2023-04-13 更新
CamDiff: Camouflage Image Augmentation via Diffusion Model
Authors:Xue-Jing Luo, Shuo Wang, Zongwei Wu, Christos Sakaridis, Yun Cheng, Deng-Ping Fan, Luc Van Gool
The burgeoning field of camouflaged object detection (COD) seeks to identify objects that blend into their surroundings. Despite the impressive performance of recent models, we have identified a limitation in their robustness, where existing methods may misclassify salient objects as camouflaged ones, despite these two characteristics being contradictory. This limitation may stem from lacking multi-pattern training images, leading to less saliency robustness. To address this issue, we introduce CamDiff, a novel approach inspired by AI-Generated Content (AIGC) that overcomes the scarcity of multi-pattern training images. Specifically, we leverage the latent diffusion model to synthesize salient objects in camouflaged scenes, while using the zero-shot image classification ability of the Contrastive Language-Image Pre-training (CLIP) model to prevent synthesis failures and ensure the synthesized object aligns with the input prompt. Consequently, the synthesized image retains its original camouflage label while incorporating salient objects, yielding camouflage samples with richer characteristics. The results of user studies show that the salient objects in the scenes synthesized by our framework attract the user’s attention more; thus, such samples pose a greater challenge to the existing COD models. Our approach enables flexible editing and efficient large-scale dataset generation at a low cost. It significantly enhances COD baselines’ training and testing phases, emphasizing robustness across diverse domains. Our newly-generated datasets and source code are available at https://github.com/drlxj/CamDiff.
PDF
点此查看论文截图
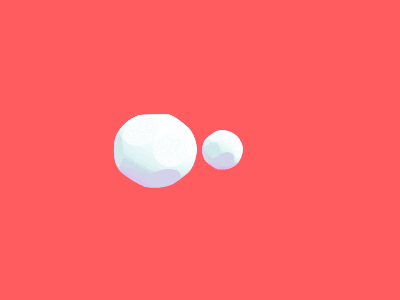
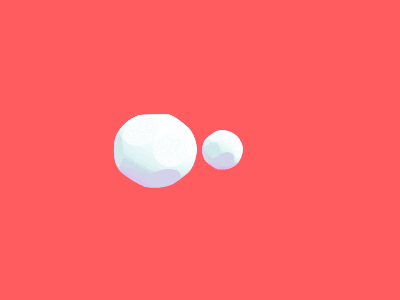
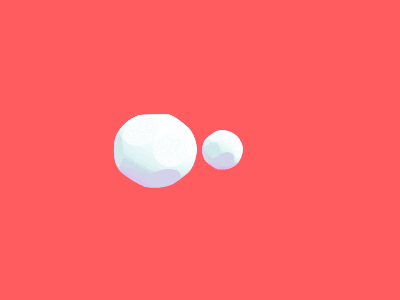
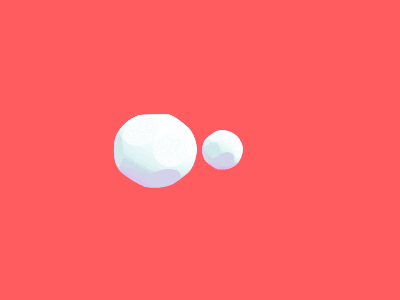
Improving Diffusion Models for Scene Text Editing with Dual Encoders
Authors:Jiabao Ji, Guanhua Zhang, Zhaowen Wang, Bairu Hou, Zhifei Zhang, Brian Price, Shiyu Chang
Scene text editing is a challenging task that involves modifying or inserting specified texts in an image while maintaining its natural and realistic appearance. Most previous approaches to this task rely on style-transfer models that crop out text regions and feed them into image transfer models, such as GANs. However, these methods are limited in their ability to change text style and are unable to insert texts into images. Recent