2023-06-19 更新
BN-DRISHTI: Bangla Document Recognition through Instance-level Segmentation of Handwritten Text Images
Authors:Sheikh Mohammad Jubaer, Nazifa Tabassum, Md. Ataur Rahman, Mohammad Khairul Islam
Handwriting recognition remains challenging for some of the most spoken languages, like Bangla, due to the complexity of line and word segmentation brought by the curvilinear nature of writing and lack of quality datasets. This paper solves the segmentation problem by introducing a state-of-the-art method (BN-DRISHTI) that combines a deep learning-based object detection framework (YOLO) with Hough and Affine transformation for skew correction. However, training deep learning models requires a massive amount of data. Thus, we also present an extended version of the BN-HTRd dataset comprising 786 full-page handwritten Bangla document images, line and word-level annotation for segmentation, and corresponding ground truths for word recognition. Evaluation on the test portion of our dataset resulted in an F-score of 99.97% for line and 98% for word segmentation. For comparative analysis, we used three external Bangla handwritten datasets, namely BanglaWriting, WBSUBNdb_text, and ICDAR 2013, where our system outperformed by a significant margin, further justifying the performance of our approach on completely unseen samples.
PDF Will be published under the Springer Springer Lecture Notes in Computer Science (LNCS) series, as part of ICDAR WML 2023
点此查看论文截图
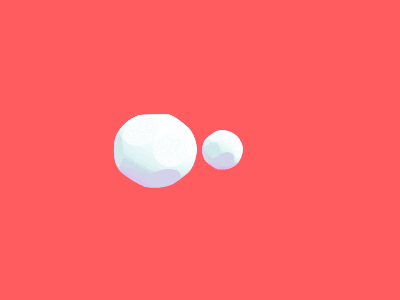
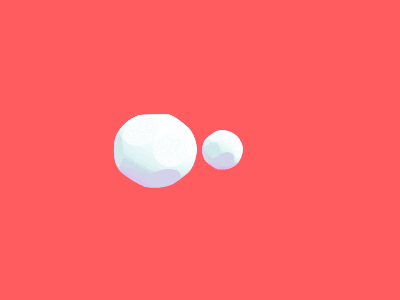
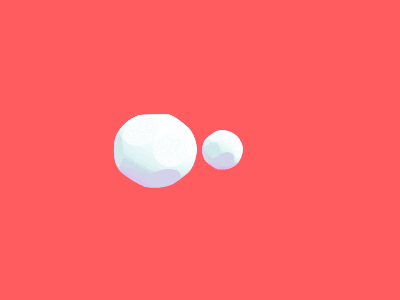
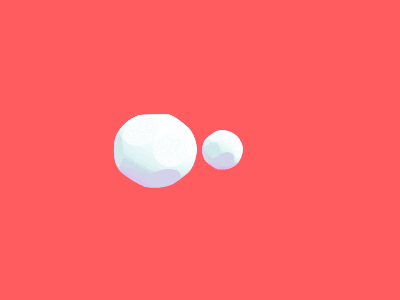
Scaling Open-Vocabulary Object Detection
Authors:Matthias Minderer, Alexey Gritsenko, Neil Houlsby
Open-vocabulary object detection has benefited greatly from pretrained vision-language models, but is still limited by the amount of available detection training data. While detection training data can be expanded by using Web image-text pairs as weak supervision, this has not been done at scales comparable to image-level pretraining. Here, we scale up detection data with self-training, which uses an existing detector to generate pseudo-box annotations on image-text pairs. Major challenges in scaling self-training are the choice of label space, pseudo-annotation filtering, and training efficiency. We present the OWLv2 model and OWL-ST self-training recipe, which address these challenges. OWLv2 surpasses the performance of previous state-of-the-art open-vocabulary detectors already at comparable training scales (~10M examples). However, with OWL-ST, we can scale to over 1B examples, yielding further large improvement: With an L/14 architecture, OWL-ST improves AP on LVIS rare classes, for which the model has seen no human box annotations, from 31.2% to 44.6% (43% relative improvement). OWL-ST unlocks Web-scale training for open-world localization, similar to what has been seen for image classification and language modelling.
PDF
点此查看论文截图
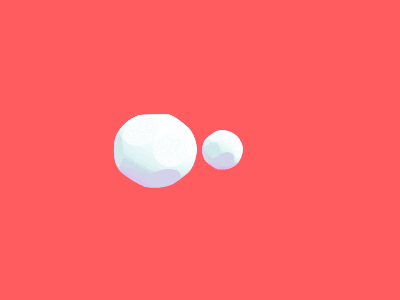
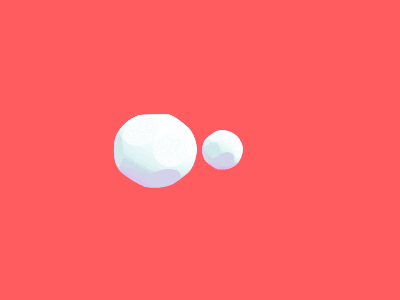
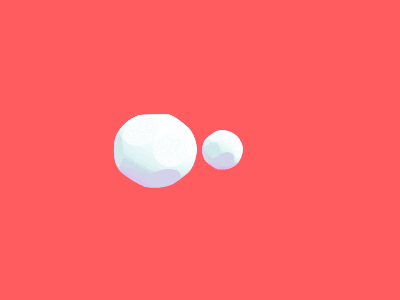
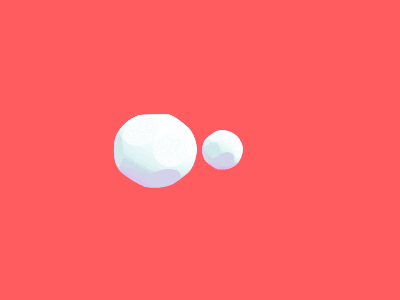