2023-03-04 更新
Few-Shot Point Cloud Semantic Segmentation via Contrastive Self-Supervision and Multi-Resolution Attention
Authors:Jiahui Wang, Haiyue Zhu, Haoren Guo, Abdullah Al Mamun, Cheng Xiang, Tong Heng Lee
This paper presents an effective few-shot point cloud semantic segmentation approach for real-world applications. Existing few-shot segmentation methods on point cloud heavily rely on the fully-supervised pretrain with large annotated datasets, which causes the learned feature extraction bias to those pretrained classes. However, as the purpose of few-shot learning is to handle unknown/unseen classes, such class-specific feature extraction in pretrain is not ideal to generalize into new classes for few-shot learning. Moreover, point cloud datasets hardly have a large number of classes due to the annotation difficulty. To address these issues, we propose a contrastive self-supervision framework for few-shot learning pretrain, which aims to eliminate the feature extraction bias through class-agnostic contrastive supervision. Specifically, we implement a novel contrastive learning approach with a learnable augmentor for a 3D point cloud to achieve point-wise differentiation, so that to enhance the pretrain with managed overfitting through the self-supervision. Furthermore, we develop a multi-resolution attention module using both the nearest and farthest points to extract the local and global point information more effectively, and a center-concentrated multi-prototype is adopted to mitigate the intra-class sparsity. Comprehensive experiments are conducted to evaluate the proposed approach, which shows our approach achieves state-of-the-art performance. Moreover, a case study on practical CAM/CAD segmentation is presented to demonstrate the effectiveness of our approach for real-world applications.
PDF ICRA 2023
点此查看论文截图
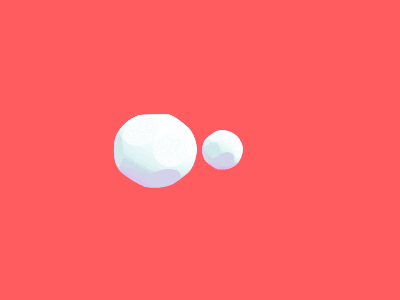
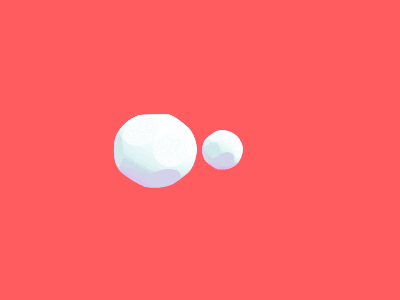
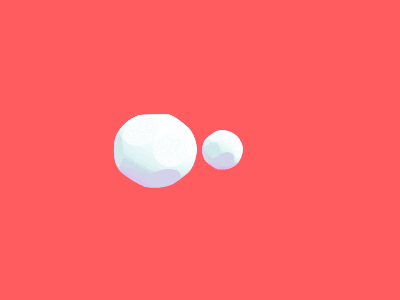
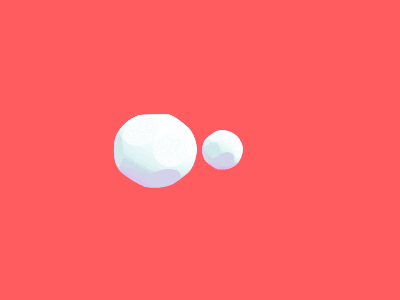
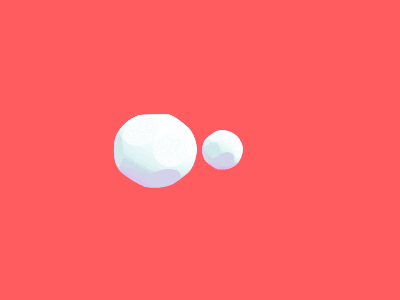
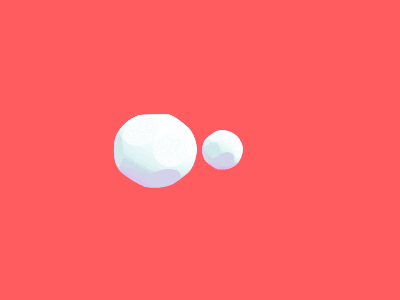
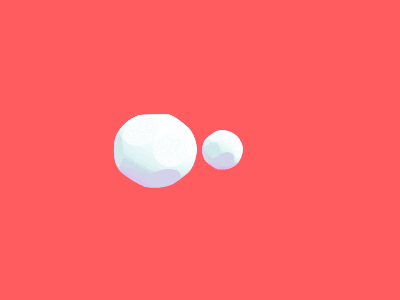
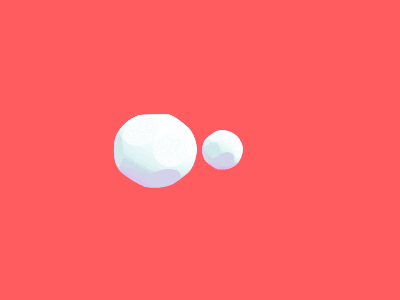
Few-Shot Structured Policy Learning for Multi-Domain and Multi-Task Dialogues
Authors:Thibault Cordier, Tanguy Urvoy, Fabrice Lefevre, Lina M. Rojas-Barahona
Reinforcement learning has been widely adopted to model dialogue managers in task-oriented dialogues. However, the user simulator provided by state-of-the-art dialogue frameworks are only rough approximations of human behaviour. The ability to learn from a small number of human interactions is hence crucial, especially on multi-domain and multi-task environments where the action space is large. We therefore propose to use structured policies to improve sample efficiency when learning on these kinds of environments. We also evaluate the impact of learning from human vs simulated experts. Among the different levels of structure that we tested, the graph neural networks (GNNs) show a remarkable superiority by reaching a success rate above 80% with only 50 dialogues, when learning from simulated experts. They also show superiority when learning from human experts, although a performance drop was observed, indicating a possible difficulty in capturing the variability of human strategies. We therefore suggest to concentrate future research efforts on bridging the gap between human data, simulators and automatic evaluators in dialogue frameworks.