advances in diffusion models have shown promise in overcoming these limitations with text-conditional image editing. However, our empirical analysis reveals that state-of-the-art diffusion models struggle with rendering correct text and controlling text style. To address these problems, we propose DIFFSTE to improve pre-trained diffusion models with a dual encoder design, which includes a character encoder for better text legibility and an instruction encoder for better style control. An instruction tuning framework is introduced to train our model to learn the mapping from the text instruction to the corresponding image with either the specified style or the style of the surrounding texts in the background. Such a training method further brings our method the zero-shot generalization ability to the following three scenarios: generating text with unseen font variation, e.g., italic and bold, mixing different fonts to construct a new font, and using more relaxed forms of natural language as the instructions to guide the generation task. We evaluate our approach on five datasets and demonstrate its superior performance in terms of text correctness, image naturalness, and style controllability. Our code is publicly available. https://github.com/UCSB-NLP-Chang/DiffSTE
PDF 22 pages, 19 figures
点此查看论文截图
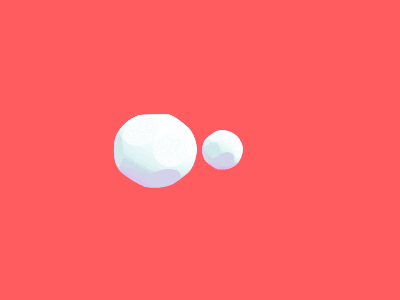
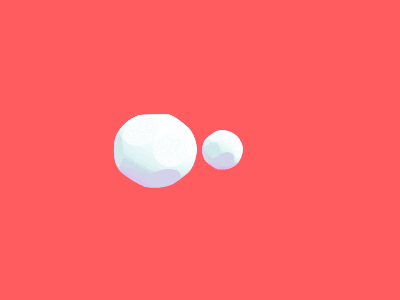
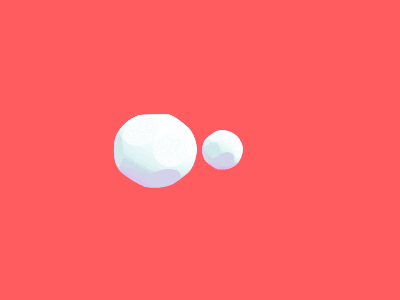
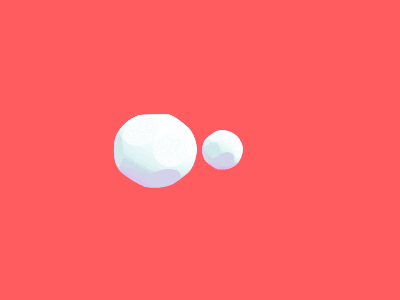
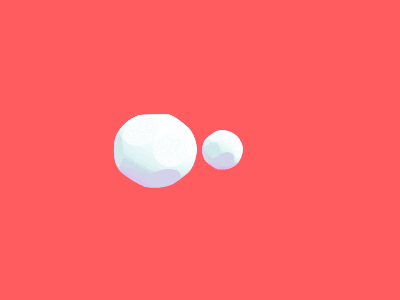
Exploring Diffusion Models for Unsupervised Video Anomaly Detection
Authors:Anil Osman Tur, Nicola Dall’Asen, Cigdem Beyan, Elisa Ricci
This paper investigates the performance of diffusion models for video anomaly detection (VAD) within the most challenging but also the most operational scenario in which the data annotations are not used. As being sparse, diverse, contextual, and often ambiguous, detecting abnormal events precisely is a very ambitious task. To this end, we rely only on the information-rich spatio-temporal data, and the reconstruction power of the diffusion models such that a high reconstruction error is utilized to decide the abnormality. Experiments performed on two large-scale video anomaly detection datasets demonstrate the consistent improvement of the proposed method over the state-of-the-art generative models while in some cases our method achieves better scores than the more complex models. This is the first study using a diffusion model and examining its parameters’ influence to present guidance for VAD in surveillance scenarios.
