2023-06-15 更新
RePaint-NeRF: NeRF Editting via Semantic Masks and Diffusion Models
Authors:Xingchen Zhou, Ying He, F. Richard Yu, Jianqiang Li, You Li
The emergence of Neural Radiance Fields (NeRF) has promoted the development of synthesized high-fidelity views of the intricate real world. However, it is still a very demanding task to repaint the content in NeRF. In this paper, we propose a novel framework that can take RGB images as input and alter the 3D content in neural scenes. Our work leverages existing diffusion models to guide changes in the designated 3D content. Specifically, we semantically select the target object and a pre-trained diffusion model will guide the NeRF model to generate new 3D objects, which can improve the editability, diversity, and application range of NeRF. Experiment results show that our algorithm is effective for editing 3D objects in NeRF under different text prompts, including editing appearance, shape, and more. We validate our method on both real-world datasets and synthetic-world datasets for these editing tasks. Please visit https://repaintnerf.github.io for a better view of our results.
PDF IJCAI 2023 Accepted (Main Track)
点此查看论文截图
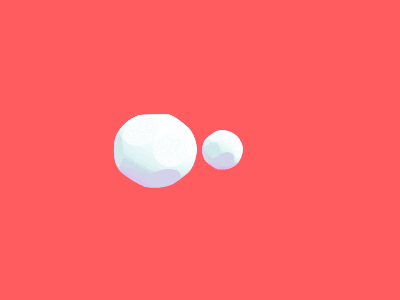
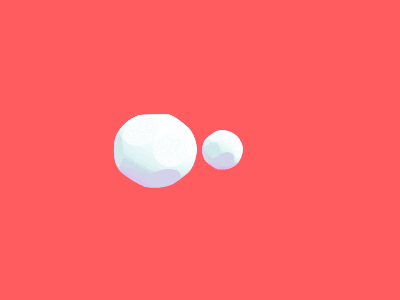
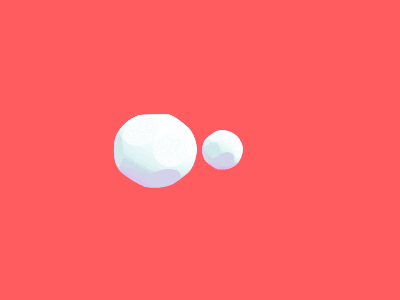
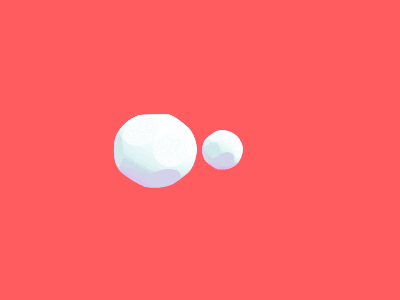
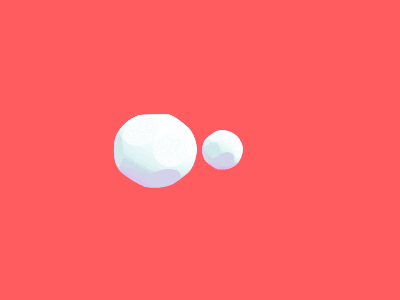
HyP-NeRF: Learning Improved NeRF Priors using a HyperNetwork
Authors:Bipasha Sen, Gaurav Singh, Aditya Agarwal, Rohith Agaram, K Madhava Krishna, Srinath Sridhar
Neural Radiance Fields (NeRF) have become an increasingly popular representation to capture high-quality appearance and shape of scenes and objects. However, learning generalizable NeRF priors over categories of scenes or objects has been challenging due to the high dimensionality of network weight space. To address the limitations of existing work on generalization, multi-view consistency and to improve quality, we propose HyP-NeRF, a latent conditioning method for learning generalizable category-level NeRF priors using hypernetworks. Rather than using hypernetworks to estimate only the weights of a NeRF, we estimate both the weights and the multi-resolution hash encodings resulting in significant quality gains. To improve quality even further, we incorporate a denoise and finetune strategy that denoises images rendered from NeRFs estimated by the hypernetwork and finetunes it while retaining multiview consistency. These improvements enable us to use HyP-NeRF as a generalizable prior for multiple downstream tasks including NeRF reconstruction from single-view or cluttered scenes and text-to-NeRF. We provide qualitative comparisons and evaluate HyP-NeRF on three tasks: generalization, compression, and retrieval, demonstrating our state-of-the-art results.
