2023-05-30 更新
A Model-Based Solution to the Offline Multi-Agent Reinforcement Learning Coordination Problem
Authors:Paul Barde, Jakob Foerster, Derek Nowrouzezahrai, Amy Zhang
Training multiple agents to coordinate is an important problem with applications in robotics, game theory, economics, and social sciences. However, most existing Multi-Agent Reinforcement Learning (MARL) methods are online and thus impractical for real-world applications in which collecting new interactions is costly or dangerous. While these algorithms should leverage offline data when available, doing so gives rise to the offline coordination problem. Specifically, we identify and formalize the strategy agreement (SA) and the strategy fine-tuning (SFT) challenges, two coordination issues at which current offline MARL algorithms fail. To address this setback, we propose a simple model-based approach that generates synthetic interaction data and enables agents to converge on a strategy while fine-tuning their policies accordingly. Our resulting method, Model-based Offline Multi-Agent Proximal Policy Optimization (MOMA-PPO), outperforms the prevalent learning methods in challenging offline multi-agent MuJoCo tasks even under severe partial observability and with learned world models.
PDF
点此查看论文截图
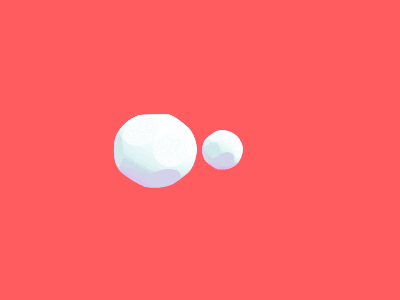
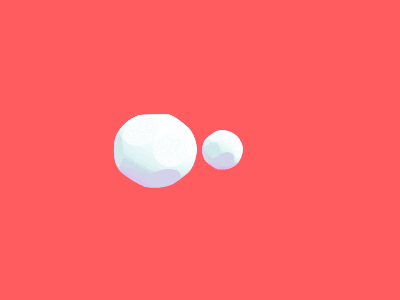