PDF Submitted to IEEE ICIP 2023
点此查看论文截图
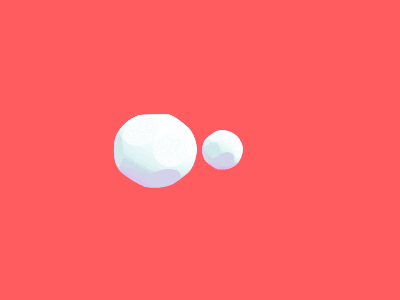
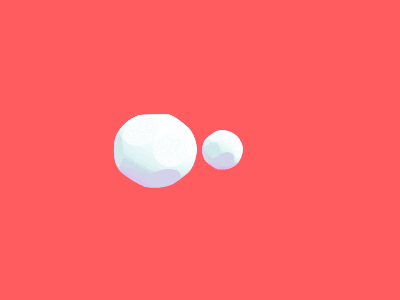
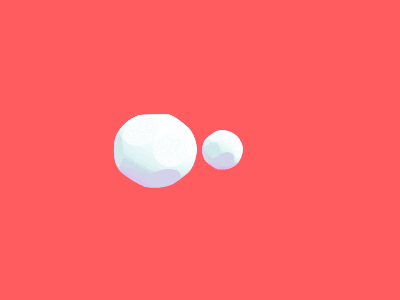
Diffusion models with location-scale noise
Authors:Alexia Jolicoeur-Martineau, Kilian Fatras, Ke Li, Tal Kachman
Diffusion Models (DMs) are powerful generative models that add Gaussian noise to the data and learn to remove it. We wanted to determine which noise distribution (Gaussian or non-Gaussian) led to better generated data in DMs. Since DMs do not work by design with non-Gaussian noise, we built a framework that allows reversing a diffusion process with non-Gaussian location-scale noise. We use that framework to show that the Gaussian distribution performs the best over a wide range of other distributions (Laplace, Uniform, t, Generalized-Gaussian).
PDF
点此查看论文截图
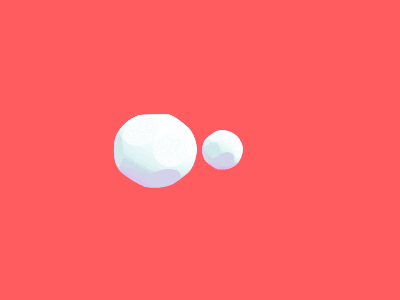
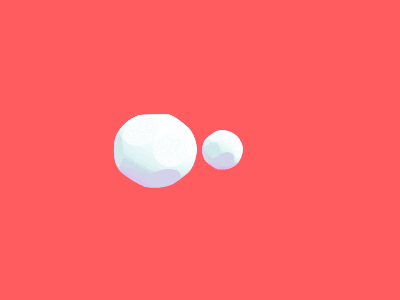
SpectralDiff: Hyperspectral Image Classification with Spectral-Spatial Diffusion Models
Authors:Ning Chen, Jun Yue, Leyuan Fang, Shaobo Xia
Hyperspectral image (HSI) classification is an important topic in the field of remote sensing, and has a wide range of applications in Earth science. HSIs contain hundreds of continuous bands, which are characterized by high dimension and high correlation between adjacent bands. The high dimension and redundancy of HSI data bring great difficulties to HSI classification. In recent years, a large number of HSI feature extraction and classification methods based on deep learning have been proposed. However, their ability to model the global relationships among samples in both spatial and spectral domains is still limited. In order to solve this problem, an HSI classification method with spectral-spatial diffusion models is proposed. The proposed method realizes the reconstruction of spectral-spatial distribution of the training samples with the forward and reverse spectral-spatial diffusion process, thus modeling the global spatial-spectral relationship between samples. Then, we use the spectral-spatial denoising network of the reverse process to extract the unsupervised diffusion features. Features extracted by the spectral-spatial diffusion models can achieve cross-sample perception from the reconstructed distribution of the training samples, thus obtaining better classification performance. Experiments on three public HSI datasets show that the proposed method can achieve better performance than the state-of-the-art methods. The source code and the pre-trained spectral-spatial diffusion model will be publicly available at https://github.com/chenning0115/SpectralDiff.