PDF 8 pages, at the EACL2023 conference (Findings)
点此查看论文截图
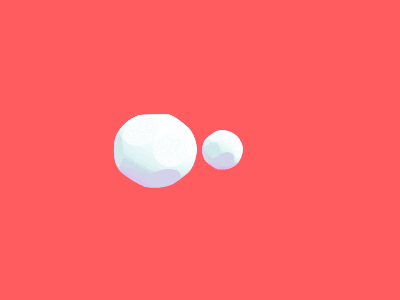
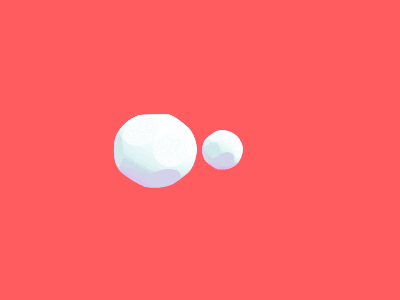
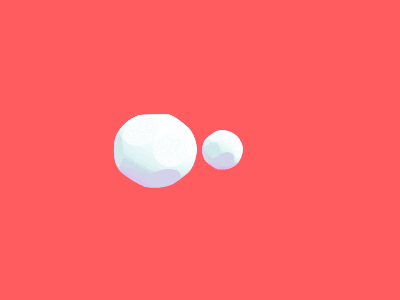
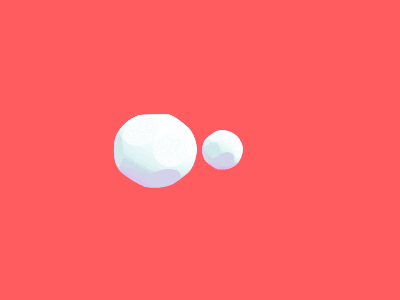
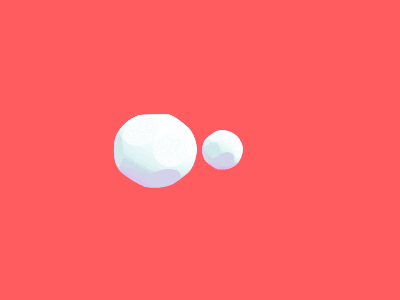
Few-Shot Table-to-Text Generation with Prompt-based Adapter
Authors:Zhixin Guo, Minyxuan Yan, Jiexing Qi, Jianping Zhou, Ziwei He, Zhouhan Lin, Guanjie Zheng, Xinbing Wang
Pre-trained language models (PLMs) have made remarkable progress in table-to-text generation tasks. However, the topological gap between tabular data and text and the lack of domain-specific knowledge make it difficult for PLMs to produce faithful text, especially in real-world applications with limited resources. In this paper, we mitigate the above challenges by introducing a novel augmentation method: Prompt-based Adapter (PA), which targets table-to-text generation under few-shot conditions. The core insight design of the PA is to inject prompt templates for augmenting domain-specific knowledge and table-related representations into the model for bridging the structural gap between tabular data and descriptions through adapters. Such prompt-based knowledge augmentation method brings at least two benefits: (1) enables us to fully use the large amounts of unlabelled domain-specific knowledge, which can alleviate the PLMs’ inherent shortcomings of lacking domain knowledge; (2) allows us to design different types of tasks supporting the generative challenge. Extensive experiments and analyses are conducted on three open-domain few-shot NLG datasets: Humans, Books, and Songs. Compared to previous state-of-the-art approaches, our model achieves superior performance in terms of both fluency and accuracy as judged by human and automatic evaluations.
PDF arXiv admin note: substantial text overlap with arXiv:2302.04415
点此查看论文截图
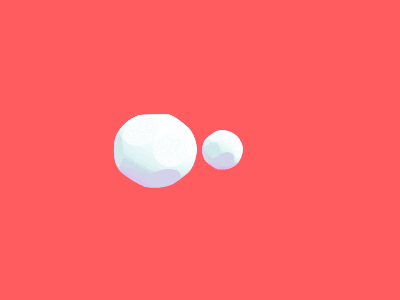
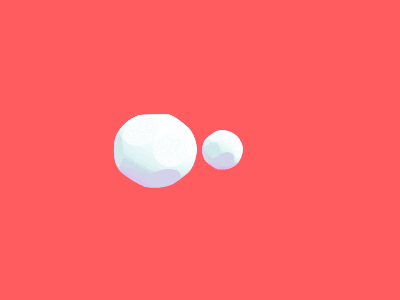
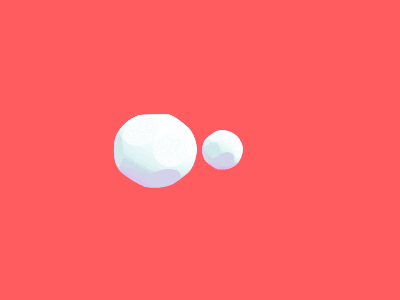
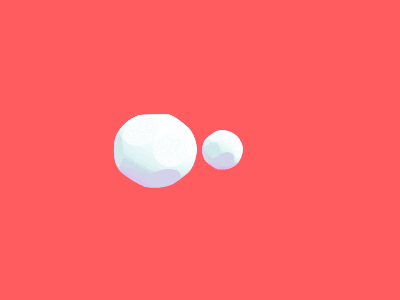
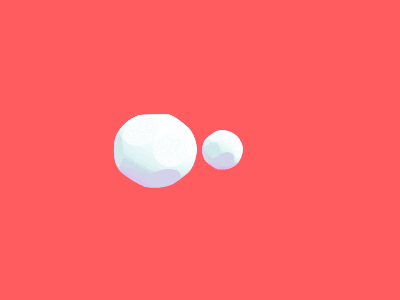
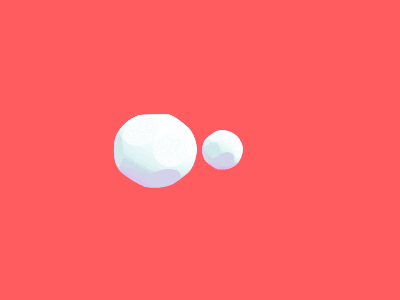
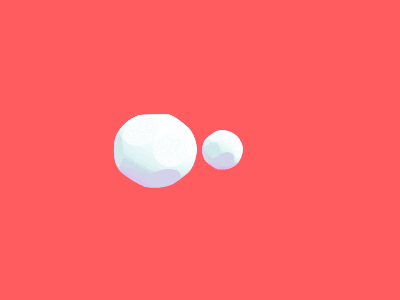
Language Models are Few-shot Learners for Prognostic Prediction
Authors:Zekai Chen, Mariann Micsinai Balan, Kevin Brown
Clinical prediction is an essential task in the healthcare industry. However, the recent success of transformers, on which large language models are built, has not been extended to this domain. In this research, we explore the use of transformers and language models in prognostic prediction for immunotherapy using real-world patients’ clinical data and molecular profiles. This paper investigates the potential of transformers to improve clinical prediction compared to conventional machine learning approaches and addresses the challenge of few-shot learning in predicting rare disease areas. The study benchmarks the efficacy of baselines and language models on prognostic prediction across multiple cancer types and investigates the impact of different pretrained language models under few-shot regimes. The results demonstrate significant improvements in accuracy and highlight the potential of NLP in clinical research to improve early detection and intervention for different diseases. Anonymous codes are available at \url{https://anonymous.4open.science/r/table2text-88ED}.