PDF
点此查看论文截图
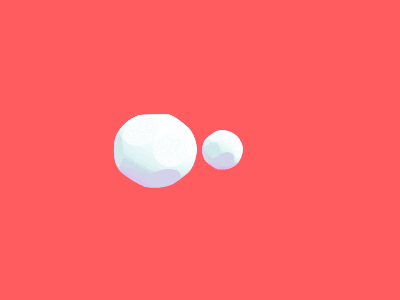
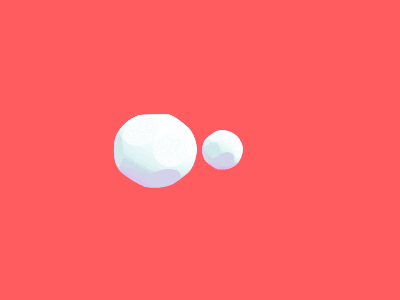
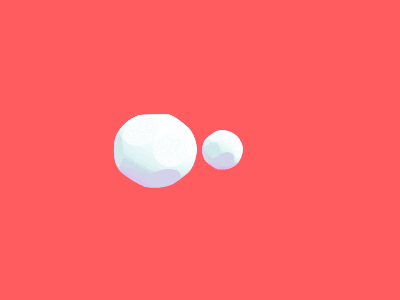
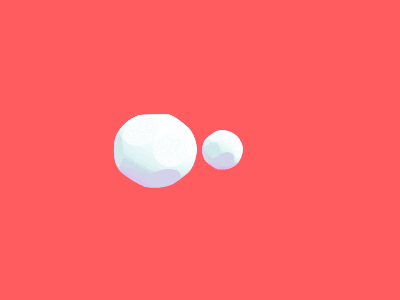
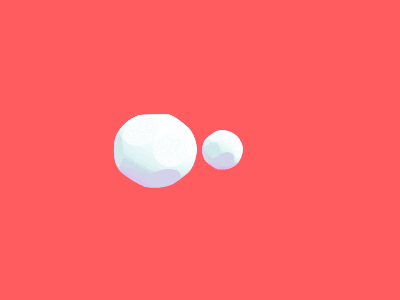
NeRFool: Uncovering the Vulnerability of Generalizable Neural Radiance Fields against Adversarial Perturbations
Authors:Yonggan Fu, Ye Yuan, Souvik Kundu, Shang Wu, Shunyao Zhang, Yingyan Lin
Generalizable Neural Radiance Fields (GNeRF) are one of the most promising real-world solutions for novel view synthesis, thanks to their cross-scene generalization capability and thus the possibility of instant rendering on new scenes. While adversarial robustness is essential for real-world applications, little study has been devoted to understanding its implication on GNeRF. We hypothesize that because GNeRF is implemented by conditioning on the source views from new scenes, which are often acquired from the Internet or third-party providers, there are potential new security concerns regarding its real-world applications. Meanwhile, existing understanding and solutions for neural networks’ adversarial robustness may not be applicable to GNeRF, due to its 3D nature and uniquely diverse operations. To this end, we present NeRFool, which to the best of our knowledge is the first work that sets out to understand the adversarial robustness of GNeRF. Specifically, NeRFool unveils the vulnerability patterns and important insights regarding GNeRF’s adversarial robustness. Built upon the above insights gained from NeRFool, we further develop NeRFool+, which integrates two techniques capable of effectively attacking GNeRF across a wide range of target views, and provide guidelines for defending against our proposed attacks. We believe that our NeRFool/NeRFool+ lays the initial foundation for future innovations in developing robust real-world GNeRF solutions. Our codes are available at: https://github.com/GATECH-EIC/NeRFool.
