2023-03-30 更新
Domain Adaptive Semantic Segmentation by Optimal Transport
Authors:Yaqian Guo, Xin Wang, Ce Li, Shihui Ying
Scene segmentation is widely used in the field of autonomous driving for environment perception, and semantic scene segmentation (3S) has received a great deal of attention due to the richness of the semantic information it contains. It aims to assign labels to pixels in an image, thus enabling automatic image labeling. Current approaches are mainly based on convolutional neural networks (CNN), but they rely on a large number of labels. Therefore, how to use a small size of labeled data to achieve semantic segmentation becomes more and more important. In this paper, we propose a domain adaptation (DA) framework based on optimal transport (OT) and attention mechanism to address this issue. Concretely, first we generate the output space via CNN due to its superiority of feature representation. Second, we utilize OT to achieve a more robust alignment of source and target domains in output space, where the OT plan defines a well attention mechanism to improve the adaptation of the model. In particular, with OT, the number of network parameters has been reduced and the network has been better interpretable. Third, to better describe the multi-scale property of features, we construct a multi-scale segmentation network to perform domain adaptation. Finally, in order to verify the performance of our proposed method, we conduct experimental comparison with three benchmark and four SOTA methods on three scene datasets, and the mean intersection-over-union (mIOU) has been significant improved, and visualization results under multiple domain adaptation scenarios also show that our proposed method has better performance than compared semantic segmentation methods.
PDF
点此查看论文截图
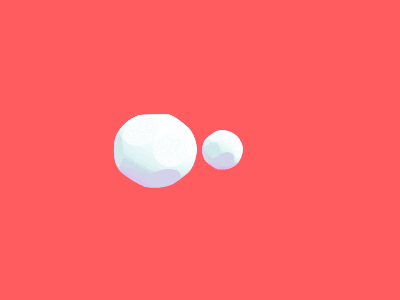
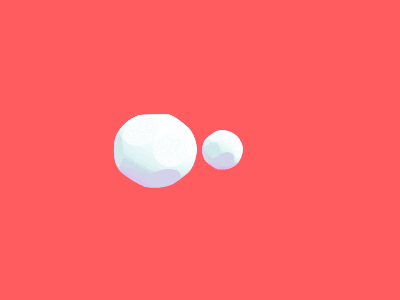
Global Adaptation meets Local Generalization: Unsupervised Domain Adaptation for 3D Human Pose Estimation
Authors:Wenhao Chai, Zhongyu Jiang, Jenq-Neng Hwang, Gaoang Wang
When applying a pre-trained 2D-to-3D human pose lifting model to a target unseen dataset, large performance degradation is commonly encountered due to domain shift issues. We observe that the degradation is caused by two factors: 1) the large distribution gap over global positions of poses between the source and target datasets due to variant camera parameters and settings, and 2) the deficient diversity of local structures of poses in training. To this end, we combine \textbf{global adaptation} and \textbf{local generalization} in \textit{PoseDA}, a simple yet effective framework of unsupervised domain adaptation for 3D human pose estimation. Specifically, global adaptation aims to align global positions of poses from the source domain to the target domain with a proposed global position alignment (GPA) module. And local generalization is designed to enhance the diversity of 2D-3D pose mapping with a local pose augmentation (LPA) module. These modules bring significant performance improvement without introducing additional learnable parameters. In addition, we propose local pose augmentation (LPA) to enhance the diversity of 3D poses following an adversarial training scheme consisting of 1) a augmentation generator that generates the parameters of pre-defined pose transformations and 2) an anchor discriminator to ensure the reality and quality of the augmented data. Our approach can be applicable to almost all 2D-3D lifting models. \textit{PoseDA} achieves 61.3 mm of MPJPE on MPI-INF-3DHP under a cross-dataset evaluation setup, improving upon the previous state-of-the-art method by 10.2\%.
