2023-04-24 更新
DiFaReli: Diffusion Face Relighting
Authors:Puntawat Ponglertnapakorn, Nontawat Tritrong, Supasorn Suwajanakorn
We present a novel approach to single-view face relighting in the wild. Handling non-diffuse effects, such as global illumination or cast shadows, has long been a challenge in face relighting. Prior work often assumes Lambertian surfaces, simplified lighting models or involves estimating 3D shape, albedo, or a shadow map. This estimation, however, is error-prone and requires many training examples with lighting ground truth to generalize well. Our work bypasses the need for accurate estimation of intrinsic components and can be trained solely on 2D images without any light stage data, multi-view images, or lighting ground truth. Our key idea is to leverage a conditional diffusion implicit model (DDIM) for decoding a disentangled light encoding along with other encodings related to 3D shape and facial identity inferred from off-the-shelf estimators. We also propose a novel conditioning technique that eases the modeling of the complex interaction between light and geometry by using a rendered shading reference to spatially modulate the DDIM. We achieve state-of-the-art performance on standard benchmark Multi-PIE and can photorealistically relight in-the-wild images. Please visit our page: https://diffusion-face-relighting.github.io
PDF
点此查看论文截图
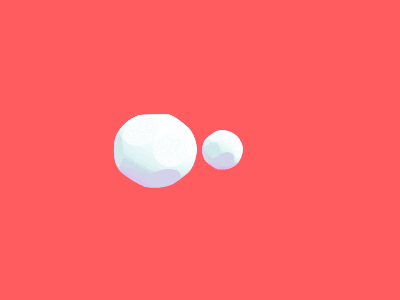
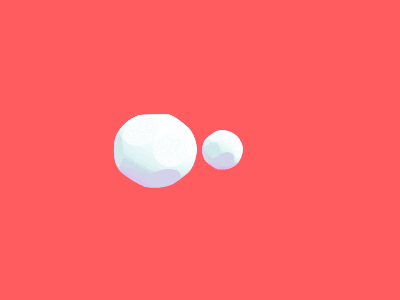
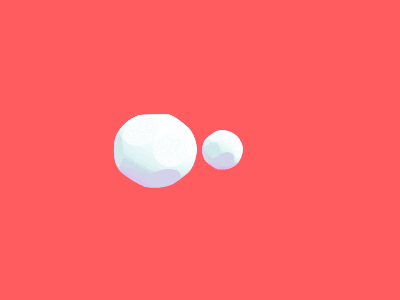
IDQL: Implicit Q-Learning as an Actor-Critic Method with Diffusion Policies
Authors:Philippe Hansen-Estruch, Ilya Kostrikov, Michael Janner, Jakub Grudzien Kuba, Sergey Levine
Effective offline RL methods require properly handling out-of-distribution actions. Implicit Q-learning (IQL) addresses this by training a Q-function using only dataset actions through a modified Bellman backup. However, it is unclear which policy actually attains the values represented by this implicitly trained Q-function. In this paper, we reinterpret IQL as an actor-critic method by generalizing the critic objective and connecting it to a behavior-regularized implicit actor. This generalization shows how the induced actor balances reward maximization and divergence from the behavior policy, with the specific loss choice determining the nature of this tradeoff. Notably, this actor can exhibit complex and multimodal characteristics, suggesting issues with the conditional Gaussian actor fit with advantage weighted regression (AWR) used in prior methods. Instead, we propose using samples from a diffusion parameterized behavior policy and weights computed from the critic to then importance sampled our intended policy. We introduce Implicit Diffusion Q-learning (IDQL), combining our general IQL critic with the policy extraction method. IDQL maintains the ease of implementation of IQL while outperforming prior offline RL methods and demonstrating robustness to hyperparameters. Code is available at https://github.com/philippe-eecs/IDQL.