PDF Accepted by ICML 2023
点此查看论文截图
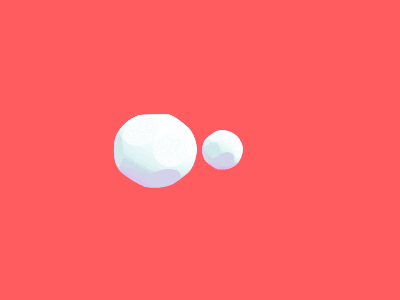
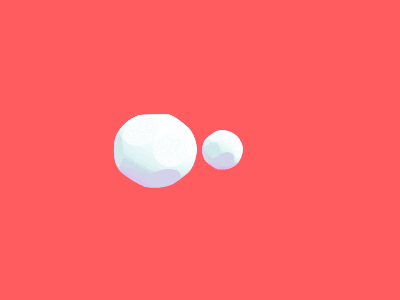
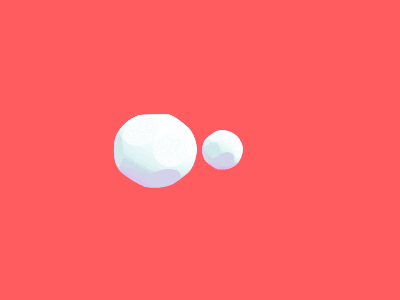
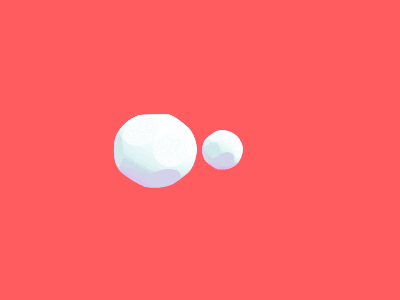
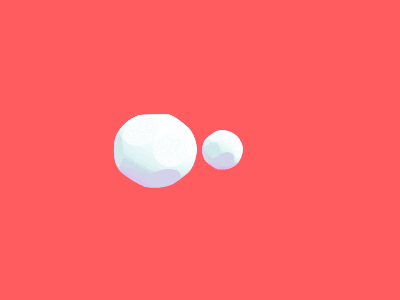
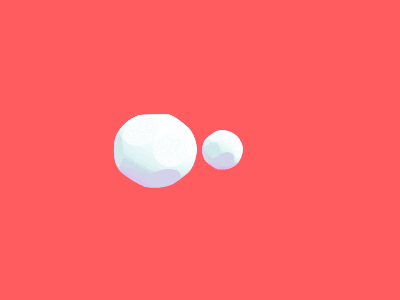
Parametric Implicit Face Representation for Audio-Driven Facial Reenactment
Authors:Ricong Huang, Peiwen Lai, Yipeng Qin, Guanbin Li
Audio-driven facial reenactment is a crucial technique that has a range of applications in film-making, virtual avatars and video conferences. Existing works either employ explicit intermediate face representations (e.g., 2D facial landmarks or 3D face models) or implicit ones (e.g., Neural Radiance Fields), thus suffering from the trade-offs between interpretability and expressive power, hence between controllability and quality of the results. In this work, we break these trade-offs with our novel parametric implicit face representation and propose a novel audio-driven facial reenactment framework that is both controllable and can generate high-quality talking heads. Specifically, our parametric implicit representation parameterizes the implicit representation with interpretable parameters of 3D face models, thereby taking the best of both explicit and implicit methods. In addition, we propose several new techniques to improve the three components of our framework, including i) incorporating contextual information into the audio-to-expression parameters encoding; ii) using conditional image synthesis to parameterize the implicit representation and implementing it with an innovative tri-plane structure for efficient learning; iii) formulating facial reenactment as a conditional image inpainting problem and proposing a novel data augmentation technique to improve model generalizability. Extensive experiments demonstrate that our method can generate more realistic results than previous methods with greater fidelity to the identities and talking styles of speakers.
PDF CVPR 2023
点此查看论文截图
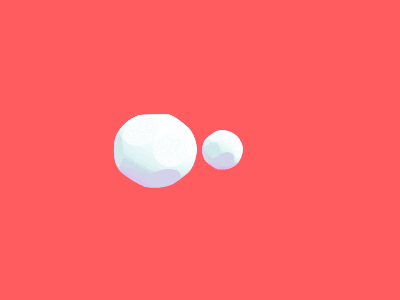
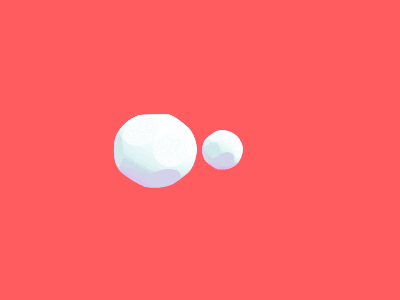
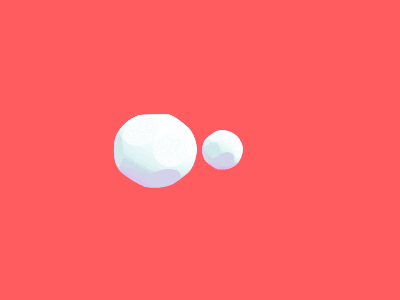
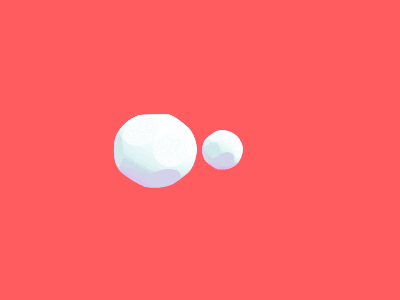
Binary Radiance Fields
Authors:Seungjoo Shin, Jaesik Park
In this paper, we propose binary radiance fields (BiRF), a storage-efficient radiance field representation employing binary feature encoding that encodes local features using binary encoding parameters in a format of either $+1$ or $-1$. This binarization strategy lets us represent the feature grid with highly compact feature encoding and a dramatic reduction in storage size. Furthermore, our 2D-3D hybrid feature grid design enhances the compactness of feature encoding as the 3D grid includes main components while 2D grids capture details. In our experiments, binary radiance field representation successfully outperforms the reconstruction performance of state-of-the-art (SOTA) efficient radiance field models with lower storage allocation. In particular, our model achieves impressive results in static scene reconstruction, with a PSNR of 31.53 dB for Synthetic-NeRF scenes, 34.26 dB for Synthetic-NSVF scenes, 28.02 dB for Tanks and Temples scenes while only utilizing 0.7 MB, 0.8 MB, and 0.8 MB of storage space, respectively. We hope the proposed binary radiance field representation will make radiance fields more accessible without a storage bottleneck.