PDF 7 pages, 5 figures, 5 tables
点此查看论文截图
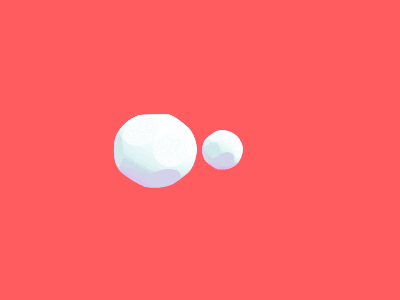
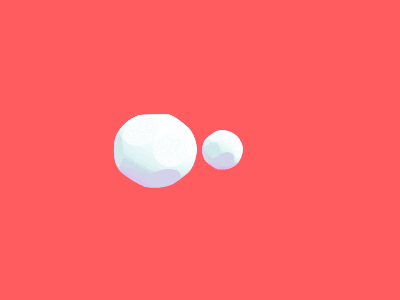
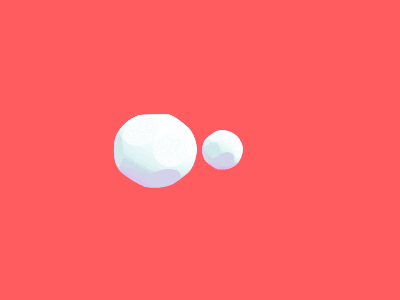
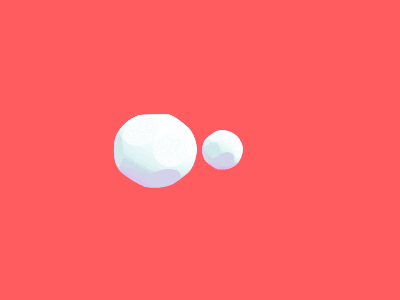
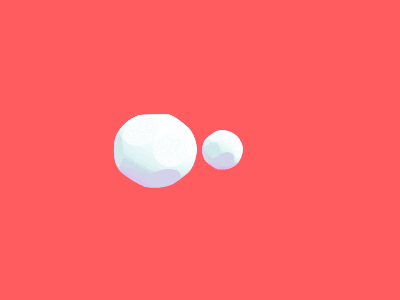
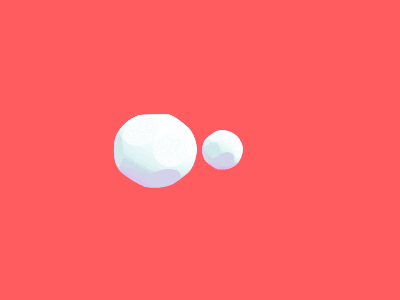
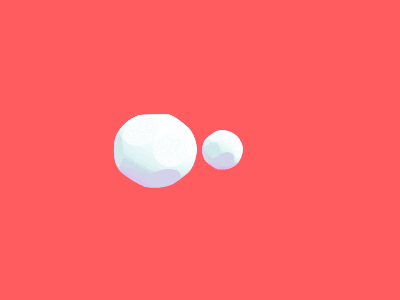
A Prototypical Semantic Decoupling Method via Joint Contrastive Learning for Few-Shot Name Entity Recognition
Authors:Guanting Dong, Zechen Wang, Liwen Wang, Daichi Guo, Dayuan Fu, Yuxiang Wu, Chen Zeng, Xuefeng Li, Tingfeng Hui, Keqing He, Xinyue Cui, Qixiang Gao, Weiran Xu
Few-shot named entity recognition (NER) aims at identifying named entities based on only few labeled instances. Most existing prototype-based sequence labeling models tend to memorize entity mentions which would be easily confused by close prototypes. In this paper, we proposed a Prototypical Semantic Decoupling method via joint Contrastive learning (PSDC) for few-shot NER. Specifically, we decouple class-specific prototypes and contextual semantic prototypes by two masking strategies to lead the model to focus on two different semantic information for inference. Besides, we further introduce joint contrastive learning objectives to better integrate two kinds of decoupling information and prevent semantic collapse. Experimental results on two few-shot NER benchmarks demonstrate that PSDC consistently outperforms the previous SOTA methods in terms of overall performance. Extensive analysis further validates the effectiveness and generalization of PSDC.
PDF 5 pages, 2 figures, published to ICASSP 2023
点此查看论文截图
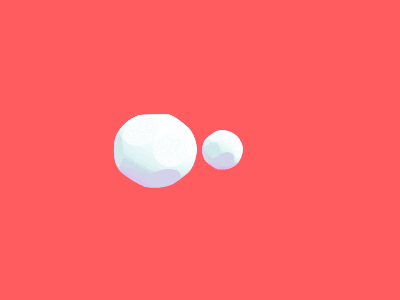
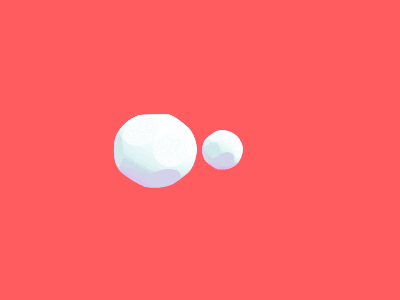
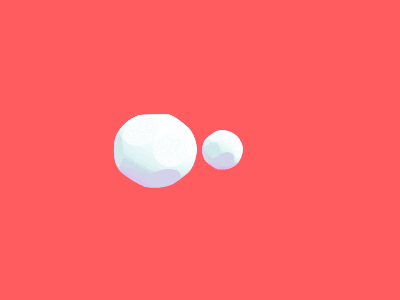
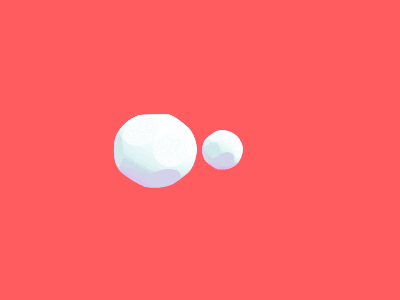
Orca: A Few-shot Benchmark for Chinese Conversational Machine Reading Comprehension
Authors:Nuo Chen, Hongguang Li, Yinan Bao, Junqing He, Xinshi Lin, Qi Yang, Jianfeng Liu, Ruyi Gan, Jiaxing Zhang, Baoyuan Wang, Jia Li
The conversational machine reading comprehension (CMRC) task aims to answer questions in conversations, which has been a hot research topic in recent years because of its wide applications. However, existing CMRC benchmarks in which each conversation is assigned a static passage are inconsistent with real scenarios. Thus, model’s comprehension ability towards real scenarios are hard to evaluate reasonably. To this end, we propose the first Chinese CMRC benchmark Orca and further provide zero-shot/few-shot settings to evaluate model’s generalization ability towards diverse domains. We collect 831 hot-topic driven conversations with 4,742 turns in total. Each turn of a conversation is assigned with a response-related passage, aiming to evaluate model’s comprehension ability more reasonably. The topics of conversations are collected from social media platform and cover 33 domains, trying to be consistent with real scenarios. Importantly, answers in Orca are all well-annotated natural responses rather than the specific spans or short phrase in previous datasets. Besides, we implement three strong baselines to tackle the challenge in Orca. The results indicate the great challenge of our CMRC benchmark. Our datatset and checkpoints are available at https://github.com/nuochenpku/Orca.
