2023-06-15 更新
Revisiting Token Pruning for Object Detection and Instance Segmentation
Authors:Yifei Liu, Mathias Gehrig, Nico Messikommer, Marco Cannici, Davide Scaramuzza
Vision Transformers (ViTs) have shown impressive performance in computer vision, but their high computational cost, quadratic in the number of tokens, limits their adoption in computation-constrained applications. However, this large number of tokens may not be necessary, as not all tokens are equally important. In this paper, we investigate token pruning to accelerate inference for object detection and instance segmentation, extending prior works from image classification. Through extensive experiments, we offer four insights for dense tasks: (i) tokens should not be completely pruned and discarded, but rather preserved in the feature maps for later use. (ii) reactivating previously pruned tokens can further enhance model performance. (iii) a dynamic pruning rate based on images is better than a fixed pruning rate. (iv) a lightweight, 2-layer MLP can effectively prune tokens, achieving accuracy comparable with complex gating networks with a simpler design. We evaluate the impact of these design choices on COCO dataset and present a method integrating these insights that outperforms prior art token pruning models, significantly reducing performance drop from ~1.5 mAP to ~0.3 mAP for both boxes and masks. Compared to the dense counterpart that uses all tokens, our method achieves up to 34% faster inference speed for the whole network and 46% for the backbone.
PDF
点此查看论文截图
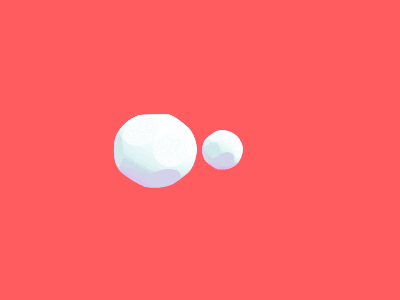
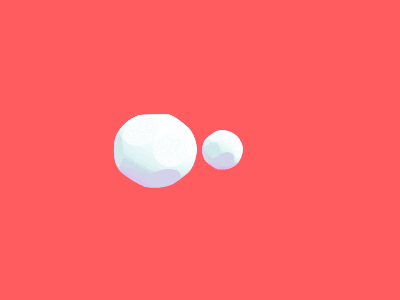
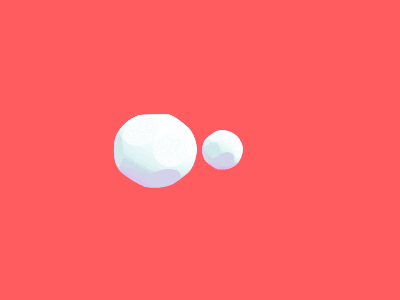
