2023-03-13 更新
Boosting Adversarial Attacks by Leveraging Decision Boundary Information
Authors:Boheng Zeng, LianLi Gao, QiLong Zhang, ChaoQun Li, JingKuan Song, ShuaiQi Jing
Due to the gap between a substitute model and a victim model, the gradient-based noise generated from a substitute model may have low transferability for a victim model since their gradients are different. Inspired by the fact that the decision boundaries of different models do not differ much, we conduct experiments and discover that the gradients of different models are more similar on the decision boundary than in the original position. Moreover, since the decision boundary in the vicinity of an input image is flat along most directions, we conjecture that the boundary gradients can help find an effective direction to cross the decision boundary of the victim models. Based on it, we propose a Boundary Fitting Attack to improve transferability. Specifically, we introduce a method to obtain a set of boundary points and leverage the gradient information of these points to update the adversarial examples. Notably, our method can be combined with existing gradient-based methods. Extensive experiments prove the effectiveness of our method, i.e., improving the success rate by 5.6% against normally trained CNNs and 14.9% against defense CNNs on average compared to state-of-the-art transfer-based attacks. Further we compare transformers with CNNs, the results indicate that transformers are more robust than CNNs. However, our method still outperforms existing methods when attacking transformers. Specifically, when using CNNs as substitute models, our method obtains an average attack success rate of 58.2%, which is 10.8% higher than other state-of-the-art transfer-based attacks.
PDF
点此查看论文截图
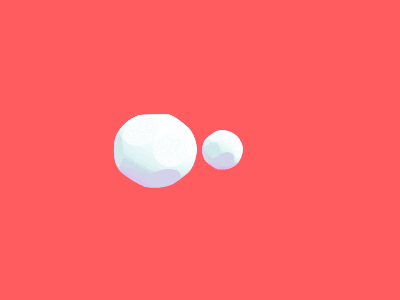
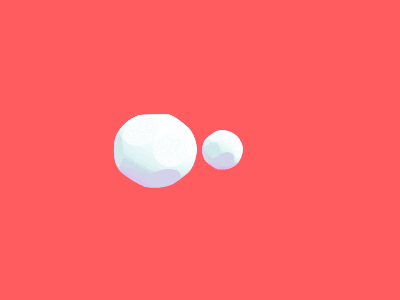
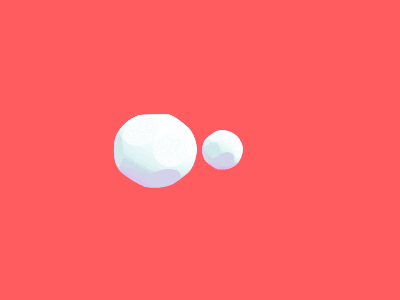
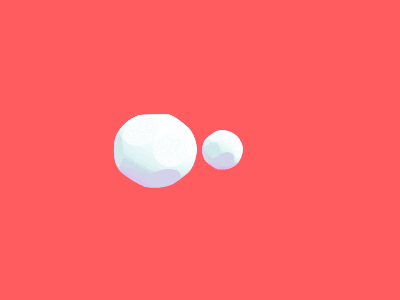
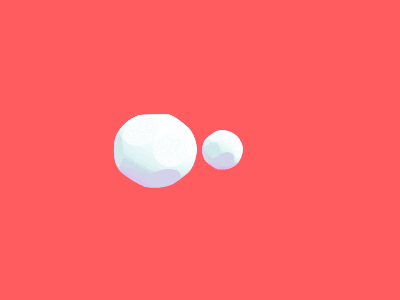
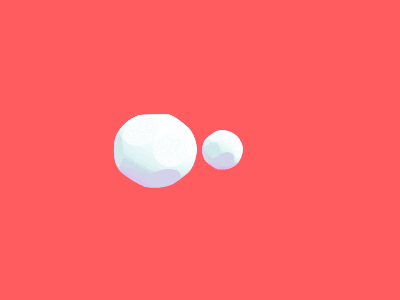
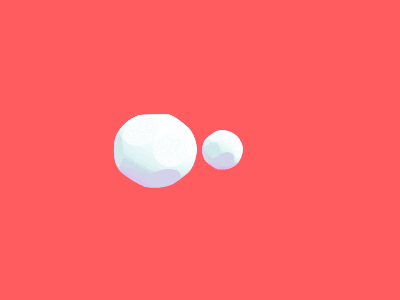
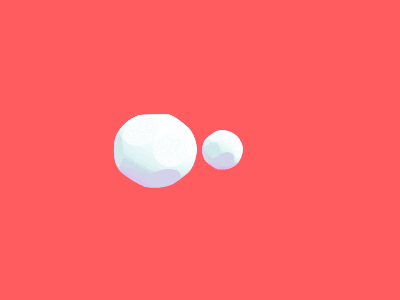
TrojDiff: Trojan Attacks on Diffusion Models with Diverse Targets
Authors:Weixin Chen, Dawn Song, Bo Li
Diffusion models have achieved great success in a range of tasks, such as image synthesis and molecule design. As such successes hinge on large-scale training data collected from diverse sources, the trustworthiness of these collected data is hard to control or audit. In this work, we aim to explore the vulnerabilities of diffusion models under potential training data manipulations and try to answer: How hard is it to perform Trojan attacks on well-trained diffusion models? What are the adversarial targets that such Trojan attacks can achieve? To answer these questions, we propose an effective Trojan attack against diffusion models, TrojDiff, which optimizes the Trojan diffusion and generative processes during training. In particular, we design novel transitions during the Trojan diffusion process to diffuse adversarial targets into a biased Gaussian distribution and propose a new parameterization of the Trojan generative process that leads to an effective training objective for the attack. In addition, we consider three types of adversarial targets: the Trojaned diffusion models will always output instances belonging to a certain class from the in-domain distribution (In-D2D attack), out-of-domain distribution (Out-D2D-attack), and one specific instance (D2I attack). We evaluate TrojDiff on CIFAR-10 and CelebA datasets against both DDPM and DDIM diffusion models. We show that TrojDiff always achieves high attack performance under different adversarial targets using different types of triggers, while the performance in benign environments is preserved. The code is available at https://github.com/chenweixin107/TrojDiff.
PDF CVPR2023
点此查看论文截图
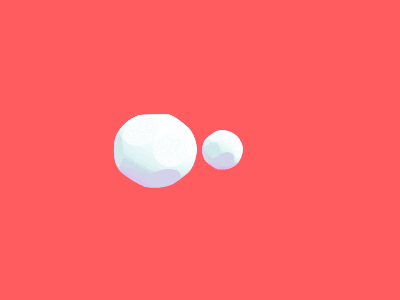
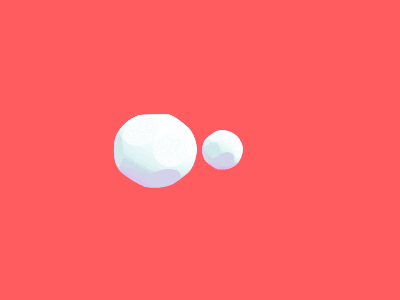
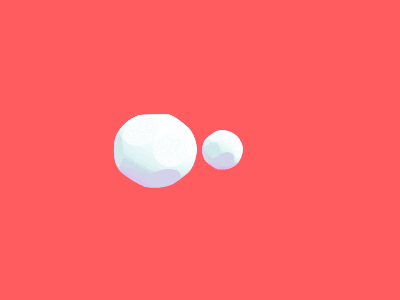