PDF 14 pages
点此查看论文截图
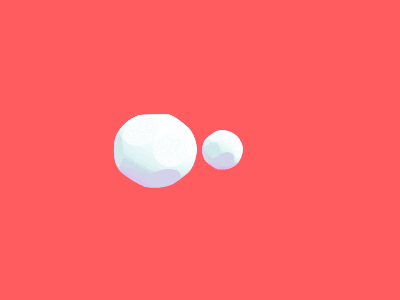
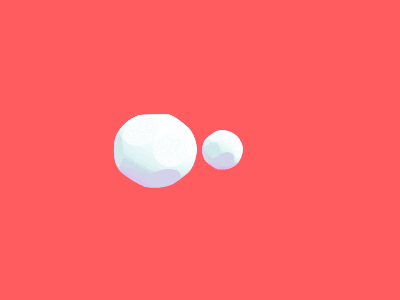
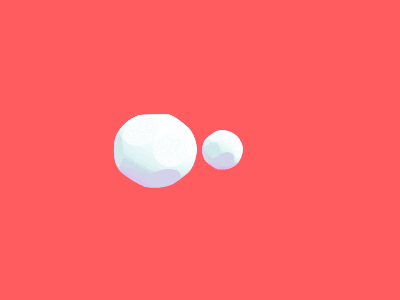
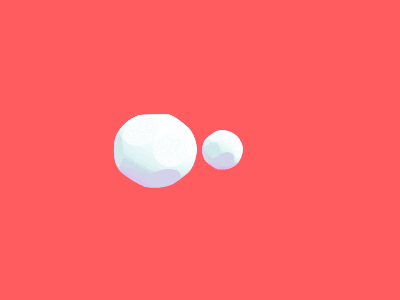
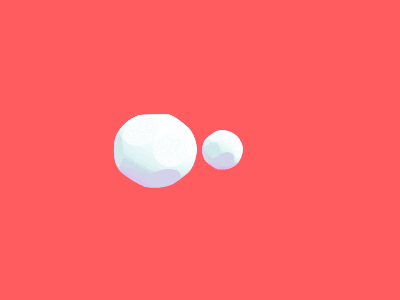
Layer Grafted Pre-training: Bridging Contrastive Learning And Masked Image Modeling For Label-Efficient Representations
Authors:Ziyu Jiang, Yinpeng Chen, Mengchen Liu, Dongdong Chen, Xiyang Dai, Lu Yuan, Zicheng Liu, Zhangyang Wang
Recently, both Contrastive Learning (CL) and Mask Image Modeling (MIM) demonstrate that self-supervision is powerful to learn good representations. However, naively combining them is far from success. In this paper, we start by making the empirical observation that a naive joint optimization of CL and MIM losses leads to conflicting gradient directions - more severe as the layers go deeper. This motivates us to shift the paradigm from combining loss at the end, to choosing the proper learning method per network layer. Inspired by experimental observations, we find that MIM and CL are suitable to lower and higher layers, respectively. We hence propose to combine them in a surprisingly simple, “sequential cascade” fashion: early layers are first trained under one MIM loss, on top of which latter layers continue to be trained under another CL loss. The proposed Layer Grafted Pre-training learns good visual representations that demonstrate superior label efficiency in downstream applications, in particular yielding strong few-shot performance besides linear evaluation. For instance, on ImageNet-1k, Layer Grafted Pre-training yields 65.5% Top-1 accuracy in terms of 1% few-shot learning with ViT-B/16, which improves MIM and CL baselines by 14.4% and 2.1% with no bells and whistles. The code is available at https://github.com/VITA-Group/layerGraftedPretraining_ICLR23.git.
PDF Accepted by ICLR 2023
点此查看论文截图
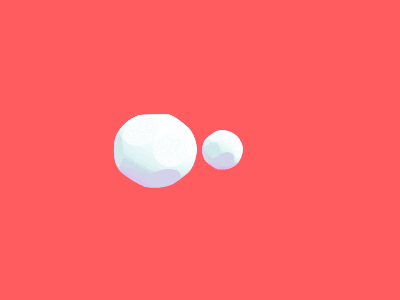
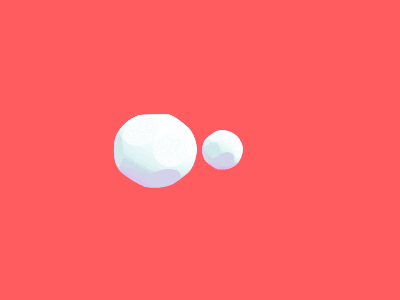
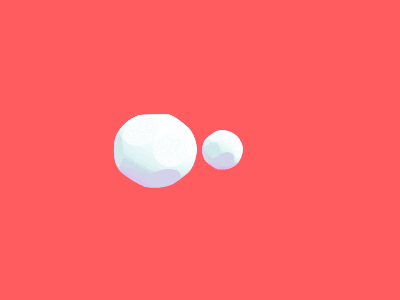
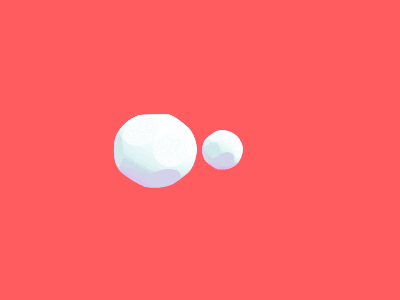
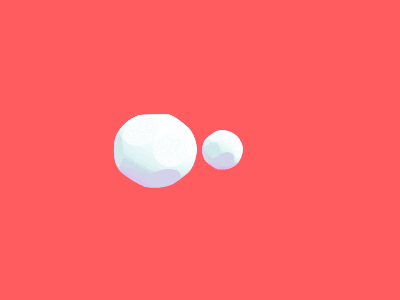
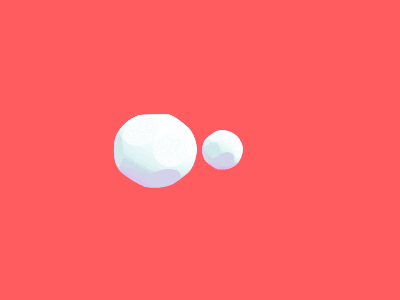
CLR-GAM: Contrastive Point Cloud Learning with Guided Augmentation and Feature Mapping
Authors:Srikanth Malla, Yi-Ting Chen
Point cloud data plays an essential role in robotics and self-driving applications. Yet, annotating point cloud data is time-consuming and nontrivial while they enable learning discriminative 3D representations that empower downstream tasks, such as classification and segmentation. Recently, contrastive learning-based frameworks have shown promising results for learning 3D representations in a self-supervised manner. However, existing contrastive learning methods cannot precisely encode and associate structural features and search the higher dimensional augmentation space efficiently. In this paper, we present CLR-GAM, a novel contrastive learning-based framework with Guided Augmentation (GA) for efficient dynamic exploration strategy and Guided Feature Mapping (GFM) for similar structural feature association between augmented point clouds. We empirically demonstrate that the proposed approach achieves state-of-the-art performance on both simulated and real-world 3D point cloud datasets for three different downstream tasks, i.e., 3D point cloud classification, few-shot learning, and object part segmentation.
