2023-03-07 更新
Learning to Localize in Unseen Scenes with Relative Pose Regressors
Authors:Ofer Idan, Yoli Shavit, Yosi Keller
Relative pose regressors (RPRs) localize a camera by estimating its relative translation and rotation to a pose-labelled reference. Unlike scene coordinate regression and absolute pose regression methods, which learn absolute scene parameters, RPRs can (theoretically) localize in unseen environments, since they only learn the residual pose between camera pairs. In practice, however, the performance of RPRs is significantly degraded in unseen scenes. In this work, we propose to aggregate paired feature maps into latent codes, instead of operating on global image descriptors, in order to improve the generalization of RPRs. We implement aggregation with concatenation, projection, and attention operations (Transformer Encoders) and learn to regress the relative pose parameters from the resulting latent codes. We further make use of a recently proposed continuous representation of rotation matrices, which alleviates the limitations of the commonly used quaternions. Compared to state-of-the-art RPRs, our model is shown to localize significantly better in unseen environments, across both indoor and outdoor benchmarks, while maintaining competitive performance in seen scenes. We validate our findings and architecture design through multiple ablations. Our code and pretrained models is publicly available.
PDF
点此查看论文截图
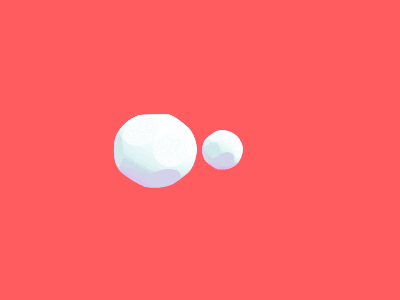
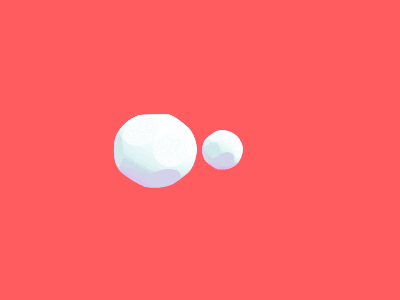
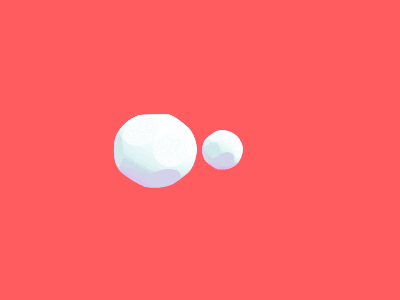
DwinFormer: Dual Window Transformers for End-to-End Monocular Depth Estimation
Authors:Md Awsafur Rahman, Shaikh Anowarul Fattah
Depth estimation from a single image is of paramount importance in the realm of computer vision, with a multitude of applications. Conventional methods suffer from the trade-off between consistency and fine-grained details due to the local-receptive field limiting their practicality. This lack of long-range dependency inherently comes from the convolutional neural network part of the architecture. In this paper, a dual window transformer-based network, namely DwinFormer, is proposed, which utilizes both local and global features for end-to-end monocular depth estimation. The DwinFormer consists of dual window self-attention and cross-attention transformers, Dwin-SAT and Dwin-CAT, respectively. The Dwin-SAT seamlessly extracts intricate, locally aware features while concurrently capturing global context. It harnesses the power of local and global window attention to adeptly capture both short-range and long-range dependencies, obviating the need for complex and computationally expensive operations, such as attention masking or window shifting. Moreover, Dwin-SAT introduces inductive biases which provide desirable properties, such as translational equvariance and less dependence on large-scale data. Furthermore, conventional decoding methods often rely on skip connections which may result in semantic discrepancies and a lack of global context when fusing encoder and decoder features. In contrast, the Dwin-CAT employs both local and global window cross-attention to seamlessly fuse encoder and decoder features with both fine-grained local and contextually aware global information, effectively amending semantic gap. Empirical evidence obtained through extensive experimentation on the NYU-Depth-V2 and KITTI datasets demonstrates the superiority of the proposed method, consistently outperforming existing approaches across both indoor and outdoor environments.
PDF
点此查看论文截图
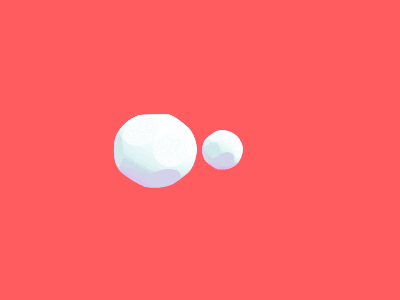
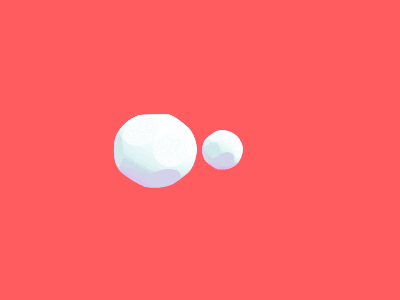
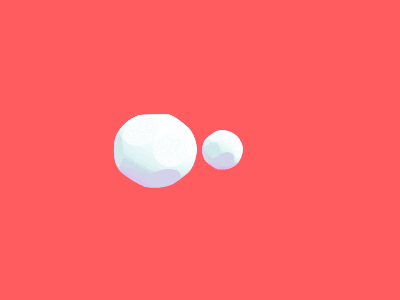
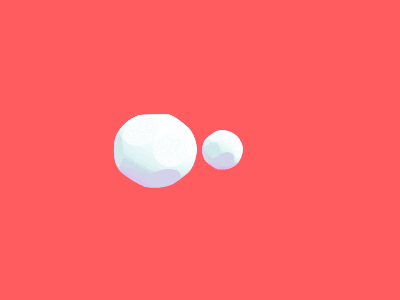
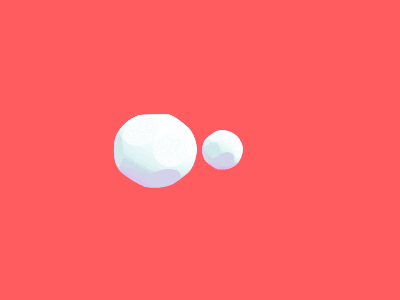