2023-03-09 更新
Soft labelling for semantic segmentation: Bringing coherence to label down-sampling
Authors:Roberto Alcover-Couso, Marcos Escudero-Vinolo, Juan C. SanMiguel, Jose M. Martinez
In semantic segmentation, training data down-sampling is commonly performed due to limited resources, the need to adapt image size to the model input, or improve data augmentation. This down-sampling typically employs different strategies for the image data and the annotated labels. Such discrepancy leads to mismatches between the down-sampled color and label images. Hence, the training performance significantly decreases as the down-sampling factor increases. In this paper, we bring together the down-sampling strategies for the image data and the training labels. To that aim, we propose a novel framework for label down-sampling via soft-labeling that better conserves label information after down-sampling. Therefore, fully aligning soft-labels with image data to keep the distribution of the sampled pixels. This proposal also produces reliable annotations for under-represented semantic classes. Altogether, it allows training competitive models at lower resolutions. Experiments show that the proposal outperforms other down-sampling strategies. Moreover, state-of-the-art performance is achieved for reference benchmarks, but employing significantly less computational resources than foremost approaches. This proposal enables competitive research for semantic segmentation under resource constraints.
PDF
点此查看论文截图
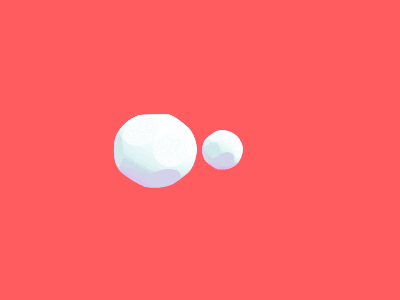
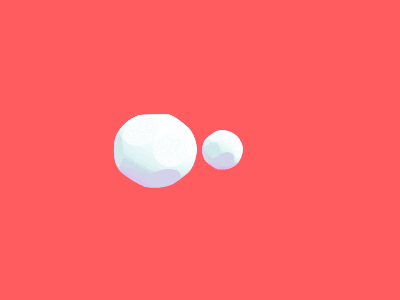
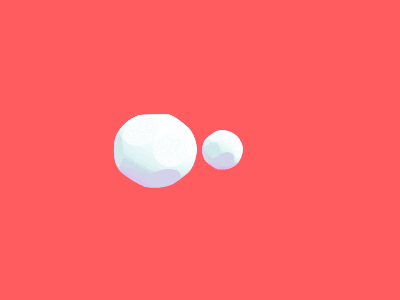
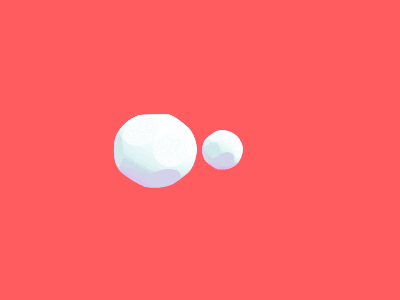
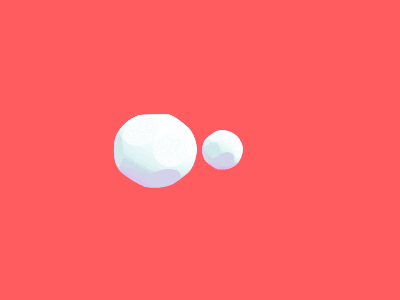
GLOW: Global Layout Aware Attacks on Object Detection
Authors:Buyu Liu, BaoJun, Jianping Fan, Xi Peng, Kui Ren, Jun Yu
Adversarial attacks aim to perturb images such that a predictor outputs incorrect results. Due to the limited research in structured attacks, imposing consistency checks on natural multi-object scenes is a promising yet practical defense against conventional adversarial attacks. More desired attacks, to this end, should be able to fool defenses with such consistency checks. Therefore, we present the first approach GLOW that copes with various attack requests by generating global layout-aware adversarial attacks, in which both categorical and geometric layout constraints are explicitly established. Specifically, we focus on object detection task and given a victim image, GLOW first localizes victim objects according to target labels. And then it generates multiple attack plans, together with their context-consistency scores. Our proposed GLOW, on the one hand, is capable of handling various types of requests, including single or multiple victim objects, with or without specified victim objects. On the other hand, it produces a consistency score for each attack plan, reflecting the overall contextual consistency that both semantic category and global scene layout are considered. In experiment, we design multiple types of attack requests and validate our ideas on MS COCO and Pascal. Extensive experimental results demonstrate that we can achieve about 30$\%$ average relative improvement compared to state-of-the-art methods in conventional single object attack request; Moreover, our method outperforms SOTAs significantly on more generic attack requests by about 20$\%$ in average; Finally, our method produces superior performance under challenging zero-query black-box setting, or 20$\%$ better than SOTAs. Our code, model and attack requests would be made available.