PDF 11 Pages, 6 Figures, 3 Tables
点此查看论文截图
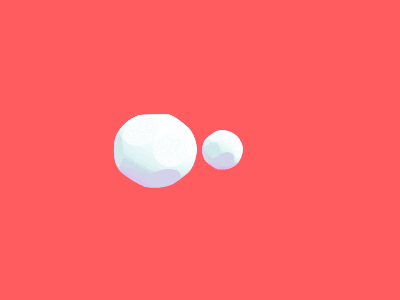
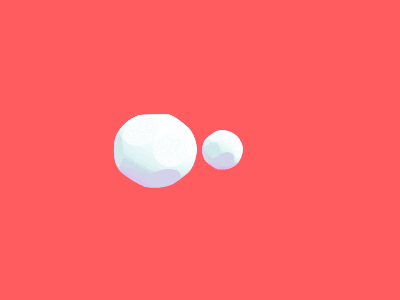
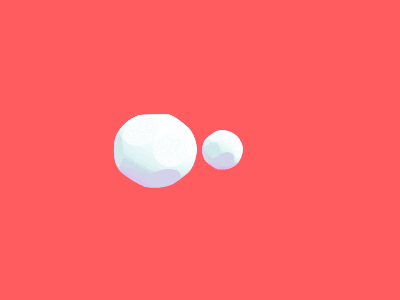
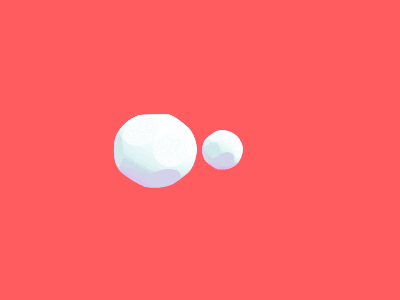
Omni-Line-of-Sight Imaging for Holistic Shape Reconstruction
Authors:Binbin Huang, Xingyue Peng, Siyuan Shen, Suan Xia, Ruiqian Li, Yanhua Yu, Yuehan Wang, Shenghua Gao, Wenzheng Chen, Shiying Li, Jingyi Yu
We introduce Omni-LOS, a neural computational imaging method for conducting holistic shape reconstruction (HSR) of complex objects utilizing a Single-Photon Avalanche Diode (SPAD)-based time-of-flight sensor. As illustrated in Fig. 1, our method enables new capabilities to reconstruct near-$360^\circ$ surrounding geometry of an object from a single scan spot. In such a scenario, traditional line-of-sight (LOS) imaging methods only see the front part of the object and typically fail to recover the occluded back regions. Inspired by recent advances of non-line-of-sight (NLOS) imaging techniques which have demonstrated great power to reconstruct occluded objects, Omni-LOS marries LOS and NLOS together, leveraging their complementary advantages to jointly recover the holistic shape of the object from a single scan position. The core of our method is to put the object nearby diffuse walls and augment the LOS scan in the front view with the NLOS scans from the surrounding walls, which serve as virtual ``mirrors’’ to trap lights toward the object. Instead of separately recovering the LOS and NLOS signals, we adopt an implicit neural network to represent the object, analogous to NeRF and NeTF. While transients are measured along straight rays in LOS but over the spherical wavefronts in NLOS, we derive differentiable ray propagation models to simultaneously model both types of transient measurements so that the NLOS reconstruction also takes into account the direct LOS measurements and vice versa. We further develop a proof-of-concept Omni-LOS hardware prototype for real-world validation. Comprehensive experiments on various wall settings demonstrate that Omni-LOS successfully resolves shape ambiguities caused by occlusions, achieves high-fidelity 3D scan quality, and manages to recover objects of various scales and complexity.