PDF
点此查看论文截图
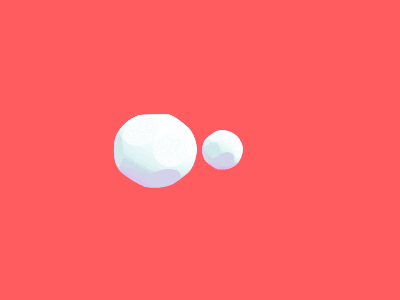
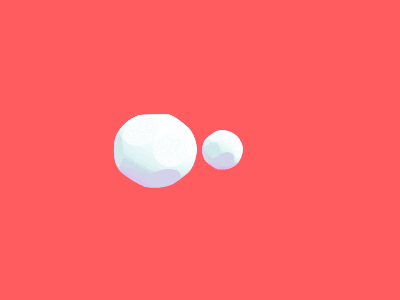
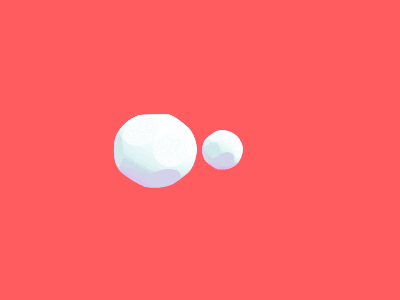
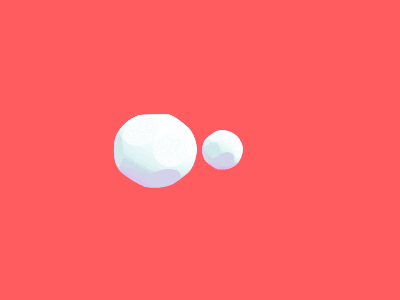
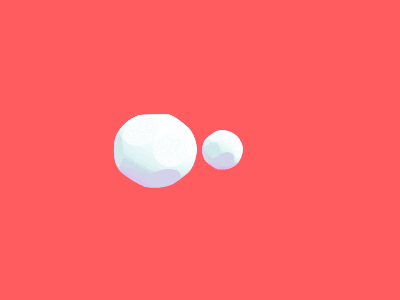
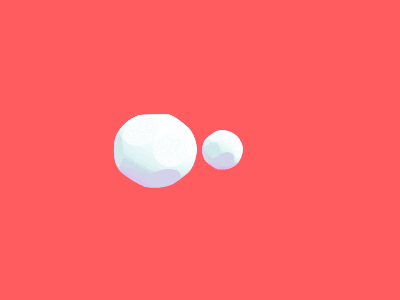
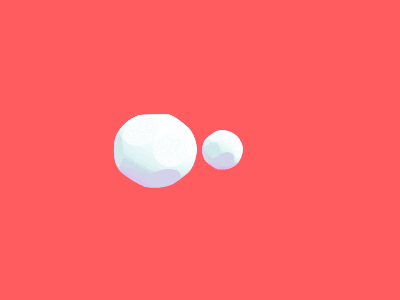
Turning a CLIP Model into a Scene Text Detector
Authors:Wenwen Yu, Yuliang Liu, Wei Hua, Deqiang Jiang, Bo Ren, Xiang Bai
The recent large-scale Contrastive Language-Image Pretraining (CLIP) model has shown great potential in various downstream tasks via leveraging the pretrained vision and language knowledge. Scene text, which contains rich textual and visual information, has an inherent connection with a model like CLIP. Recently, pretraining approaches based on vision language models have made effective progresses in the field of text detection. In contrast to these works, this paper proposes a new method, termed TCM, focusing on Turning the CLIP Model directly for text detection without pretraining process. We demonstrate the advantages of the proposed TCM as follows: (1) The underlying principle of our framework can be applied to improve existing scene text detector. (2) It facilitates the few-shot training capability of existing methods, e.g., by using 10% of labeled data, we significantly improve the performance of the baseline method with an average of 22% in terms of the F-measure on 4 benchmarks. (3) By turning the CLIP model into existing scene text detection methods, we further achieve promising domain adaptation ability. The code will be publicly released at https://github.com/wenwenyu/TCM.
PDF CVPR2023
点此查看论文截图
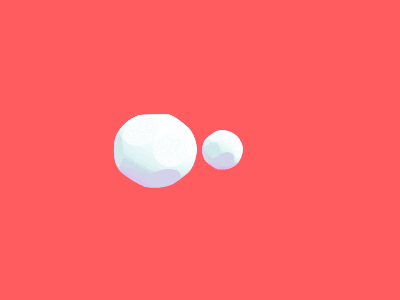
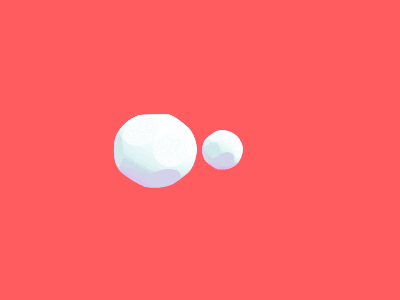
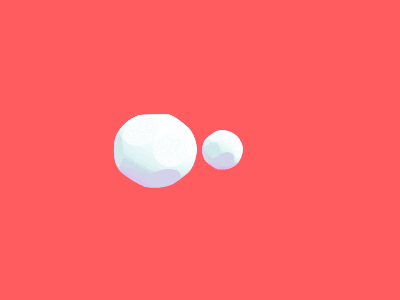
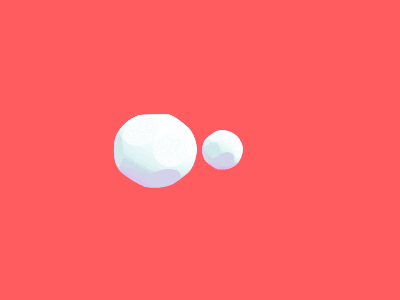
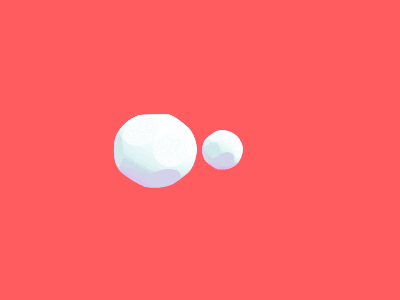
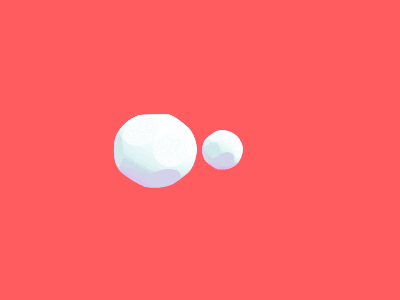
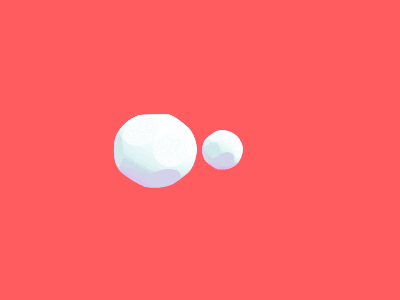
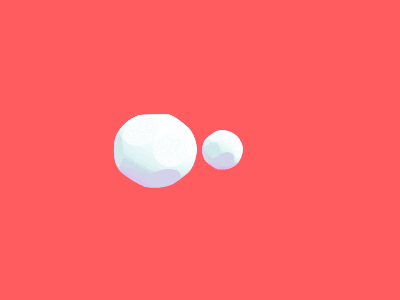
Meta Learning to Bridge Vision and Language Models for Multimodal Few-Shot Learning
Authors:Ivona Najdenkoska, Xiantong Zhen, Marcel Worring
Multimodal few-shot learning is challenging due to the large domain gap between vision and language modalities. Existing methods are trying to communicate visual concepts as prompts to frozen language models, but rely on hand-engineered task induction to reduce the hypothesis space. To make the whole process learnable, we introduce a multimodal meta-learning approach. Specifically, our approach decomposes the training of the model into a set of related multimodal few-shot tasks. We define a meta-mapper network, acting as a meta-learner, to efficiently bridge frozen large-scale vision and language models and leverage their already learned capacity. By updating the learnable parameters only of the meta-mapper, it learns to accrue shared meta-knowledge among these tasks. Thus, it can rapidly adapt to newly presented samples with only a few gradient updates. Importantly, it induces the task in a completely data-driven manner, with no need for a hand-engineered task induction. We evaluate our approach on recently proposed multimodal few-shot benchmarks, measuring how rapidly the model can bind novel visual concepts to words and answer visual questions by observing only a limited set of labeled examples. The experimental results show that our meta-learning approach outperforms the baseline across multiple datasets and various training settings while being computationally more efficient.
