2023-06-19 更新
R2-Diff: Denoising by diffusion as a refinement of retrieved motion for image-based motion prediction
Authors:Takeru Oba, Norimichi Ukita
Image-based motion prediction is one of the essential techniques for robot manipulation. Among the various prediction models, we focus on diffusion models because they have achieved state-of-the-art performance in various applications. In image-based motion prediction, diffusion models stochastically predict contextually appropriate motion by gradually denoising random Gaussian noise based on the image context. While diffusion models are able to predict various motions by changing the random noise, they sometimes fail to predict a contextually appropriate motion based on the image because the random noise is sampled independently of the image context. To solve this problem, we propose R2-Diff. In R2-Diff, a motion retrieved from a dataset based on image similarity is fed into a diffusion model instead of random noise. Then, the retrieved motion is refined through the denoising process of the diffusion model. Since the retrieved motion is almost appropriate to the context, it becomes easier to predict contextually appropriate motion. However, traditional diffusion models are not optimized to refine the retrieved motion. Therefore, we propose the method of tuning the hyperparameters based on the distance of the nearest neighbor motion among the dataset to optimize the diffusion model for refinement. Furthermore, we propose an image-based retrieval method to retrieve the nearest neighbor motion in inference. Our proposed retrieval efficiently computes the similarity based on the image features along the motion trajectory. We demonstrate that R2-Diff accurately predicts appropriate motions and achieves high task success rates compared to recent state-of-the-art models in robot manipulation.
PDF 22 pages, preprint submitted to Neurocomputing
点此查看论文截图
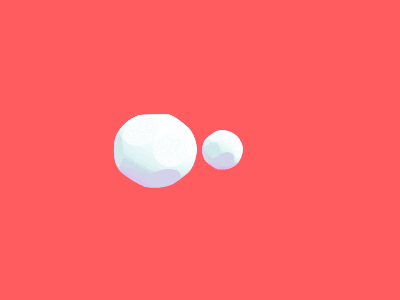
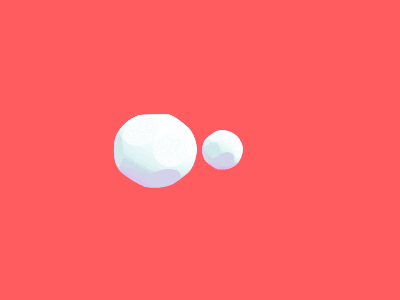
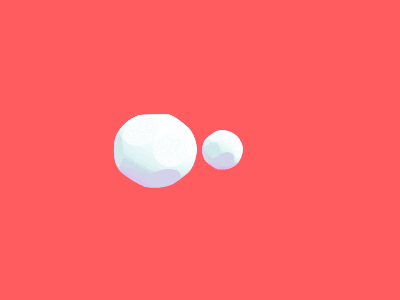
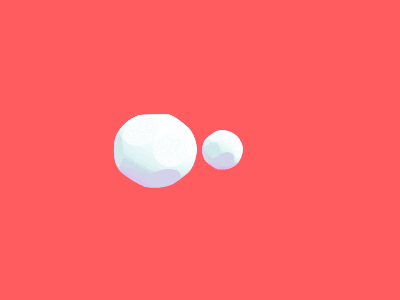
AvatarBooth: High-Quality and Customizable 3D Human Avatar Generation
Authors:Yifei Zeng, Yuanxun Lu, Xinya Ji, Yao Yao, Hao Zhu, Xun Cao
We introduce AvatarBooth, a novel method for generating high-quality 3D avatars using text prompts or specific images. Unlike previous approaches that can only synthesize avatars based on simple text descriptions, our method enables the creation of personalized avatars from casually captured face or body images, while still supporting text-based model generation and editing. Our key contribution is the precise avatar generation control by using dual fine-tuned diffusion models separately for the human face and body. This enables us to capture intricate details of facial appearance, clothing, and accessories, resulting in highly realistic avatar generations. Furthermore, we introduce pose-consistent constraint to the optimization process to enhance the multi-view consistency of synthesized head images from the diffusion model and thus eliminate interference from uncontrolled human poses. In addition, we present a multi-resolution rendering strategy that facilitates coarse-to-fine supervision of 3D avatar generation, thereby enhancing the performance of the proposed system. The resulting avatar model can be further edited using additional text descriptions and driven by motion sequences. Experiments show that AvatarBooth outperforms previous text-to-3D methods in terms of rendering and geometric quality from either text prompts or specific images. Please check our project website at https://zeng-yifei.github.io/avatarbooth_page/.
