, a common issue in real-world scenarios. In this paper, we investigate the effects of class imbalance on the generalization performance of V-L models and extend Neural Collapse phenomenon to these models, revealing the geometric reasons behind the impact of class imbalance on their generalization ability. To address this problem, we propose Neural Collapse based Prompt Tuning (NPT), a novel method that optimizes prompts so that both text and image features satisfy the same simplex ETF structure. NPT incorporates two regularization terms, geometric de-biasing and multi-modal isomorphism, to enhance the robustness of V-L models under class imbalance conditions while maintaining their generalization capabilities. Our comprehensive experiments show that NPT outperforms existing prompt learning techniques across 11 diverse image recognition datasets, achieving an absolute average gain of 2.63\% for novel classes and 2.47\% for harmonic mean when facing imbalanced data.
PDF
点此查看论文截图
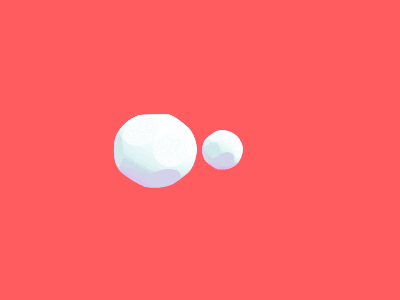
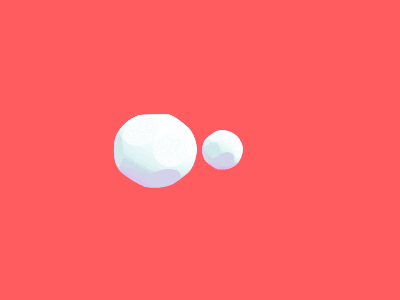
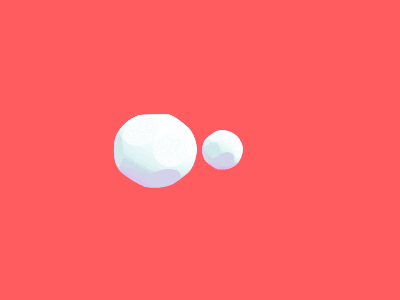
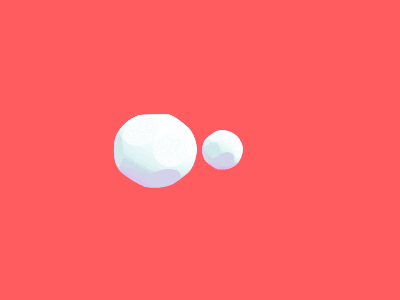
Prompt Ensemble Self-training for Open-Vocabulary Domain Adaptation
Authors:Jiaxing Huang, Jingyi Zhang, Han Qiu, Sheng Jin, Shijian Lu
Traditional domain adaptation assumes the same vocabulary across source and target domains, which often struggles with limited transfer flexibility and efficiency while handling target domains with different vocabularies. Inspired by recent vision-language models (VLMs) that enable open-vocabulary visual recognition by reasoning on both images and texts, we study open-vocabulary domain adaptation (OVDA), a new unsupervised domain adaptation framework that positions a pre-trained VLM as the source model and transfers it towards arbitrary unlabelled target domains. To this end, we design a Prompt Ensemble Self-training (PEST) technique that exploits the synergy between vision and language to mitigate the domain discrepancies in image and text distributions simultaneously. Specifically, PEST makes use of the complementary property of multiple prompts within and across vision and language modalities, which enables joint exploitation of vision and language information and effective learning of image-text correspondences in the unlabelled target domains. Additionally, PEST captures temporal information via temporal prompt ensemble which helps memorize previously learnt target information. Extensive experiments show that PEST outperforms the state-of-the-art consistently across 10 image recognition tasks.
PDF
点此查看论文截图
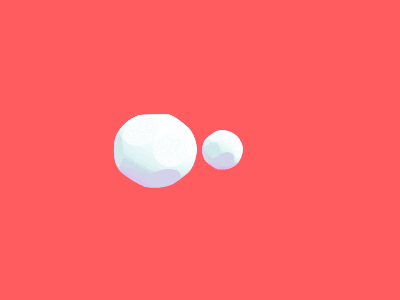
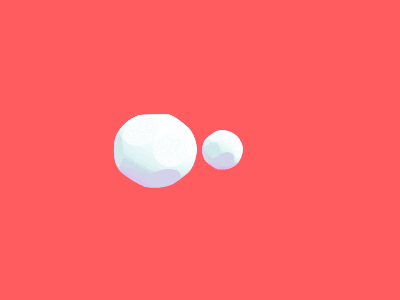
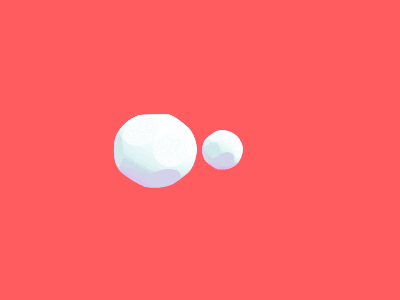
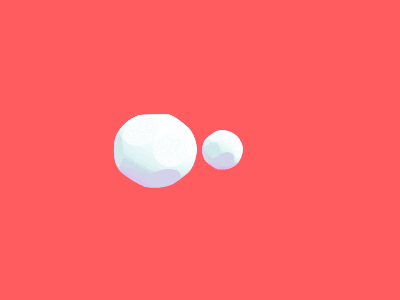
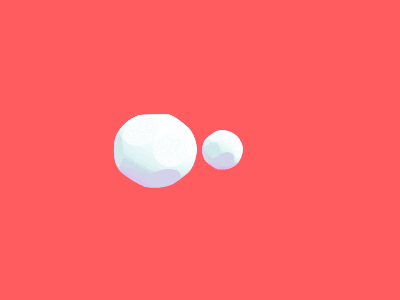