PDF International Conference on Learning Representations 2023
点此查看论文截图
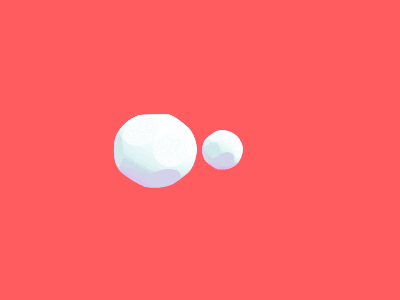
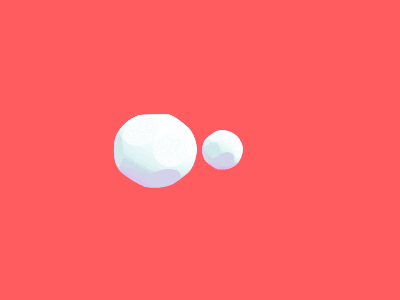
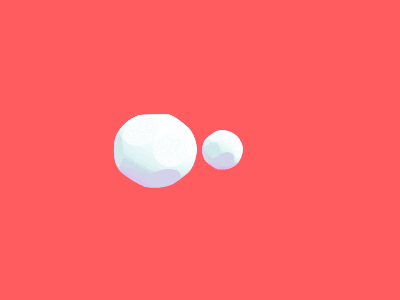
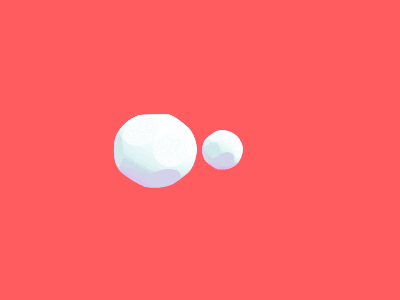
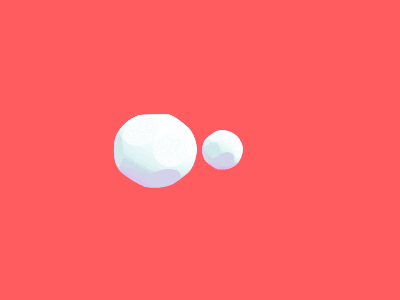
Few-shots Portrait Generation with Style Enhancement and Identity Preservation
Authors:Runchuan Zhu, Naye Ji, Youbing Zhao, Fan Zhang
Nowadays, the wide application of virtual digital human promotes the comprehensive prosperity and development of digital culture supported by digital economy. The personalized portrait automatically generated by AI technology needs both the natural artistic style and human sentiment. In this paper, we propose a novel StyleIdentityGAN model, which can ensure the identity and artistry of the generated portrait at the same time. Specifically, the style-enhanced module focuses on artistic style features decoupling and transferring to improve the artistry of generated virtual face images. Meanwhile, the identity-enhanced module preserves the significant features extracted from the input photo. Furthermore, the proposed method requires a small number of reference style data. Experiments demonstrate the superiority of StyleIdentityGAN over state-of-art methods in artistry and identity effects, with comparisons done qualitatively, quantitatively and through a perceptual user study. Code has been released on Github3.
PDF
点此查看论文截图
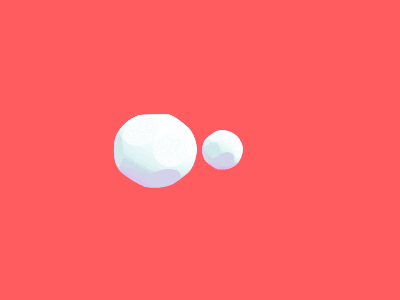
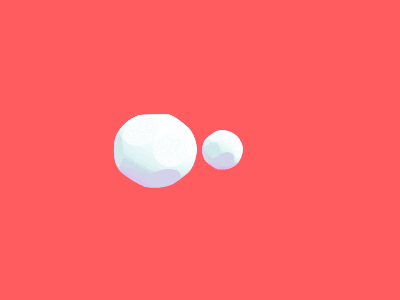
STUNT: Few-shot Tabular Learning with Self-generated Tasks from Unlabeled Tables
Authors:Jaehyun Nam, Jihoon Tack, Kyungmin Lee, Hankook Lee, Jinwoo Shin
Learning with few labeled tabular samples is often an essential requirement for industrial machine learning applications as varieties of tabular data suffer from high annotation costs or have difficulties in collecting new samples for novel tasks. Despite the utter importance, such a problem is quite under-explored in the field of tabular learning, and existing few-shot learning schemes from other domains are not straightforward to apply, mainly due to the heterogeneous characteristics of tabular data. In this paper, we propose a simple yet effective framework for few-shot semi-supervised tabular learning, coined Self-generated Tasks from UNlabeled Tables (STUNT). Our key idea is to self-generate diverse few-shot tasks by treating randomly chosen columns as a target label. We then employ a meta-learning scheme to learn generalizable knowledge with the constructed tasks. Moreover, we introduce an unsupervised validation scheme for hyperparameter search (and early stopping) by generating a pseudo-validation set using STUNT from unlabeled data. Our experimental results demonstrate that our simple framework brings significant performance gain under various tabular few-shot learning benchmarks, compared to prior semi- and self-supervised baselines. Code is available at https://github.com/jaehyun513/STUNT.
