2023-04-21 更新
Adaptive Stylization Modulation for Domain Generalized Semantic Segmentation
Authors:Gabriel Tjio, Ping Liu, Chee-Keong Kwoh, Joey Tianyi Zhou
Obtaining sufficient labelled data for model training is impractical for most real-life applications. Therefore, we address the problem of domain generalization for semantic segmentation tasks to reduce the need to acquire and label additional data. Recent work on domain generalization increase data diversity by varying domain-variant features such as colour, style and texture in images. However, excessive stylization or even uniform stylization may reduce performance. Performance reduction is especially pronounced for pixels from minority classes, which are already more challenging to classify compared to pixels from majority classes. Therefore, we introduce a module, $ASH_{+}$, that modulates stylization strength for each pixel depending on the pixel’s semantic content. In this work, we also introduce a parameter that balances the element-wise and channel-wise proportion of stylized features with the original source domain features in the stylized source domain images. This learned parameter replaces an empirically determined global hyperparameter, allowing for more fine-grained control over the output stylized image. We conduct multiple experiments to validate the effectiveness of our proposed method. Finally, we evaluate our model on the publicly available benchmark semantic segmentation datasets (Cityscapes and SYNTHIA). Quantitative and qualitative comparisons indicate that our approach is competitive with state-of-the-art. Code is made available at \url{https://github.com/placeholder}
PDF
点此查看论文截图
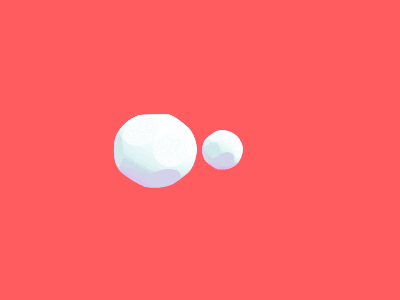
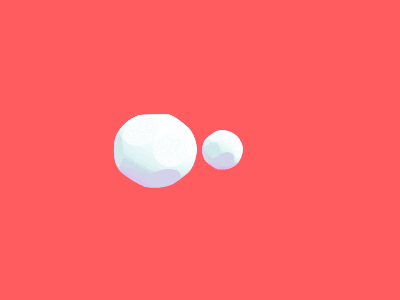
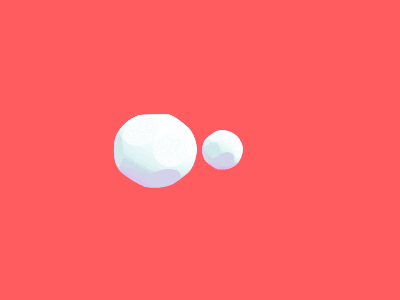
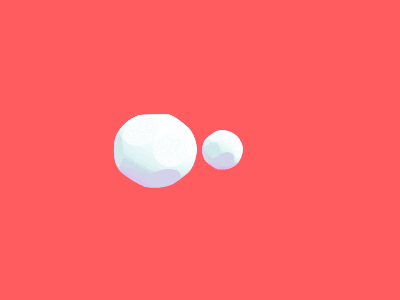
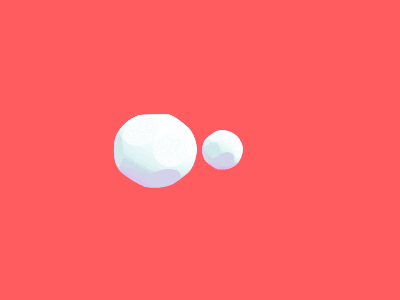
MARS: Model-agnostic Biased Object Removal without Additional Supervision for Weakly-Supervised Semantic Segmentation
Authors:Sanghyun Jo, In-Jae Yu, Kyungsu Kim
Weakly-supervised semantic segmentation aims to reduce labeling costs by training semantic segmentation models using weak supervision, such as image-level class labels. However, most approaches struggle to produce accurate localization maps and suffer from false predictions in class-related backgrounds (i.e., biased objects), such as detecting a railroad with the train class. Recent methods that remove biased objects require additional supervision for manually identifying biased objects for each problematic class and collecting their datasets by reviewing predictions, limiting their applicability to the real-world dataset with multiple labels and complex relationships for biasing. Following the first observation that biased features can be separated and eliminated by matching biased objects with backgrounds in the same dataset, we propose a fully-automatic/model-agnostic biased removal framework called MARS (Model-Agnostic biased object Removal without additional Supervision), which utilizes semantically consistent features of an unsupervised technique to eliminate biased objects in pseudo labels. Surprisingly, we show that MARS achieves new state-of-the-art results on two popular benchmarks, PASCAL VOC 2012 (val: 77.7%, test: 77.2%) and MS COCO 2014 (val: 49.4%), by consistently improving the performance of various WSSS models by at least 30% without additional supervision.
PDF
点此查看论文截图
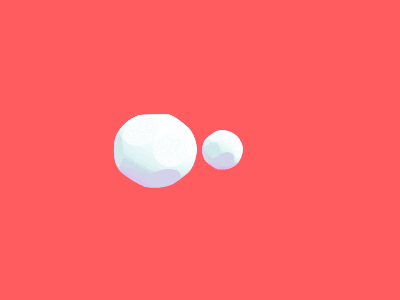
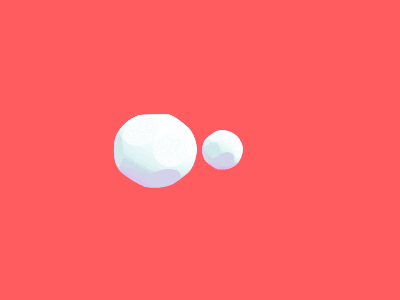
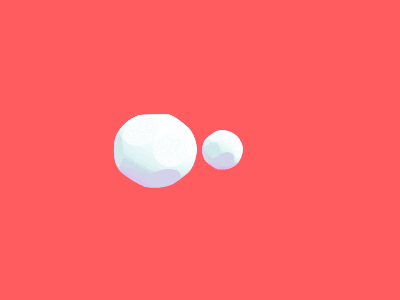
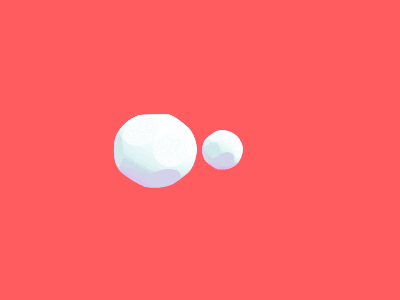
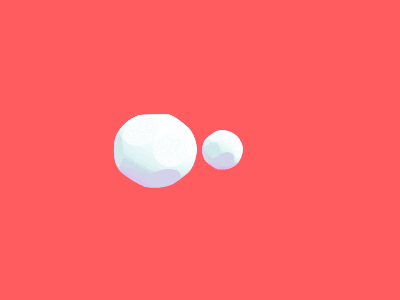