2023-04-24 更新
Text2Seg: Remote Sensing Image Semantic Segmentation via Text-Guided Visual Foundation Models
Authors:Jielu Zhang, Zhongliang Zhou, Gengchen Mai, Lan Mu, Mengxuan Hu, Sheng Li
Recent advancements in foundation models (FMs), such as GPT-4 and LLaMA, have attracted significant attention due to their exceptional performance in zero-shot learning scenarios. Similarly, in the field of visual learning, models like Grounding DINO and the Segment Anything Model (SAM) have exhibited remarkable progress in open-set detection and instance segmentation tasks. It is undeniable that these FMs will profoundly impact a wide range of real-world visual learning tasks, ushering in a new paradigm shift for developing such models. In this study, we concentrate on the remote sensing domain, where the images are notably dissimilar from those in conventional scenarios. We developed a pipeline that leverages multiple FMs to facilitate remote sensing image semantic segmentation tasks guided by text prompt, which we denote as Text2Seg. The pipeline is benchmarked on several widely-used remote sensing datasets, and we present preliminary results to demonstrate its effectiveness. Through this work, we aim to provide insights into maximizing the applicability of visual FMs in specific contexts with minimal model tuning. The code is available at https://github.com/Douglas2Code/Text2Seg.
PDF 10 pages, 6 figures
点此查看论文截图
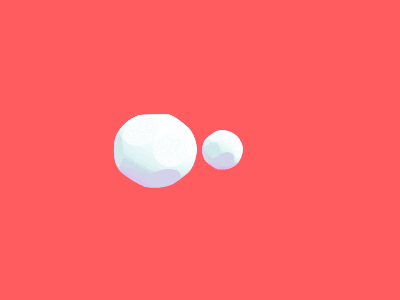
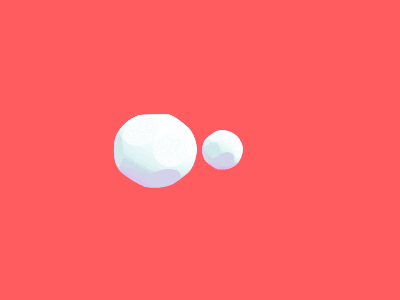
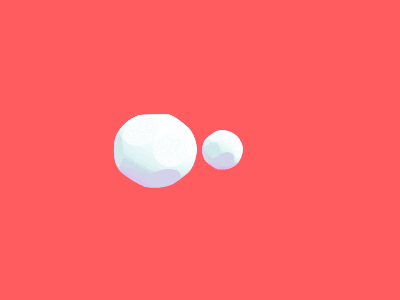
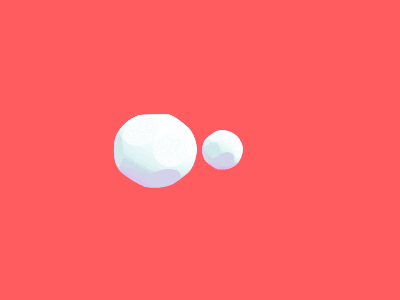
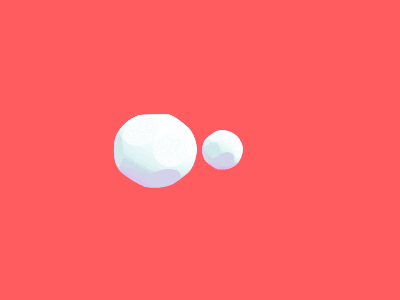
HabitatDyn Dataset: Dynamic Object Detection to Kinematics Estimation
Authors:Zhengcheng Shen, Yi Gao, Linh Kästner, Jens Lambrecht
The advancement of computer vision and machine learning has made datasets a crucial element for further research and applications. However, the creation and development of robots with advanced recognition capabilities are hindered by the lack of appropriate datasets. Existing image or video processing datasets are unable to accurately depict observations from a moving robot, and they do not contain the kinematics information necessary for robotic tasks. Synthetic data, on the other hand, are cost-effective to create and offer greater flexibility for adapting to various applications. Hence, they are widely utilized in both research and industry. In this paper, we propose the dataset HabitatDyn, which contains both synthetic RGB videos, semantic labels, and depth information, as well as kinetics information. HabitatDyn was created from the perspective of a mobile robot with a moving camera, and contains 30 scenes featuring six different types of moving objects with varying velocities. To demonstrate the usability of our dataset, two existing algorithms are used for evaluation and an approach to estimate the distance between the object and camera is implemented based on these segmentation methods and evaluated through the dataset. With the availability of this dataset, we aspire to foster further advancements in the field of mobile robotics, leading to more capable and intelligent robots that can navigate and interact with their environments more effectively. The code is publicly available at https://github.com/ignc-research/HabitatDyn.
