2023-04-03 更新
GlyphDraw: Learning to Draw Chinese Characters in Image Synthesis Models Coherently
Authors:Jian Ma, Mingjun Zhao, Chen Chen, Ruichen Wang, Di Niu, Haonan Lu, Xiaodong Lin
Recent breakthroughs in the field of language-guided image generation have yielded impressive achievements, enabling the creation of high-quality and diverse images based on user instructions. Although the synthesis performance is fascinating, one significant limitation of current image generation models is their insufficient ability to generate coherent text within images, particularly for complex glyph structures like Chinese characters. To address this problem, we introduce GlyphDraw, a general learning framework aiming at endowing image generation models with the capacity to generate images embedded with coherent text. To the best of our knowledge, this is the first work in the field of image synthesis to address the generation of Chinese characters. % we first adopt the OCR technique to collect images with Chinese characters as training samples, and extract the text and locations as auxiliary information. We first sophisticatedly design the image-text dataset’s construction strategy, then build our model specifically on a diffusion-based image generator and carefully modify the network structure to allow the model to learn drawing Chinese characters with the help of glyph and position information. Furthermore, we maintain the model’s open-domain image synthesis capability by preventing catastrophic forgetting by using a variety of training techniques. Extensive qualitative and quantitative experiments demonstrate that our method not only produces accurate Chinese characters as in prompts, but also naturally blends the generated text into the background. Please refer to https://1073521013.github.io/glyph-draw.github.io
PDF 24 pages, 5 figures
点此查看论文截图
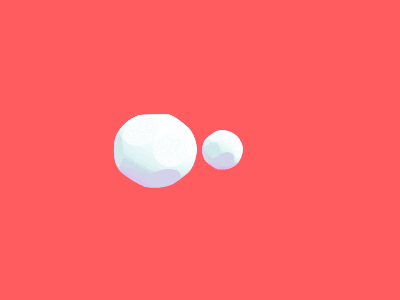
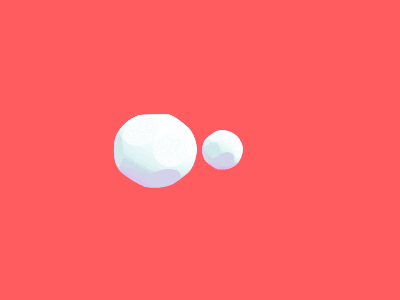
Pay Attention: Accuracy Versus Interpretability Trade-off in Fine-tuned Diffusion Models
Authors:Mischa Dombrowski, Hadrien Reynaud, Johanna P. Müller, Matthew Baugh, Bernhard Kainz
The recent progress of diffusion models in terms of image quality has led to a major shift in research related to generative models. Current approaches often fine-tune pre-trained foundation models using domain-specific text-to-image pairs. This approach is straightforward for X-ray image generation due to the high availability of radiology reports linked to specific images. However, current approaches hardly ever look at attention layers to verify whether the models understand what they are generating. In this paper, we discover an important trade-off between image fidelity and interpretability in generative diffusion models. In particular, we show that fine-tuning text-to-image models with learnable text encoder leads to a lack of interpretability of diffusion models. Finally, we demonstrate the interpretability of diffusion models by showing that keeping the language encoder frozen, enables diffusion models to achieve state-of-the-art phrase grounding performance on certain diseases for a challenging multi-label segmentation task, without any additional training. Code and models will be available at https://github.com/MischaD/chest-distillation.
