2023-03-22 更新
Self-Paced Learning for Open-Set Domain Adaptation
Authors:Xinghong Liu, Yi Zhou, Tao Zhou, Jie Qin, Shengcai Liao
Domain adaptation tackles the challenge of generalizing knowledge acquired from a source domain to a target domain with different data distributions. Traditional domain adaptation methods presume that the classes in the source and target domains are identical, which is not always the case in real-world scenarios. Open-set domain adaptation (OSDA) addresses this limitation by allowing previously unseen classes in the target domain. Open-set domain adaptation aims to not only recognize target samples belonging to common classes shared by source and target domains but also perceive unknown class samples. We propose a novel framework based on self-paced learning to distinguish common and unknown class samples precisely, referred to as SPLOS (self-paced learning for open-set). To utilize unlabeled target samples for self-paced learning, we generate pseudo labels and design a cross-domain mixup method tailored for OSDA scenarios. This strategy minimizes the noise from pseudo labels and ensures our model progressively learns common class features of the target domain, beginning with simpler examples and advancing to more complex ones. Furthermore, unlike existing OSDA methods that require manual hyperparameter $threshold$ tuning to separate common and unknown classes, our approach self-tunes a suitable threshold, eliminating the need for empirical tuning during testing. Comprehensive experiments illustrate that our method consistently achieves superior performance on different benchmarks compared with various state-of-the-art methods.
PDF
点此查看论文截图
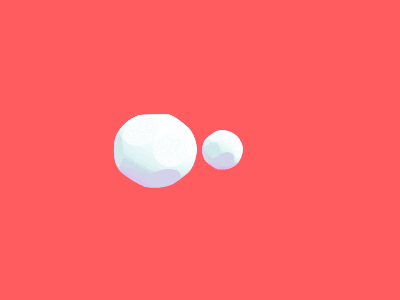
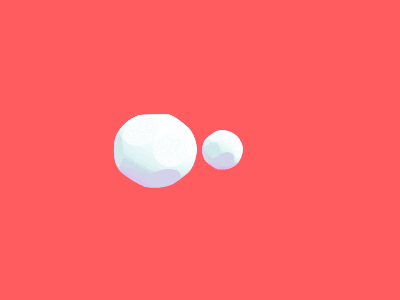
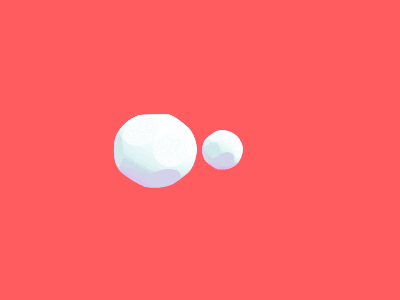
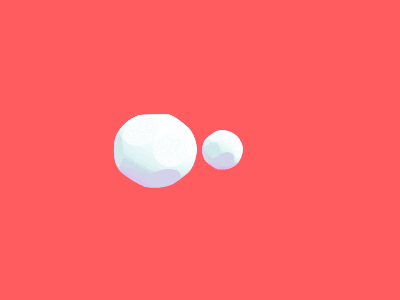
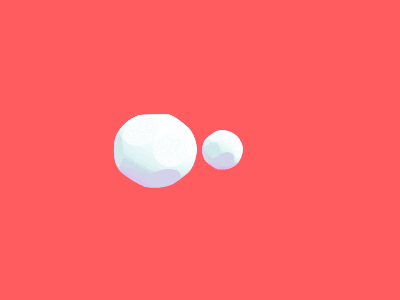
Linking generative semi-supervised learning and generative open-set recognition
Authors:Emile Reyn Engelbrecht, Johan du Preez
This study investigates the relationship between semi-supervised learning (SSL) and open-set recognition (OSR) in the context of generative adversarial networks (GANs). Although no previous study has formally linked SSL and OSR, their respective methods share striking similarities. Specifically, SSL-GANs and OSR-GANs require generator to produce samples in the complementary space. Subsequently, by regularising networks with generated samples, both SSL and OSR classifiers generalize the open space. To demonstrate the connection between SSL and OSR, we theoretically and experimentally compare state-of-the-art SSL-GAN methods with state-of-the-art OSR-GAN methods. Our results indicate that the SSL optimised margin-GANs, which have a stronger foundation in literature, set the new standard for the combined SSL-OSR task and achieves new state-of-other art results in certain general OSR experiments. However, the OSR optimised adversarial reciprocal point (ARP)-GANs still slightly out-performed margin-GANs at other OSR experiments. This result indicates unique insights for the combined optimisation task of SSL-OSR.
PDF
点此查看论文截图
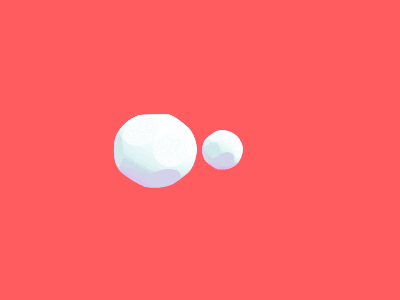
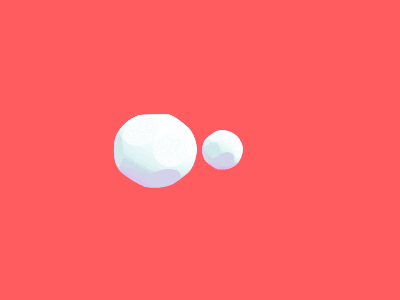
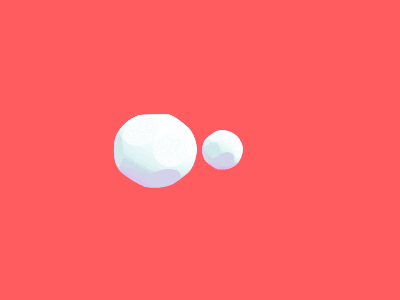