PDF This work has been submitted to the IEEE for possible publication. Copyright may be transferred without notice, after which this version may no longer be accessible
点此查看论文截图
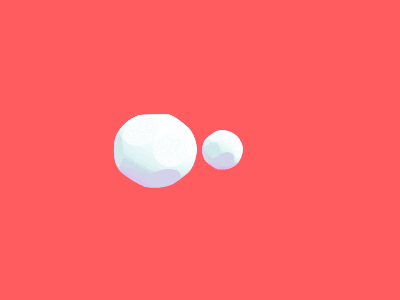
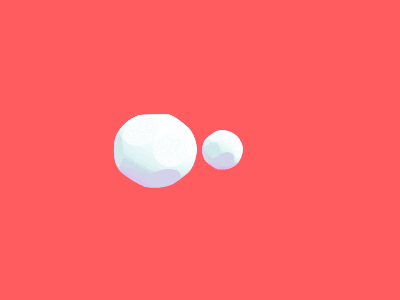
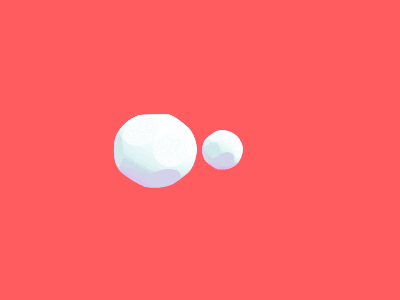
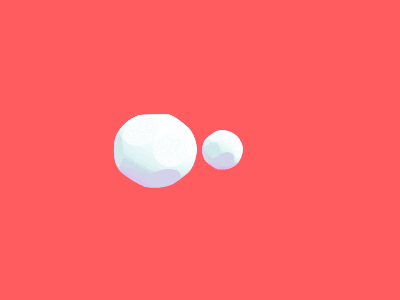
DreamPose: Fashion Image-to-Video Synthesis via Stable Diffusion
Authors:Johanna Karras, Aleksander Holynski, Ting-Chun Wang, Ira Kemelmacher-Shlizerman
We present DreamPose, a diffusion-based method for generating animated fashion videos from still images. Given an image and a sequence of human body poses, our method synthesizes a video containing both human and fabric motion. To achieve this, we transform a pretrained text-to-image model (Stable Diffusion) into a pose-and-image guided video synthesis model, using a novel finetuning strategy, a set of architectural changes to support the added conditioning signals, and techniques to encourage temporal consistency. We fine-tune on a collection of fashion videos from the UBC Fashion dataset. We evaluate our method on a variety of clothing styles and poses, and demonstrate that our method produces state-of-the-art results on fashion video animation. Video results are available on our project page.
PDF Project page: https://grail.cs.washington.edu/projects/dreampose/
点此查看论文截图
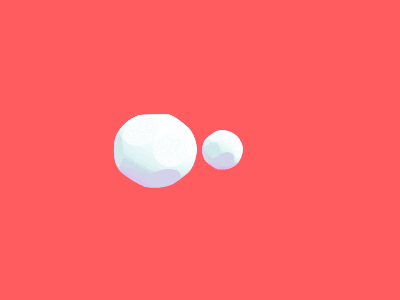
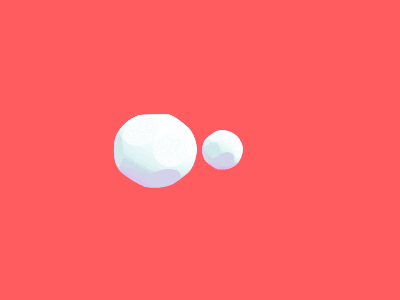
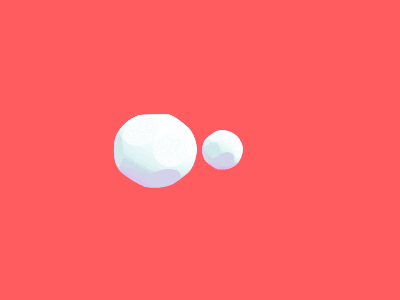
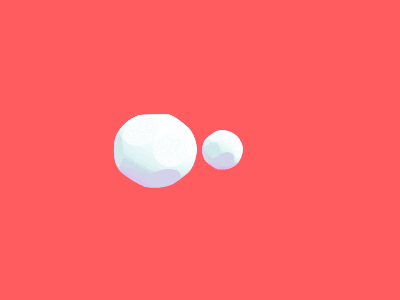
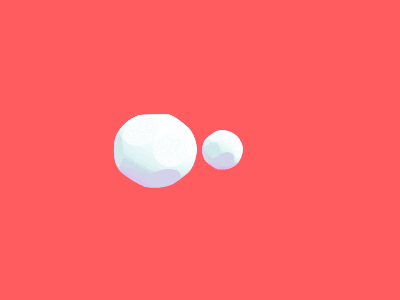
Continual Diffusion: Continual Customization of Text-to-Image Diffusion with C-LoRA
Authors:James Seale Smith, Yen-Chang Hsu, Lingyu Zhang, Ting Hua, Zsolt Kira, Yilin Shen, Hongxia Jin