PDF ICLR 2023 (Spotlight)
点此查看论文截图
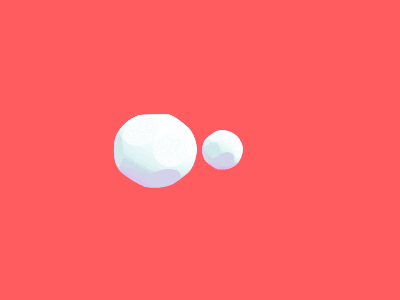
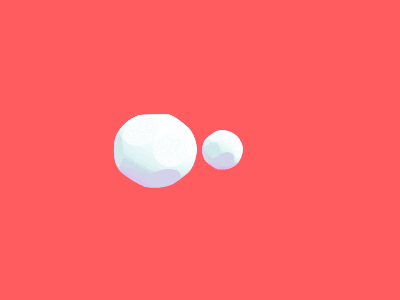
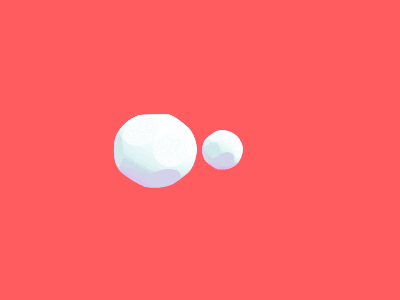
Practical Network Acceleration with Tiny Sets: Hypothesis, Theory, and Algorithm
Authors:Guo-Hua Wang, Jianxin Wu
Due to data privacy issues, accelerating networks with tiny training sets has become a critical need in practice. Previous methods achieved promising results empirically by filter-level pruning. In this paper, we both study this problem theoretically and propose an effective algorithm aligning well with our theoretical results. First, we propose the finetune convexity hypothesis to explain why recent few-shot compression algorithms do not suffer from overfitting problems. Based on it, a theory is further established to explain these methods for the first time. Compared to naively finetuning a pruned network, feature mimicking is proved to achieve a lower variance of parameters and hence enjoys easier optimization. With our theoretical conclusions, we claim dropping blocks is a fundamentally superior few-shot compression scheme in terms of more convex optimization and a higher acceleration ratio. To choose which blocks to drop, we propose a new metric, recoverability, to effectively measure the difficulty of recovering the compressed network. Finally, we propose an algorithm named PRACTISE to accelerate networks using only tiny training sets. PRACTISE outperforms previous methods by a significant margin. For 22% latency reduction, it surpasses previous methods by on average 7 percentage points on ImageNet-1k. It also works well under data-free or out-of-domain data settings. Our code is at https://github.com/DoctorKey/Practise
PDF under review for TPAMI
点此查看论文截图
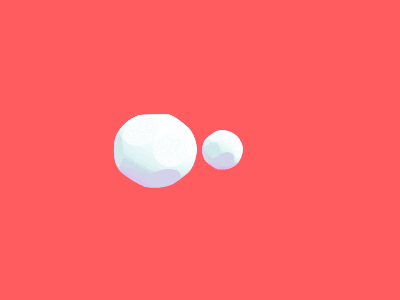
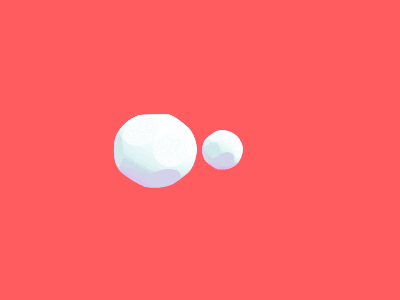
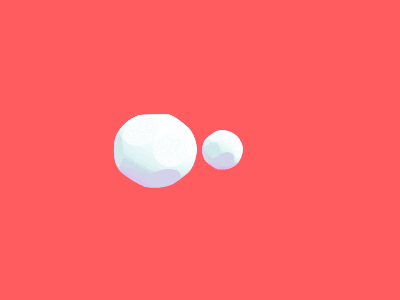
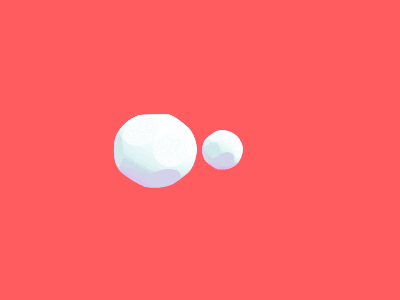
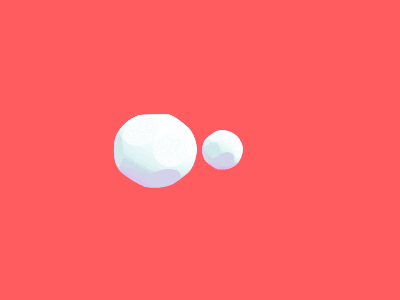
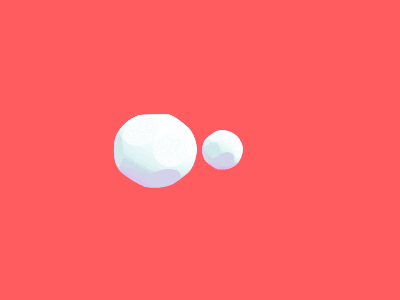
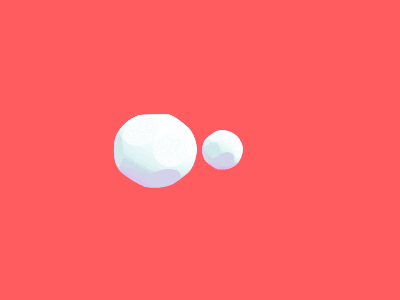
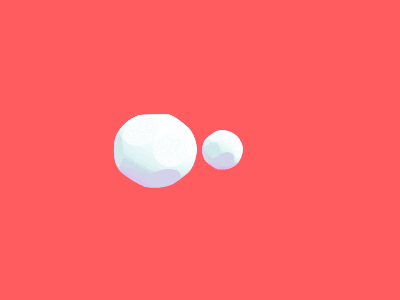
Unsupervised Meta-Learning via Few-shot Pseudo-supervised Contrastive Learning
Authors:Huiwon Jang, Hankook Lee, Jinwoo Shin
Unsupervised meta-learning aims to learn generalizable knowledge across a distribution of tasks constructed from unlabeled data. Here, the main challenge is how to construct diverse tasks for meta-learning without label information; recent works have proposed to create, e.g., pseudo-labeling via pretrained representations or creating synthetic samples via generative models. However, such a task construction strategy is fundamentally limited due to heavy reliance on the immutable pseudo-labels during meta-learning and the quality of the representations or the generated samples. To overcome the limitations, we propose a simple yet effective unsupervised meta-learning framework, coined Pseudo-supervised Contrast (PsCo), for few-shot classification. We are inspired by the recent self-supervised learning literature; PsCo utilizes a momentum network and a queue of previous batches to improve pseudo-labeling and construct diverse tasks in a progressive manner. Our extensive experiments demonstrate that PsCo outperforms existing unsupervised meta-learning methods under various in-domain and cross-domain few-shot classification benchmarks. We also validate that PsCo is easily scalable to a large-scale benchmark, while recent prior-art meta-schemes are not.
PDF Accepted to ICLR 2023 (Spotlight). The first two authors contributed equally. The code is available at https://github.com/alinlab/PsCo
点此查看论文截图
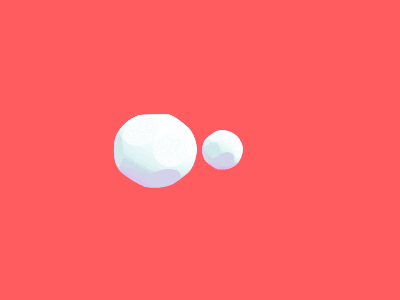
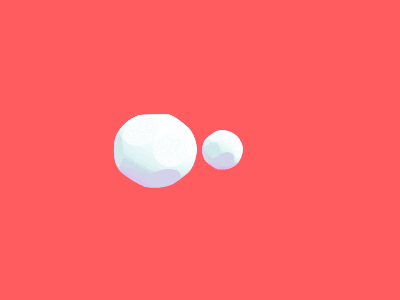
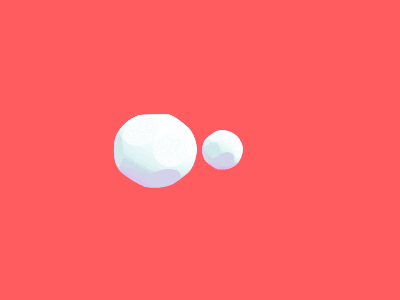
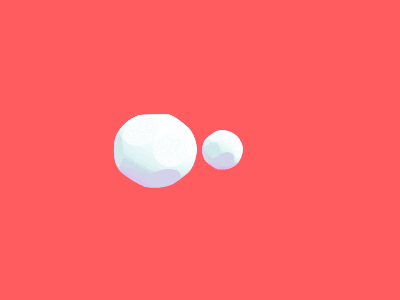
Model agnostic methods meta-learn despite misspecifications
Authors:Oguz Yuksel, Etienne Boursier, Nicolas Flammarion
Due to its empirical success on few shot classification and reinforcement learning, meta-learning recently received a lot of interest. Meta-learning leverages data from previous tasks to quickly learn a new task, despite limited data. In particular, model agnostic methods look for initialisation points from which gradient descent quickly adapts to any new task. Although it has been empirically suggested that such methods learn a good shared representation during training, there is no strong theoretical evidence of such behavior. More importantly, it is unclear whether these methods truly are model agnostic, i.e., whether they still learn a shared structure despite architecture misspecifications. To fill this gap, this work shows in the limit of an infinite number of tasks that first order ANIL with a linear two-layer network architecture successfully learns a linear shared representation. Moreover, this result holds despite misspecifications: having a large width with respect to the hidden dimension of the shared representation does not harm the algorithm performance. The learnt parameters then allow to get a small test loss after a single gradient step on any new task. Overall this illustrates how well model agnostic methods can adapt to any (unknown) model structure.