PDF
点此查看论文截图
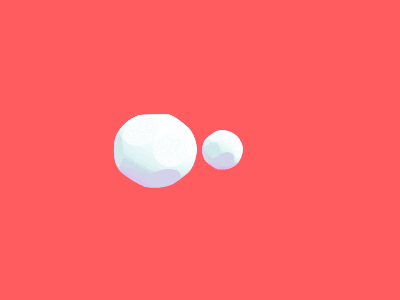
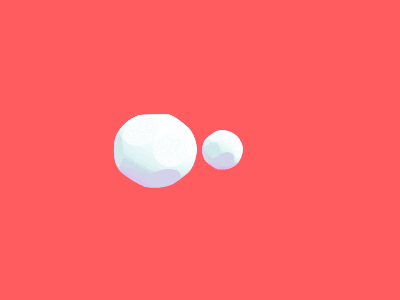
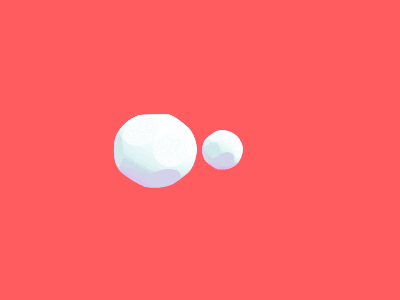
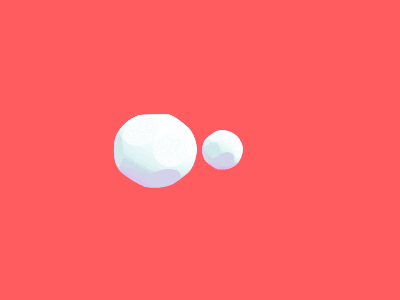
Improved Diffusion-based Image Colorization via Piggybacked Models
Authors:Hanyuan Liu, Jinbo Xing, Minshan Xie, Chengze Li, Tien-Tsin Wong
Image colorization has been attracting the research interests of the community for decades. However, existing methods still struggle to provide satisfactory colorized results given grayscale images due to a lack of human-like global understanding of colors. Recently, large-scale Text-to-Image (T2I) models have been exploited to transfer the semantic information from the text prompts to the image domain, where text provides a global control for semantic objects in the image. In this work, we introduce a colorization model piggybacking on the existing powerful T2I diffusion model. Our key idea is to exploit the color prior knowledge in the pre-trained T2I diffusion model for realistic and diverse colorization. A diffusion guider is designed to incorporate the pre-trained weights of the latent diffusion model to output a latent color prior that conforms to the visual semantics of the grayscale input. A lightness-aware VQVAE will then generate the colorized result with pixel-perfect alignment to the given grayscale image. Our model can also achieve conditional colorization with additional inputs (e.g. user hints and texts). Extensive experiments show that our method achieves state-of-the-art performance in terms of perceptual quality.
PDF project page: https://piggyback-color.github.io/
点此查看论文截图
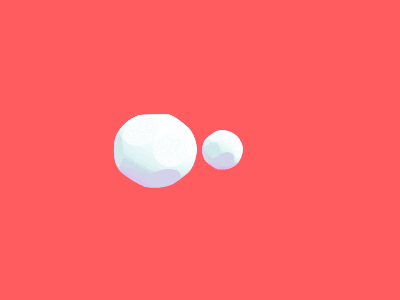