Recent works demonstrate a remarkable ability to customize text-to-image diffusion models while only providing a few example images. What happens if you try to customize such models using multiple, fine-grained concepts in a sequential (i.e., continual) manner? In our work, we show that recent state-of-the-art customization of text-to-image models suffer from catastrophic forgetting when new concepts arrive sequentially. Specifically, when adding a new concept, the ability to generate high quality images of past, similar concepts degrade. To circumvent this forgetting, we propose a new method, C-LoRA, composed of a continually self-regularized low-rank adaptation in cross attention layers of the popular Stable Diffusion model. Furthermore, we use customization prompts which do not include the word of the customized object (i.e., “person” for a human face dataset) and are initialized as completely random embeddings. Importantly, our method induces only marginal additional parameter costs and requires no storage of user data for replay. We show that C-LoRA not only outperforms several baselines for our proposed setting of text-to-image continual customization, which we refer to as Continual Diffusion, but that we achieve a new state-of-the-art in the well-established rehearsal-free continual learning setting for image classification. The high achieving performance of C-LoRA in two separate domains positions it as a compelling solution for a wide range of applications, and we believe it has significant potential for practical impact.
PDF Project page: https://jamessealesmith.github.io/continual-diffusion/
点此查看论文截图
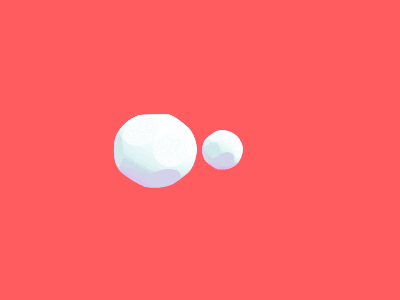
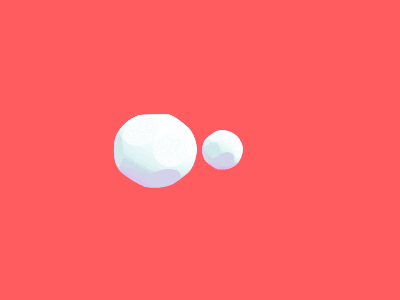
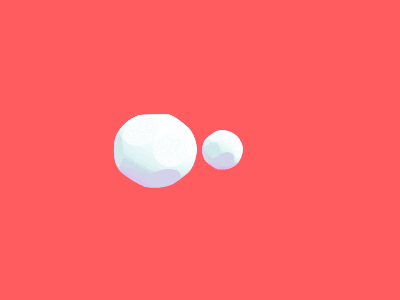
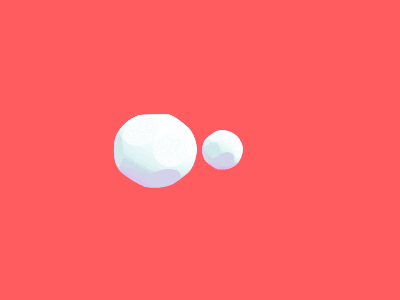