PDF 21 pages, 12 Figures, and 11 Tables
点此查看论文截图
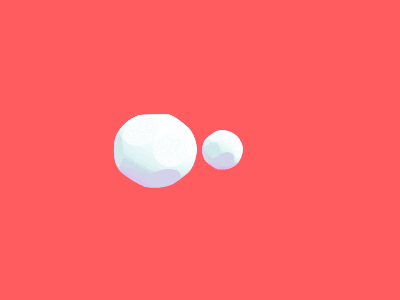
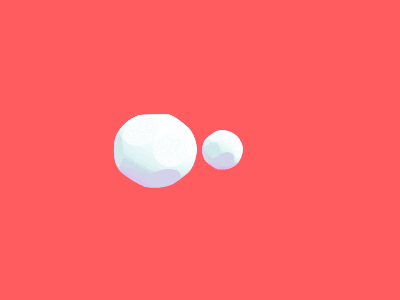
NeuS-PIR: Learning Relightable Neural Surface using Pre-Integrated Rendering
Authors:Shi Mao, Chenming Wu, Zhelun Shen, Liangjun Zhang
Recent advances in neural implicit fields enables rapidly reconstructing 3D geometry from multi-view images. Beyond that, recovering physical properties such as material and illumination is essential for enabling more applications. This paper presents a new method that effectively learns relightable neural surface using pre-intergrated rendering, which simultaneously learns geometry, material and illumination within the neural implicit field. The key insight of our work is that these properties are closely related to each other, and optimizing them in a collaborative manner would lead to consistent improvements. Specifically, we propose NeuS-PIR, a method that factorizes the radiance field into a spatially varying material field and a differentiable environment cubemap, and jointly learns it with geometry represented by neural surface. Our experiments demonstrate that the proposed method outperforms the state-of-the-art method in both synthetic and real datasets.
PDF
点此查看论文截图
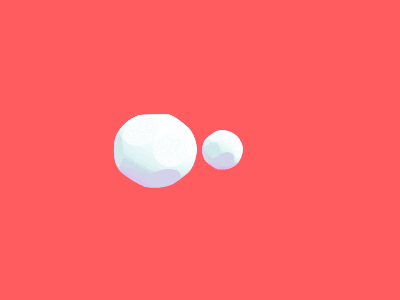
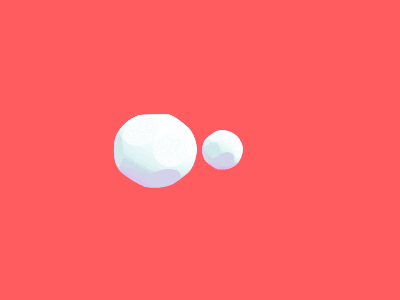
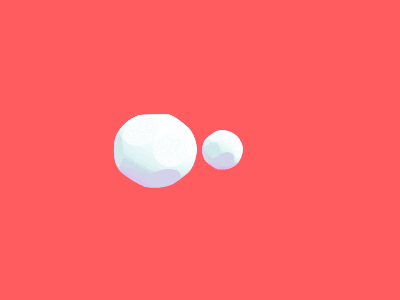
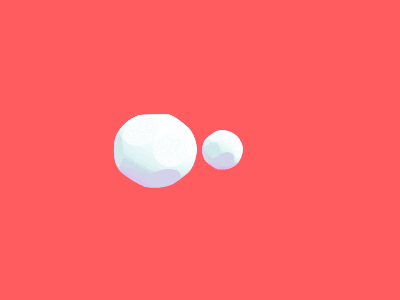
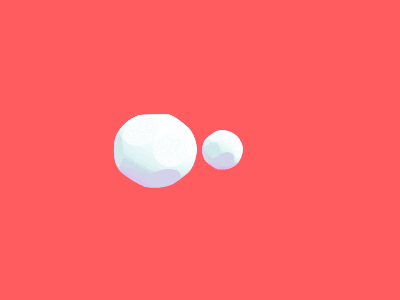