PDF ICCV
点此查看论文截图
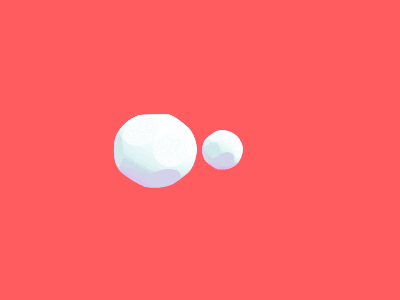
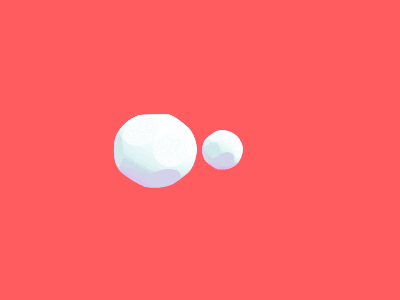
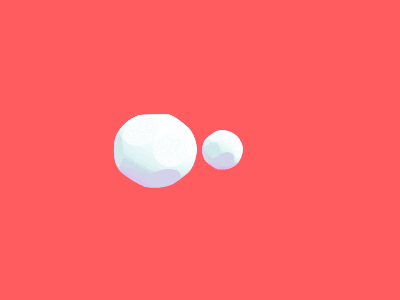
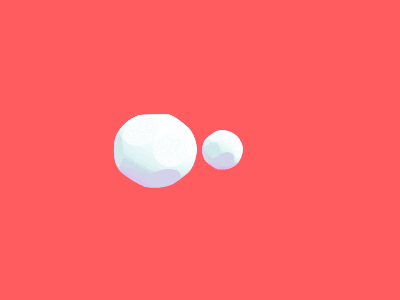
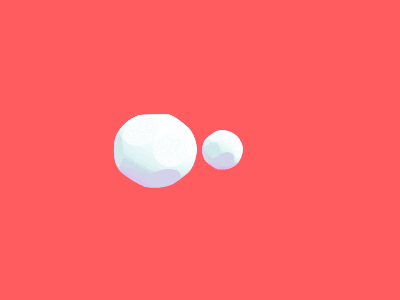
Radio astronomical images object detection and segmentation: A benchmark on deep learning methods
Authors:Renato Sortino, Daniel Magro, Giuseppe Fiameni, Eva Sciacca, Simone Riggi, Andrea DeMarco, Concetto Spampinato, Andrew M. Hopkins, Filomena Bufano, Francesco Schillirò, Cristobal Bordiu, Carmelo Pino
In recent years, deep learning has been successfully applied in various scientific domains. Following these promising results and performances, it has recently also started being evaluated in the domain of radio astronomy. In particular, since radio astronomy is entering the Big Data era, with the advent of the largest telescope in the world - the Square Kilometre Array (SKA), the task of automatic object detection and instance segmentation is crucial for source finding and analysis. In this work, we explore the performance of the most affirmed deep learning approaches, applied to astronomical images obtained by radio interferometric instrumentation, to solve the task of automatic source detection. This is carried out by applying models designed to accomplish two different kinds of tasks: object detection and semantic segmentation. The goal is to provide an overview of existing techniques, in terms of prediction performance and computational efficiency, to scientists in the astrophysics community who would like to employ machine learning in their research.
PDF
点此查看论文截图
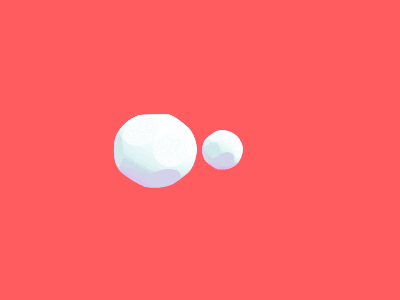