PDF Project website at https://zeng-yifei.github.io/avatarbooth_page/
点此查看论文截图
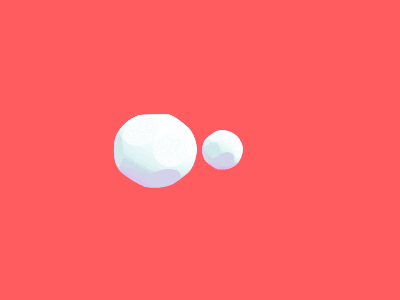
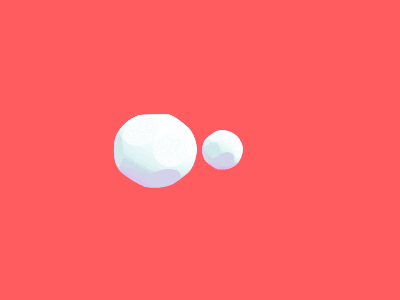
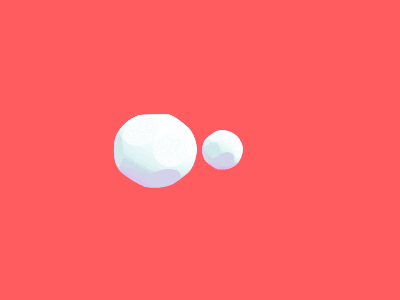
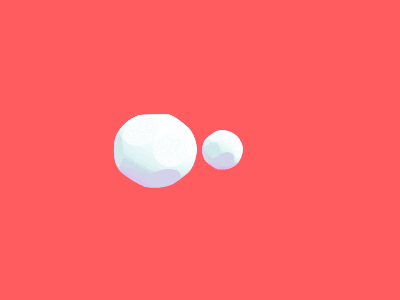
Towards Better Certified Segmentation via Diffusion Models
Authors:Othmane Laousy, Alexandre Araujo, Guillaume Chassagnon, Marie-Pierre Revel, Siddharth Garg, Farshad Khorrami, Maria Vakalopoulou
The robustness of image segmentation has been an important research topic in the past few years as segmentation models have reached production-level accuracy. However, like classification models, segmentation models can be vulnerable to adversarial perturbations, which hinders their use in critical-decision systems like healthcare or autonomous driving. Recently, randomized smoothing has been proposed to certify segmentation predictions by adding Gaussian noise to the input to obtain theoretical guarantees. However, this method exhibits a trade-off between the amount of added noise and the level of certification achieved. In this paper, we address the problem of certifying segmentation prediction using a combination of randomized smoothing and diffusion models. Our experiments show that combining randomized smoothing and diffusion models significantly improves certified robustness, with results indicating a mean improvement of 21 points in accuracy compared to previous state-of-the-art methods on Pascal-Context and Cityscapes public datasets. Our method is independent of the selected segmentation model and does not need any additional specialized training procedure.
PDF
点此查看论文截图
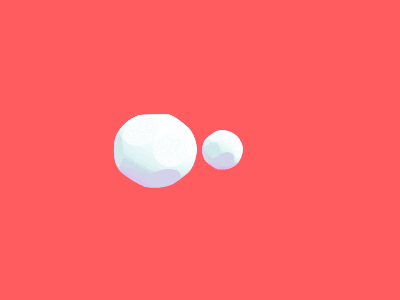
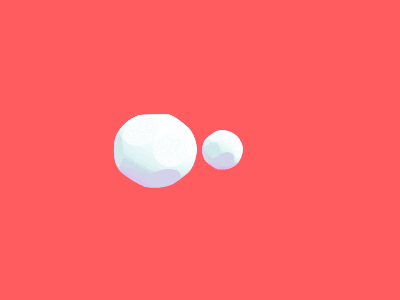
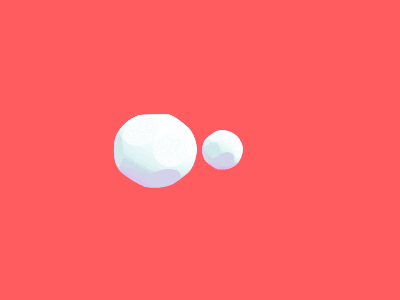
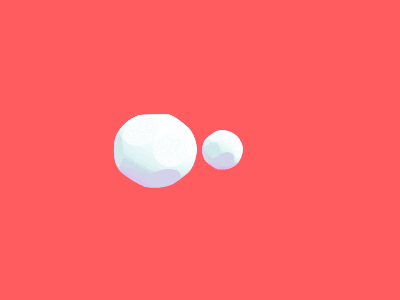
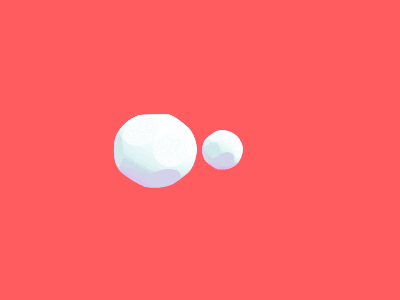