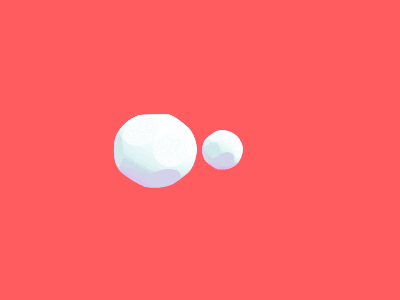
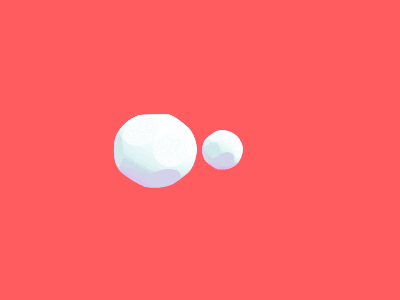
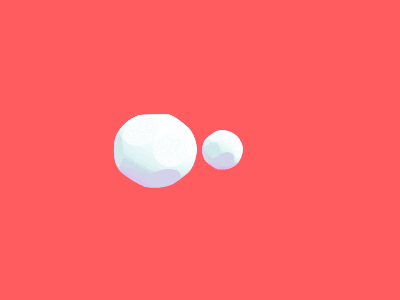
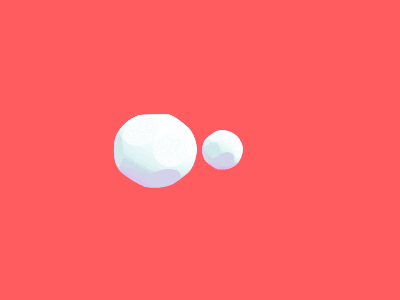
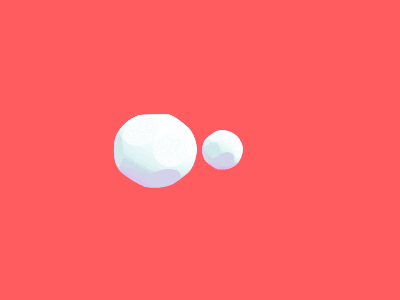
Referring Camouflaged Object Detection
Authors:Xuying Zhang, Bowen Yin, Zheng Lin, Qibin Hou, Deng-Ping Fan, Ming-Ming Cheng
In this paper, we consider the problem of referring camouflaged object detection (Ref-COD), a new task that aims to segment specified camouflaged objects based on some form of reference, e.g., image, text. We first assemble a large-scale dataset, called R2C7K, which consists of 7K images covering 64 object categories in real-world scenarios. Then, we develop a simple but strong dual-branch framework, dubbed R2CNet, with a reference branch learning common representations from the referring information and a segmentation branch identifying and segmenting camouflaged objects under the guidance of the common representations. In particular, we design a Referring Mask Generation module to generate pixel-level prior mask and a Referring Feature Enrichment module to enhance the capability of identifying camouflaged objects. Extensive experiments show the superiority of our Ref-COD methods over their COD counterparts in segmenting specified camouflaged objects and identifying the main body of target objects. Our code and dataset are publicly available at https://github.com/zhangxuying1004/RefCOD.
PDF
点此查看论文截图
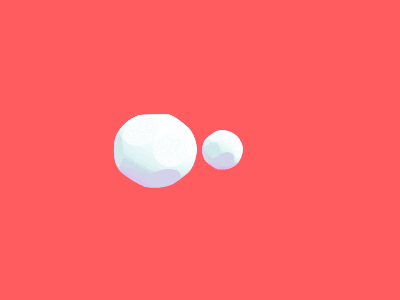
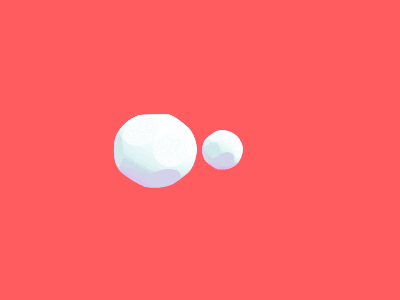
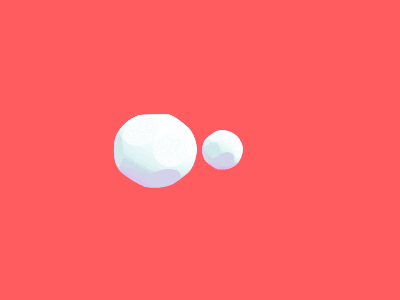
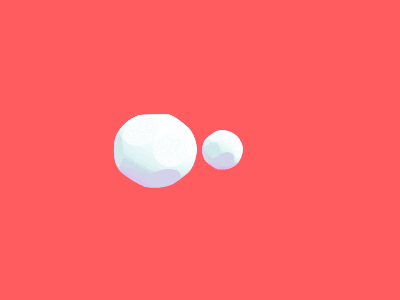
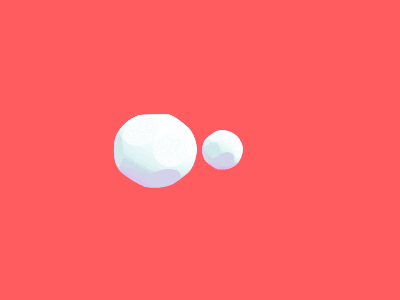
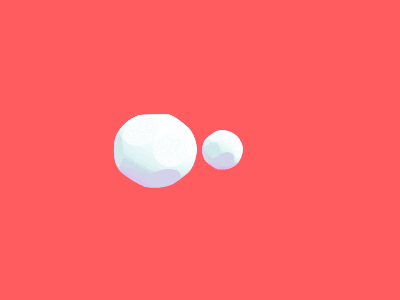