2023-03-04 更新
AIIR-MIX: Multi-Agent Reinforcement Learning Meets Attention Individual Intrinsic Reward Mixing Network
Authors:Wei Li, Weiyan Liu, Shitong Shao, Shiyi Huang
Deducing the contribution of each agent and assigning the corresponding reward to them is a crucial problem in cooperative Multi-Agent Reinforcement Learning (MARL). Previous studies try to resolve the issue through designing an intrinsic reward function, but the intrinsic reward is simply combined with the environment reward by summation in these studies, which makes the performance of their MARL framework unsatisfactory. We propose a novel method named Attention Individual Intrinsic Reward Mixing Network (AIIR-MIX) in MARL, and the contributions of AIIR-MIX are listed as follows:(a) we construct a novel intrinsic reward network based on the attention mechanism to make teamwork more effective. (b) we propose a Mixing network that is able to combine intrinsic and extrinsic rewards non-linearly and dynamically in response to changing conditions of the environment. We compare AIIR-MIX with many State-Of-The-Art (SOTA) MARL methods on battle games in StarCraft II. And the results demonstrate that AIIR-MIX performs admirably and can defeat the current advanced methods on average test win rate. To validate the effectiveness of AIIR-MIX, we conduct additional ablation studies. The results show that AIIR-MIX can dynamically assign each agent a real-time intrinsic reward in accordance with their actual contribution.
PDF
点此查看论文截图
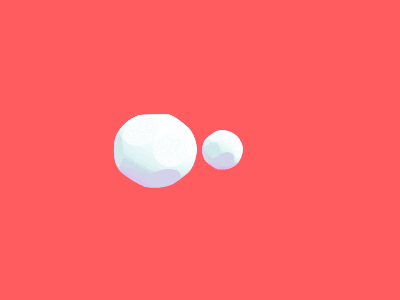
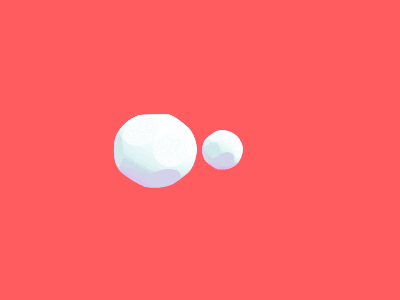
Efficient Communication via Self-supervised Information Aggregation for Online and Offline Multi-agent Reinforcement Learning
Authors:Cong Guan, Feng Chen, Lei Yuan, Zongzhang Zhang, Yang Yu
Utilizing messages from teammates can improve coordination in cooperative Multi-agent Reinforcement Learning (MARL). Previous works typically combine raw messages of teammates with local information as inputs for policy. However, neglecting message aggregation poses significant inefficiency for policy learning. Motivated by recent advances in representation learning, we argue that efficient message aggregation is essential for good coordination in cooperative MARL. In this paper, we propose Multi-Agent communication via Self-supervised Information Aggregation (MASIA), where agents can aggregate the received messages into compact representations with high relevance to augment the local policy. Specifically, we design a permutation invariant message encoder to generate common information-aggregated representation from messages and optimize it via reconstructing and shooting future information in a self-supervised manner. Hence, each agent would utilize the most relevant parts of the aggregated representation for decision-making by a novel message extraction mechanism. Furthermore, considering the potential of offline learning for real-world applications, we build offline benchmarks for multi-agent communication, which is the first as we know. Empirical results demonstrate the superiority of our method in both online and offline settings. We also release the built offline benchmarks in this paper as a testbed for communication ability validation to facilitate further future research.
PDF
点此查看论文截图
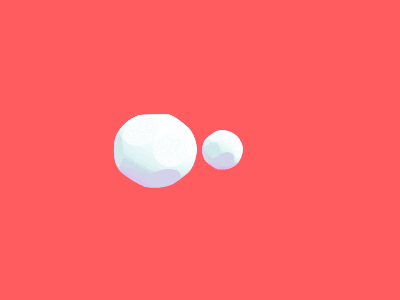
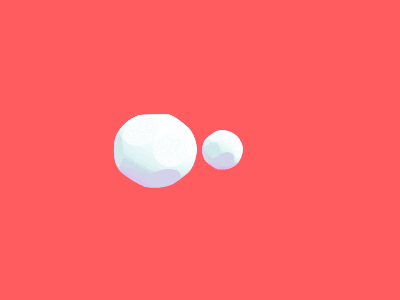
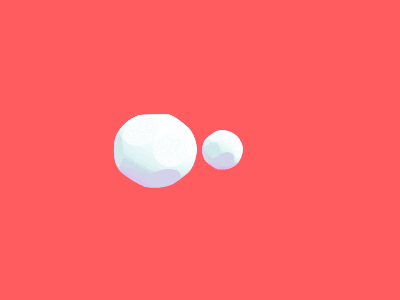