2023-03-06 更新
Semantic Attention Flow Fields for Dynamic Scene Decomposition
Authors:Yiqing Liang, Eliot Laidlaw, Alexander Meyerowitz, Srinath Sridhar, James Tompkin
We present SAFF: a dynamic neural volume reconstruction of a casual monocular video that consists of time-varying color, density, scene flow, semantics, and attention information. The semantics and attention let us identify salient foreground objects separately from the background in arbitrary spacetime views. We add two network heads to represent the semantic and attention information. For optimization, we design semantic attention pyramids from DINO-ViT outputs that trade detail with whole-image context. After optimization, we perform a saliency-aware clustering to decompose the scene. For evaluation on real-world dynamic scene decomposition across spacetime, we annotate object masks in the NVIDIA Dynamic Scene Dataset. We demonstrate that SAFF can decompose dynamic scenes without affecting RGB or depth reconstruction quality, that volume-integrated SAFF outperforms 2D baselines, and that SAFF improves foreground/background segmentation over recent static/dynamic split methods. Project Webpage: https://visual.cs.brown.edu/saff
PDF
点此查看论文截图
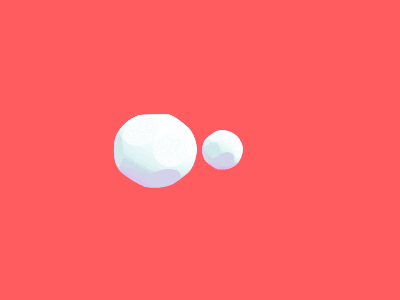
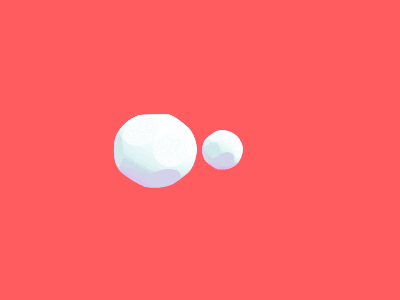
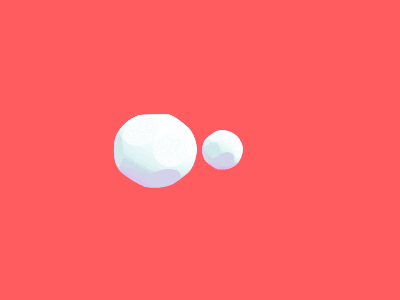
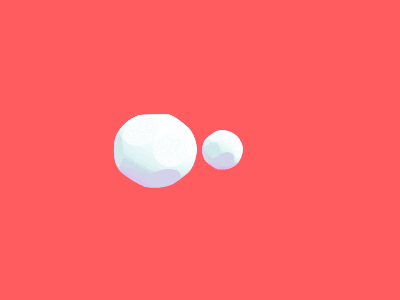
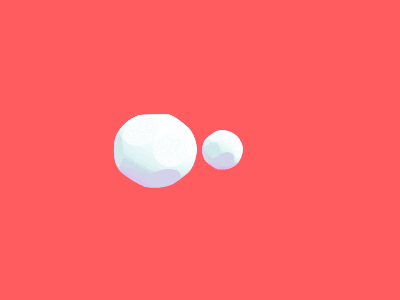
Prompt, Generate, then Cache: Cascade of Foundation Models makes Strong Few-shot Learners
Authors:Renrui Zhang, Xiangfei Hu, Bohao Li, Siyuan Huang, Hanqiu Deng, Hongsheng Li, Yu Qiao, Peng Gao
Visual recognition in low-data regimes requires deep neural networks to learn generalized representations from limited training samples. Recently, CLIP-based methods have shown promising few-shot performance benefited from the contrastive language-image pre-training. We then question, if the more diverse pre-training knowledge can be cascaded to further assist few-shot representation learning. In this paper, we propose CaFo, a Cascade of Foundation models that incorporates diverse prior knowledge of various pre-training paradigms for better few-shot learning. Our CaFo incorporates CLIP’s language-contrastive knowledge, DINO’s vision-contrastive knowledge, DALL-E’s vision-generative knowledge, and GPT-3’s language-generative knowledge. Specifically, CaFo works by ‘Prompt, Generate, then Cache’. Firstly, we leverage GPT-3 to produce textual inputs for prompting CLIP with rich downstream linguistic semantics. Then, we generate synthetic images via DALL-E to expand the few-shot training data without any manpower. At last, we introduce a learnable cache model to adaptively blend the predictions from CLIP and DINO. By such collaboration, CaFo can fully unleash the potential of different pre-training methods and unify them to perform state-of-the-art for few-shot classification. Code is available at https://github.com/ZrrSkywalker/CaFo.