PDF
点此查看论文截图
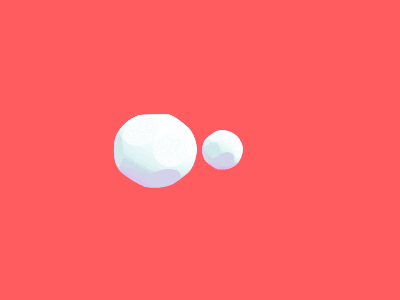
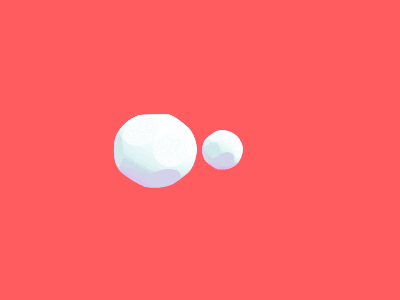
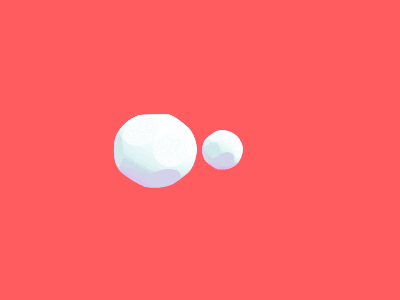
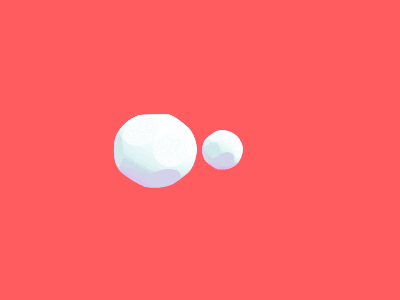
Diffusion Action Segmentation
Authors:Daochang Liu, Qiyue Li, AnhDung Dinh, Tingting Jiang, Mubarak Shah, Chang Xu
Temporal action segmentation is crucial for understanding long-form videos. Previous works on this task commonly adopt an iterative refinement paradigm by using multi-stage models. Our paper proposes an essentially different framework via denoising diffusion models, which nonetheless shares the same inherent spirit of such iterative refinement. In this framework, action predictions are progressively generated from random noise with input video features as conditions. To enhance the modeling of three striking characteristics of human actions, including the position prior, the boundary ambiguity, and the relational dependency, we devise a unified masking strategy for the conditioning inputs in our framework. Extensive experiments on three benchmark datasets, i.e., GTEA, 50Salads, and Breakfast, are performed and the proposed method achieves superior or comparable results to state-of-the-art methods, showing the effectiveness of a generative approach for action segmentation. Our codes will be made available.
PDF
点此查看论文截图
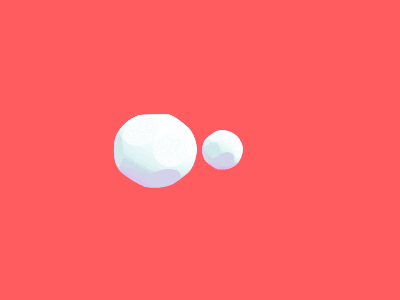
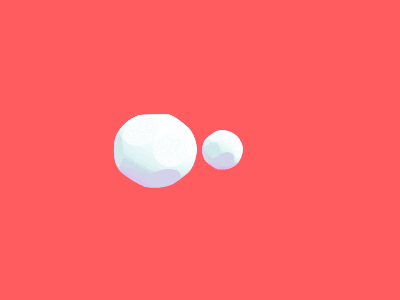
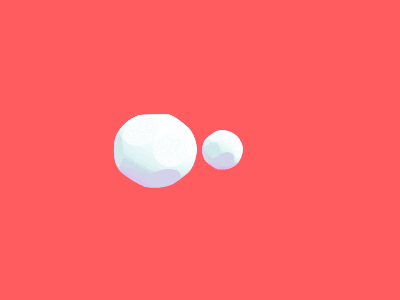
One-shot Unsupervised Domain Adaptation with Personalized Diffusion Models
Authors:Yasser Benigmim, Subhankar Roy, Slim Essid, Vicky Kalogeiton, Stéphane Lathuilière
Adapting a segmentation model from a labeled source domain to a target domain, where a single unlabeled datum is available, is one the most challenging problems in domain adaptation and is otherwise known as one-shot unsupervised domain adaptation (OSUDA). Most of the prior works have addressed the problem by relying on style transfer techniques, where the source images are stylized to have the appearance of the target domain. Departing from the common notion of transferring only the target ``texture’’ information, we leverage text-to-image diffusion models (e.g., Stable Diffusion) to generate a synthetic target dataset with photo-realistic images that not only faithfully depict the style of the target domain, but are also characterized by novel scenes