PDF
点此查看论文截图
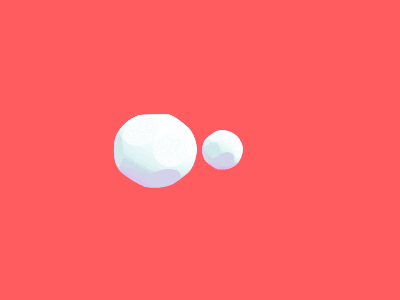
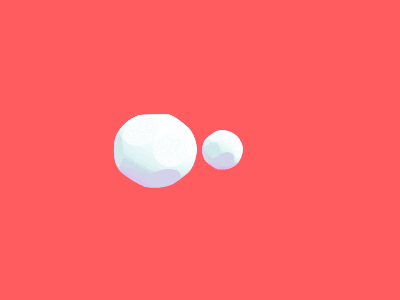
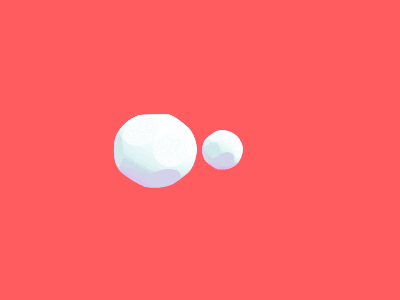
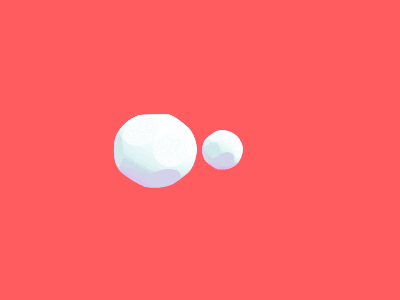
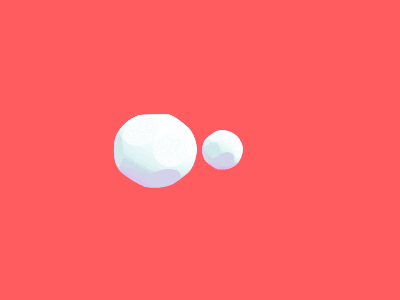
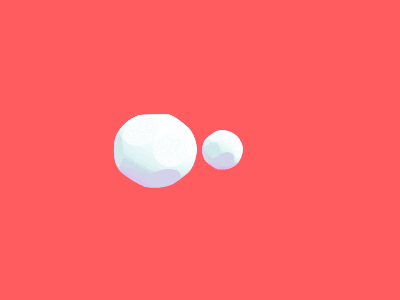
AVFormer: Injecting Vision into Frozen Speech Models for Zero-Shot AV-ASR
Authors:Paul Hongsuck Seo, Arsha Nagrani, Cordelia Schmid
Audiovisual automatic speech recognition (AV-ASR) aims to improve the robustness of a speech recognition system by incorporating visual information. Training fully supervised multimodal models for this task from scratch, however is limited by the need for large labelled audiovisual datasets (in each downstream domain of interest). We present AVFormer, a simple method for augmenting audio-only models with visual information, at the same time performing lightweight domain adaptation. We do this by (i) injecting visual embeddings into a frozen ASR model using lightweight trainable adaptors. We show that these can be trained on a small amount of weakly labelled video data with minimum additional training time and parameters. (ii) We also introduce a simple curriculum scheme during training which we show is crucial to enable the model to jointly process audio and visual information effectively; and finally (iii) we show that our model achieves state of the art zero-shot results on three different AV-ASR benchmarks (How2, VisSpeech and Ego4D), while also crucially preserving decent performance on traditional audio-only speech recognition benchmarks (LibriSpeech). Qualitative results show that our model effectively leverages visual information for robust speech recognition.