PDF Accepted by CVPR 2023. Code is available at https://github.com/ZrrSkywalker/CaFo. arXiv admin note: substantial text overlap with arXiv:2209.12255
点此查看论文截图
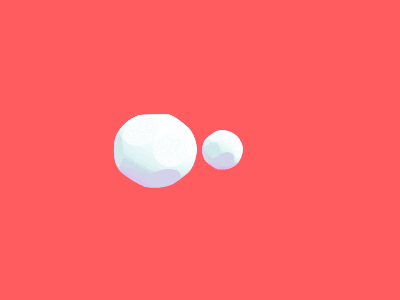
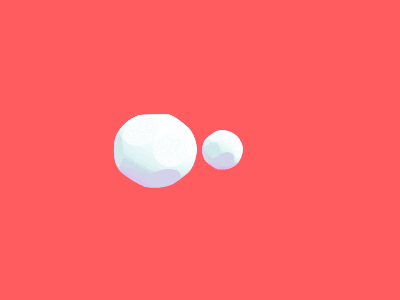
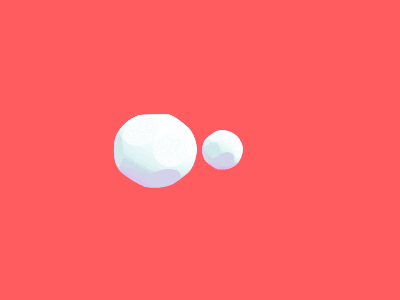
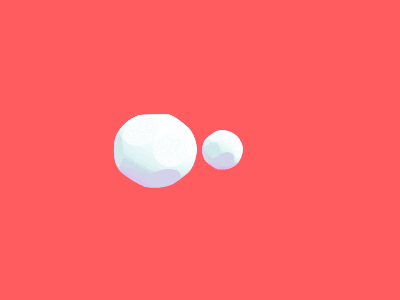
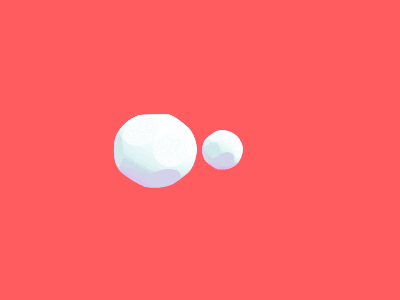
Unleashing Text-to-Image Diffusion Models for Visual Perception
Authors:Wenliang Zhao, Yongming Rao, Zuyan Liu, Benlin Liu, Jie Zhou, Jiwen Lu
Diffusion models (DMs) have become the new trend of generative models and have demonstrated a powerful ability of conditional synthesis. Among those, text-to-image diffusion models pre-trained on large-scale image-text pairs are highly controllable by customizable prompts. Unlike the unconditional generative models that focus on low-level attributes and details, text-to-image diffusion models contain more high-level knowledge thanks to the vision-language pre-training. In this paper, we propose VPD (Visual Perception with a pre-trained Diffusion model), a new framework that exploits the semantic information of a pre-trained text-to-image diffusion model in visual perception tasks. Instead of using the pre-trained denoising autoencoder in a diffusion-based pipeline, we simply use it as a backbone and aim to study how to take full advantage of the learned knowledge. Specifically, we prompt the denoising decoder with proper textual inputs and refine the text features with an adapter, leading to a better alignment to the pre-trained stage and making the visual contents interact with the text prompts. We also propose to utilize the cross-attention maps between the visual features and the text features to provide explicit guidance. Compared with other pre-training methods, we show that vision-language pre-trained diffusion models can be faster adapted to downstream visual perception tasks using the proposed VPD. Extensive experiments on semantic segmentation, referring image segmentation and depth estimation demonstrates the effectiveness of our method. Notably, VPD attains 0.254 RMSE on NYUv2 depth estimation and 73.3% oIoU on RefCOCO-val referring image segmentation, establishing new records on these two benchmarks. Code is available at https://github.com/wl-zhao/VPD
PDF project page: https://vpd.ivg-research.xyz
点此查看论文截图
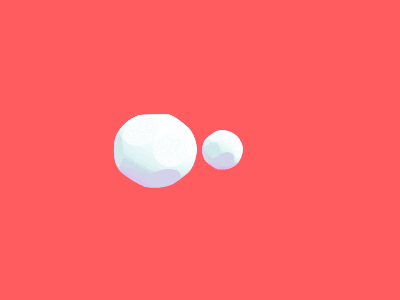
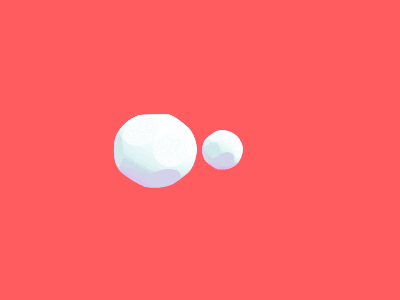
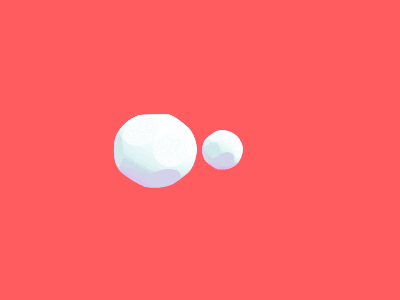