PDF The paper is under review
点此查看论文截图
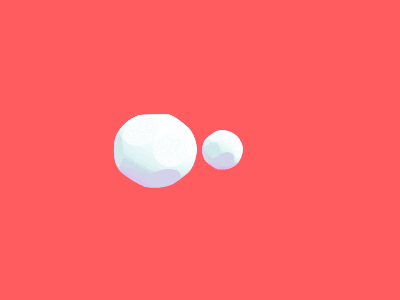
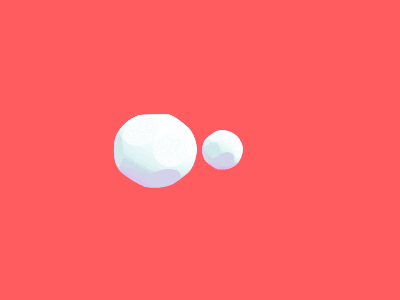
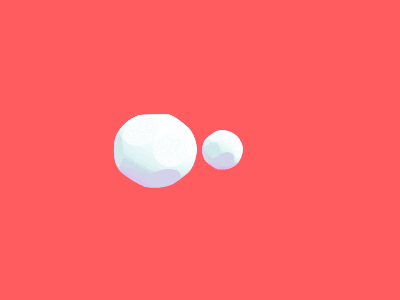
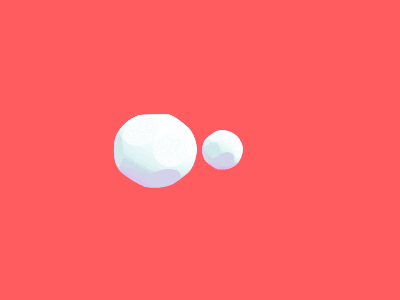
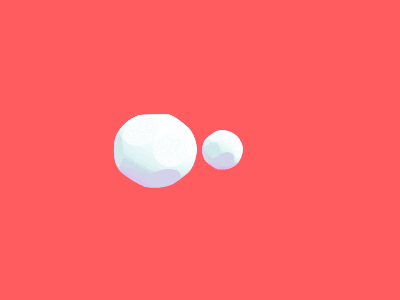
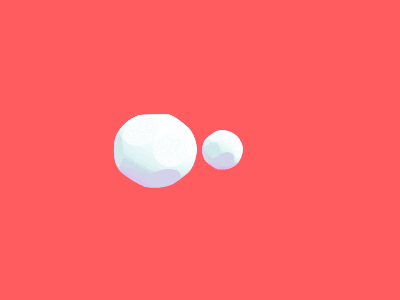
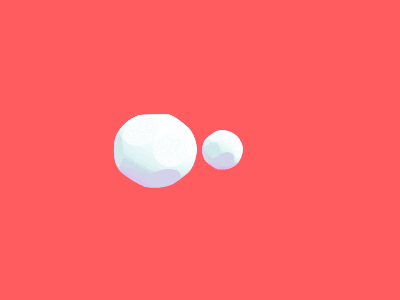
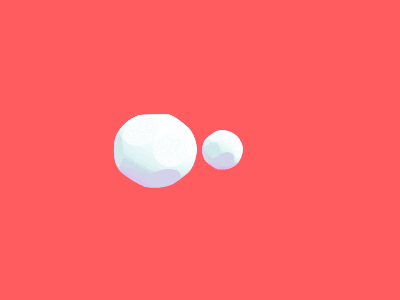