PDF CVPR 2023
点此查看论文截图
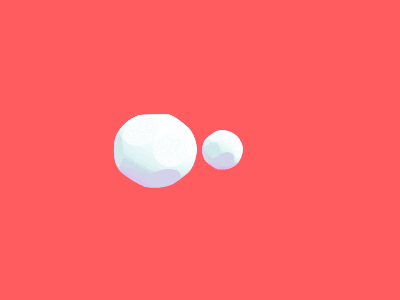
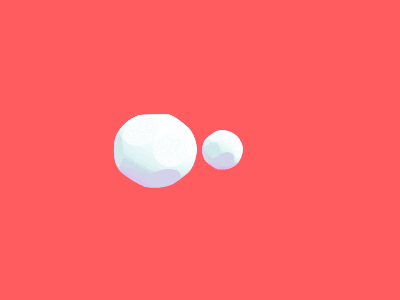
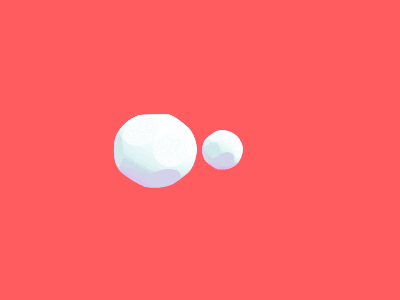
TTA-COPE: Test-Time Adaptation for Category-Level Object Pose Estimation
Authors:Taeyeop Lee, Jonathan Tremblay, Valts Blukis, Bowen Wen, Byeong-Uk Lee, Inkyu Shin, Stan Birchfield, In So Kweon, Kuk-Jin Yoon
Test-time adaptation methods have been gaining attention recently as a practical solution for addressing source-to-target domain gaps by gradually updating the model without requiring labels on the target data. In this paper, we propose a method of test-time adaptation for category-level object pose estimation called TTA-COPE. We design a pose ensemble approach with a self-training loss using pose-aware confidence. Unlike previous unsupervised domain adaptation methods for category-level object pose estimation, our approach processes the test data in a sequential, online manner, and it does not require access to the source domain at runtime. Extensive experimental results demonstrate that the proposed pose ensemble and the self-training loss improve category-level object pose performance during test time under both semi-supervised and unsupervised settings. Project page: https://taeyeop.com/ttacope
PDF Accepted to CVPR 2023, Project page: https://taeyeop.com/ttacope
点此查看论文截图
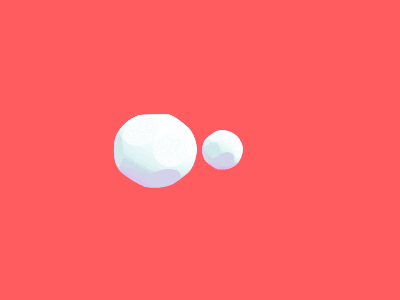
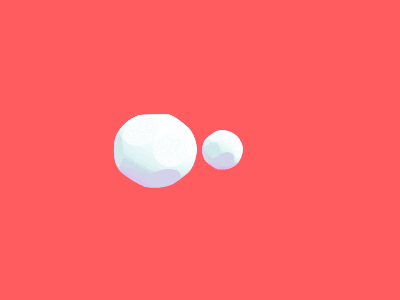
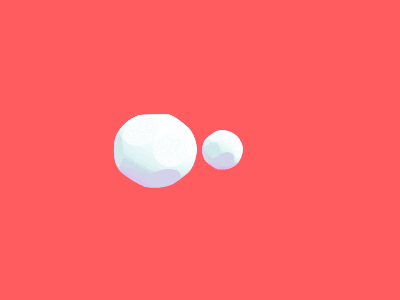
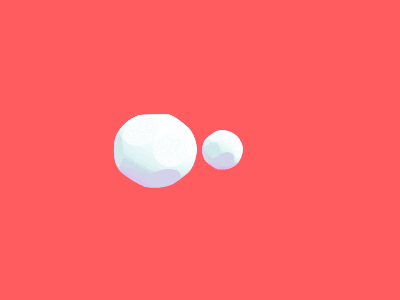
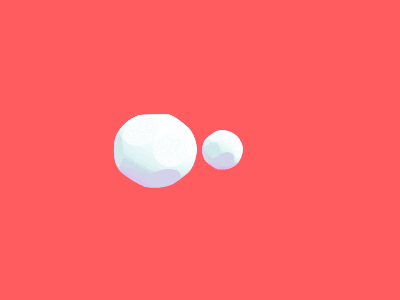