2023-04-24 更新
Can SAM Count Anything? An Empirical Study on SAM Counting
Authors:Zhiheng Ma, Xiaopeng Hong, Qinnan Shangguan
Meta AI recently released the Segment Anything model (SAM), which has garnered attention due to its impressive performance in class-agnostic segmenting. In this study, we explore the use of SAM for the challenging task of few-shot object counting, which involves counting objects of an unseen category by providing a few bounding boxes of examples. We compare SAM’s performance with other few-shot counting methods and find that it is currently unsatisfactory without further fine-tuning, particularly for small and crowded objects. Code can be found at \url{https://github.com/Vision-Intelligence-and-Robots-Group/count-anything}.
PDF An empirical study on few-shot counting using Meta AI’s segment anything model
点此查看论文截图
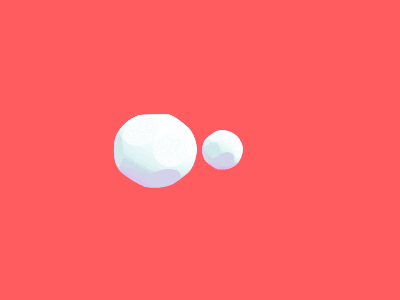
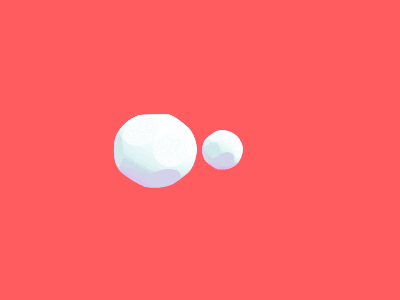
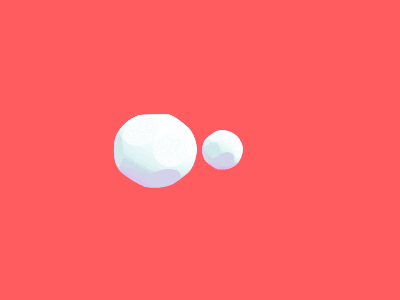
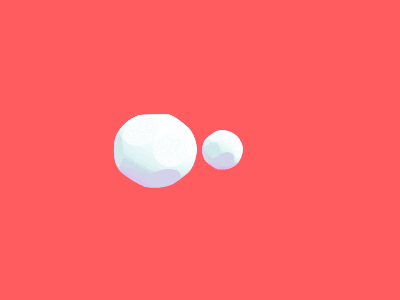
Information Extraction from Documents: Question Answering vs Token Classification in real-world setups
Authors:Laurent Lam, Pirashanth Ratnamogan, Joël Tang, William Vanhuffel, Fabien Caspani
Research in Document Intelligence and especially in Document Key Information Extraction (DocKIE) has been mainly solved as Token Classification problem. Recent breakthroughs in both natural language processing (NLP) and computer vision helped building document-focused pre-training methods, leveraging a multimodal understanding of the document text, layout and image modalities. However, these breakthroughs also led to the emergence of a new DocKIE subtask of extractive document Question Answering (DocQA), as part of the Machine Reading Comprehension (MRC) research field. In this work, we compare the Question Answering approach with the classical token classification approach for document key information extraction. We designed experiments to benchmark five different experimental setups : raw performances, robustness to noisy environment, capacity to extract long entities, fine-tuning speed on Few-Shot Learning and finally Zero-Shot Learning. Our research showed that when dealing with clean and relatively short entities, it is still best to use token classification-based approach, while the QA approach could be a good alternative for noisy environment or long entities use-cases.
PDF
点此查看论文截图
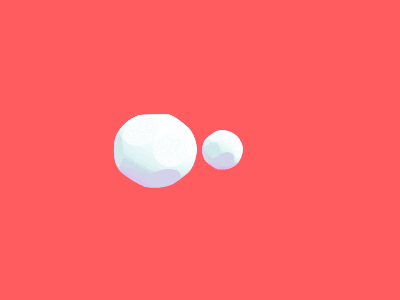
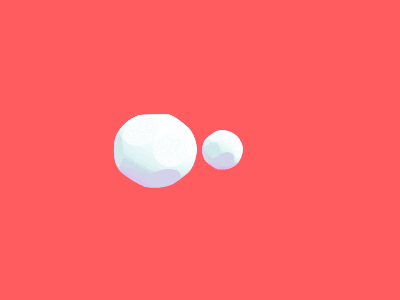