in diverse contexts. The text interface in our method Data AugmenTation with diffUsion Models (DATUM) endows us with the possibility of guiding the generation of images towards desired semantic concepts while respecting the original spatial context of a single training image, which is not possible in existing OSUDA methods. Extensive experiments on standard benchmarks show that our DATUM surpasses the state-of-the-art OSUDA methods by up to +7.1%. The implementation is available at https://github.com/yasserben/DATUM
PDF 13 pages, 8 figures, 5 tables
点此查看论文截图
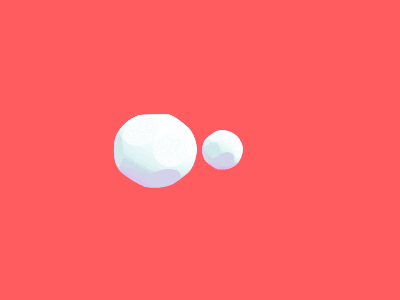
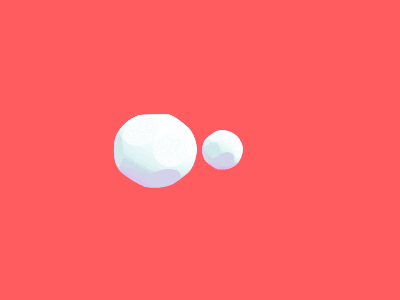
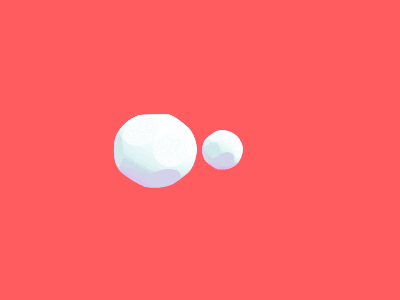
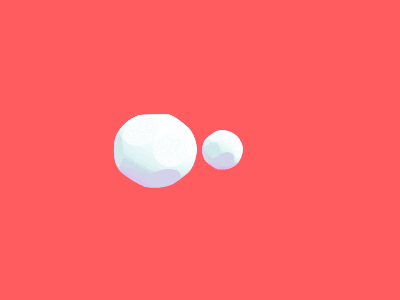
A Closer Look at Parameter-Efficient Tuning in Diffusion Models
Authors:Chendong Xiang, Fan Bao, Chongxuan Li, Hang Su, Jun Zhu
Large-scale diffusion models like Stable Diffusion are powerful and find various real-world applications while customizing such models by fine-tuning is both memory and time inefficient. Motivated by the recent progress in natural language processing, we investigate parameter-efficient tuning in large diffusion models by inserting small learnable modules (termed adapters). In particular, we decompose the design space of adapters into orthogonal factors — the input position, the output position as well as the function form, and perform Analysis of Variance (ANOVA), a classical statistical approach for analyzing the correlation between discrete (design options) and continuous variables (evaluation metrics). Our analysis suggests that the input position of adapters is the critical factor influencing the performance of downstream tasks. Then, we carefully study the choice of the input position, and we find that putting the input position after the cross-attention block can lead to the best performance, validated by additional visualization analyses. Finally, we provide a recipe for parameter-efficient tuning in diffusion models, which is comparable if not superior to the fully fine-tuned baseline (e.g., DreamBooth) with only 0.75 \% extra parameters, across various customized tasks.
PDF 8pages
点此查看论文截图
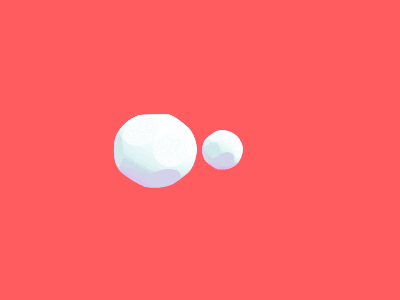
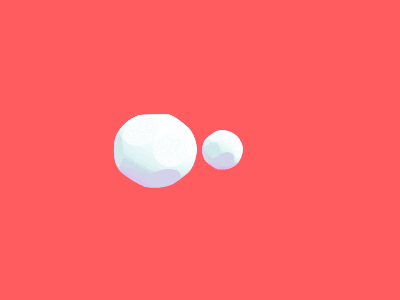
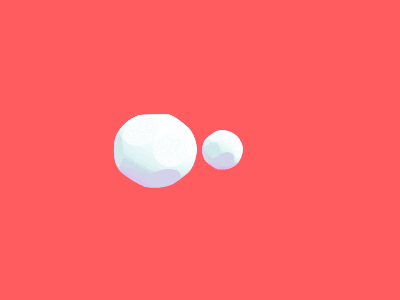
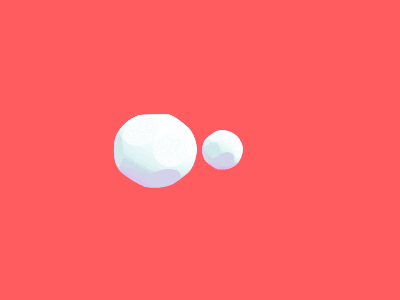
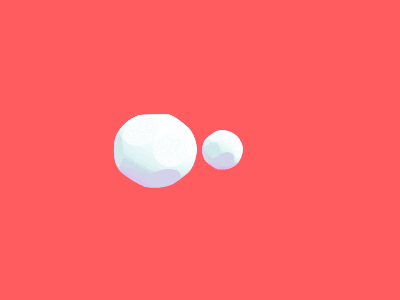