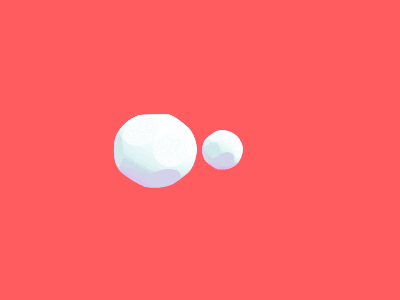
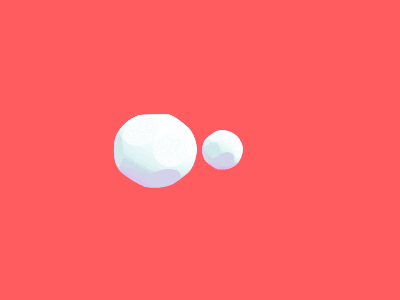
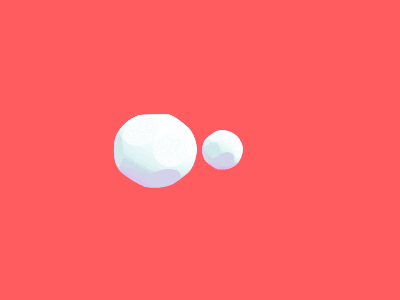
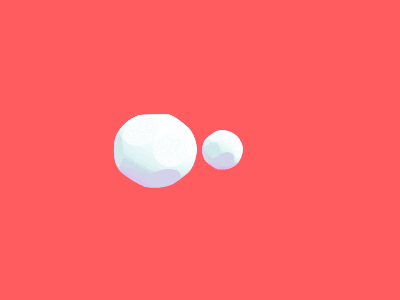
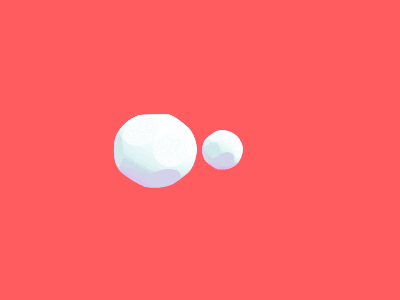
BoDiffusion: Diffusing Sparse Observations for Full-Body Human Motion Synthesis
Authors:Angela Castillo, Maria Escobar, Guillaume Jeanneret, Albert Pumarola, Pablo Arbeláez, Ali Thabet, Artsiom Sanakoyeu
Mixed reality applications require tracking the user’s full-body motion to enable an immersive experience. However, typical head-mounted devices can only track head and hand movements, leading to a limited reconstruction of full-body motion due to variability in lower body configurations. We propose BoDiffusion — a generative diffusion model for motion synthesis to tackle this under-constrained reconstruction problem. We present a time and space conditioning scheme that allows BoDiffusion to leverage sparse tracking inputs while generating smooth and realistic full-body motion sequences. To the best of our knowledge, this is the first approach that uses the reverse diffusion process to model full-body tracking as a conditional sequence generation task. We conduct experiments on the large-scale motion-capture dataset AMASS and show that our approach outperforms the state-of-the-art approaches by a significant margin in terms of full-body motion realism and joint reconstruction error.
PDF
点此查看论文截图
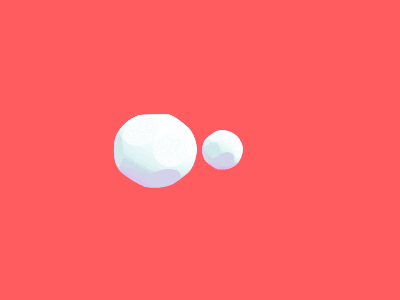
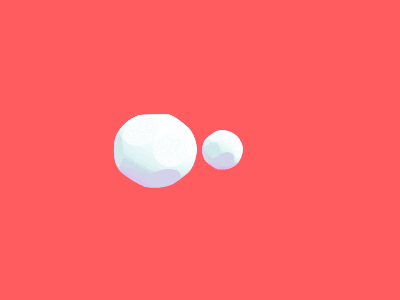
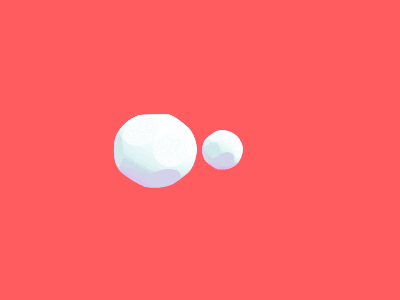
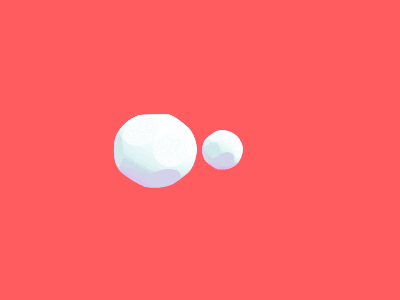
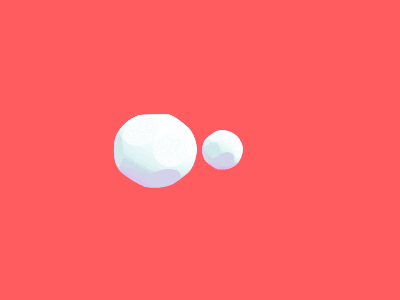
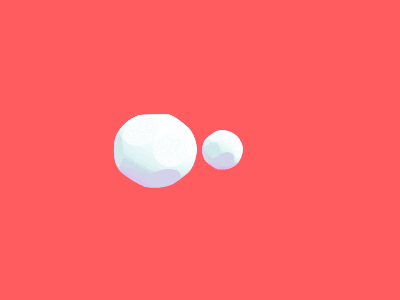