2023-06-16 更新
Object Detection in Hyperspectral Image via Unified Spectral-Spatial Feature Aggregation
Authors:Xiao He, Chang Tang, Xinwang Liu, Wei Zhang, Kun Sun, Jiangfeng Xu
Deep learning-based hyperspectral image (HSI) classification and object detection techniques have gained significant attention due to their vital role in image content analysis, interpretation, and wider HSI applications. However, current hyperspectral object detection approaches predominantly emphasize either spectral or spatial information, overlooking the valuable complementary relationship between these two aspects. In this study, we present a novel \textbf{S}pectral-\textbf{S}patial \textbf{A}ggregation (S2ADet) object detector that effectively harnesses the rich spectral and spatial complementary information inherent in hyperspectral images. S2ADet comprises a hyperspectral information decoupling (HID) module, a two-stream feature extraction network, and a one-stage detection head. The HID module processes hyperspectral images by aggregating spectral and spatial information via band selection and principal components analysis, consequently reducing redundancy. Based on the acquired spatial and spectral aggregation information, we propose a feature aggregation two-stream network for interacting spectral-spatial features. Furthermore, to address the limitations of existing databases, we annotate an extensive dataset, designated as HOD3K, containing 3,242 hyperspectral images captured across diverse real-world scenes and encompassing three object classes. These images possess a resolution of 512x256 pixels and cover 16 bands ranging from 470 nm to 620 nm. Comprehensive experiments on two datasets demonstrate that S2ADet surpasses existing state-of-the-art methods, achieving robust and reliable results. The demo code and dataset of this work are publicly available at \url{https://github.com/hexiao-cs/S2ADet}.
PDF
点此查看论文截图
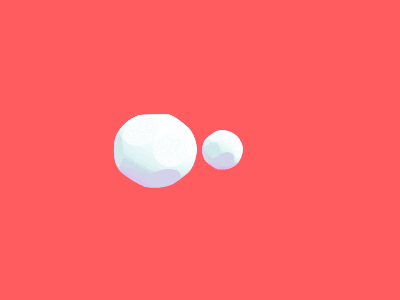
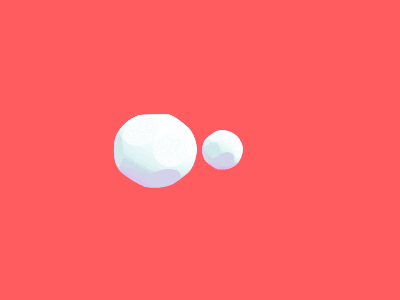
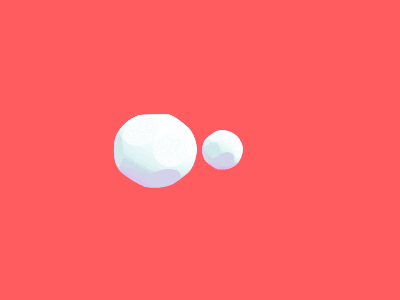
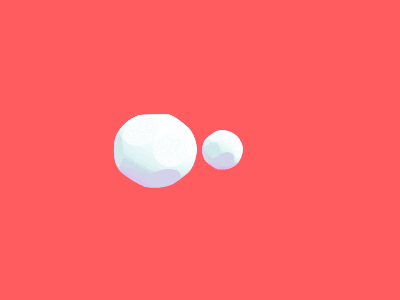
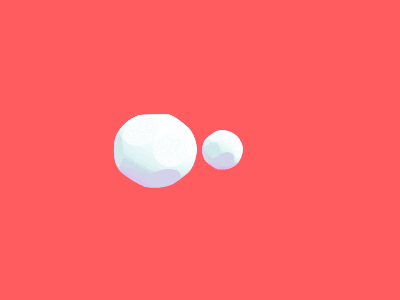
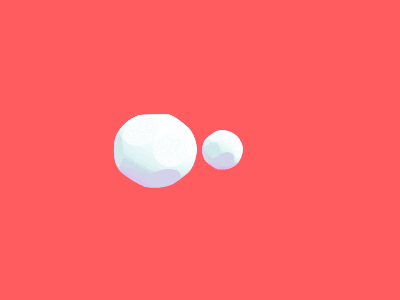