PDF
点此查看论文截图
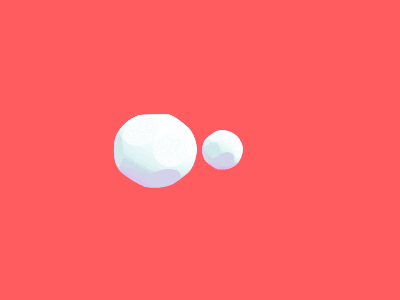
Matching-based Term Semantics Pre-training for Spoken Patient Query Understanding
Authors:Zefa Hu, Xiuyi Chen, Haoran Wu, Minglun Han, Ziyi Ni, Jing Shi, Shuang Xu, Bo Xu
Medical Slot Filling (MSF) task aims to convert medical queries into structured information, playing an essential role in diagnosis dialogue systems. However, the lack of sufficient term semantics learning makes existing approaches hard to capture semantically identical but colloquial expressions of terms in medical conversations. In this work, we formalize MSF into a matching problem and propose a Term Semantics Pre-trained Matching Network (TSPMN) that takes both terms and queries as input to model their semantic interaction. To learn term semantics better, we further design two self-supervised objectives, including Contrastive Term Discrimination (CTD) and Matching-based Mask Term Modeling (MMTM). CTD determines whether it is the masked term in the dialogue for each given term, while MMTM directly predicts the masked ones. Experimental results on two Chinese benchmarks show that TSPMN outperforms strong baselines, especially in few-shot settings.
PDF ICASSP 2023
点此查看论文截图
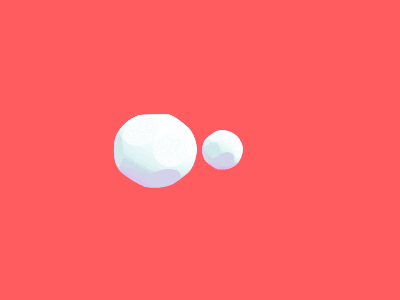
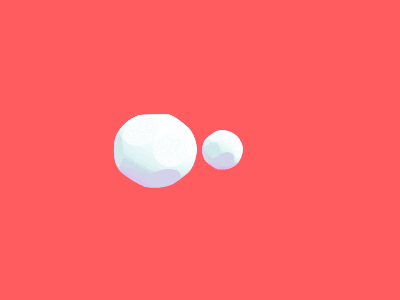
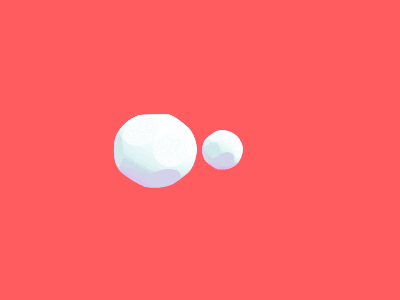
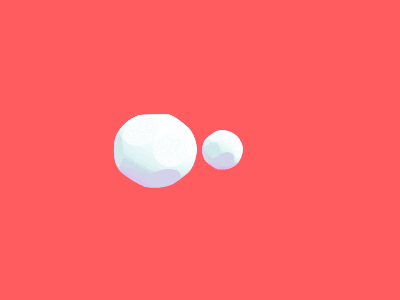
Human Motion Diffusion as a Generative Prior
Authors:Yonatan Shafir, Guy Tevet, Roy Kapon, Amit H. Bermano
In recent months, we witness a leap forward as denoising diffusion models were introduced to Motion Generation. Yet, the main gap in this field remains the low availability of data. Furthermore, the expensive acquisition process of motion biases the already modest data towards short single-person sequences. With such a shortage, more elaborate generative tasks are left behind. In this paper, we show that this gap can be mitigated using a pre-trained diffusion-based model as a generative prior. We demonstrate the prior is effective for fine-tuning, in a few-, and even a zero-shot manner. For the zero-shot setting, we tackle the challenge of long sequence generation. We introduce DoubleTake, an inference-time method with which we demonstrate up to 10-minute long animations of prompted intervals and their meaningful and controlled transition, using the prior that was trained for 10-second generations. For the few-shot setting, we consider two-person generation. Using two fixed priors and as few as a dozen training examples, we learn a slim communication block, ComMDM, to infuse interaction between the two resulting motions. Finally, using fine-tuning, we train the prior to semantically complete motions from a single prescribed joint. Then, we use our DiffusionBlending to blend a few such models into a single one that responds well to the combination of the individual control signals, enabling fine-grained joint- and trajectory-level control and editing. Using an off-the-shelf state-of-the-art (SOTA) motion diffusion model as a prior, we evaluate our approach for the three mentioned cases and show that we consistently outperform SOTA models that were designed and trained for those tasks.
PDF
点此查看论文截图
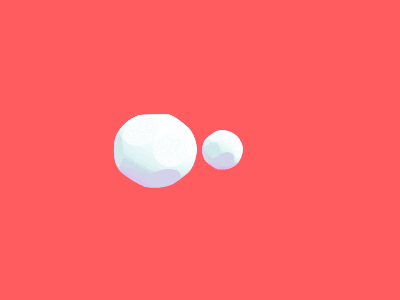
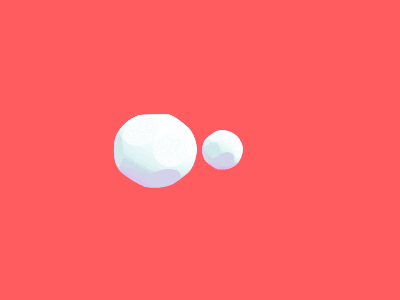
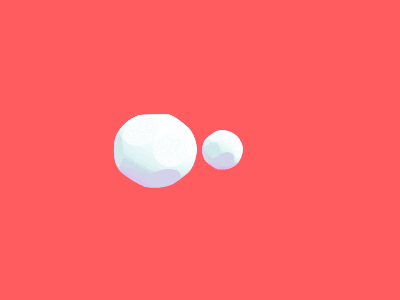
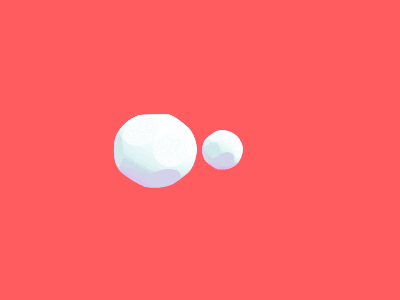
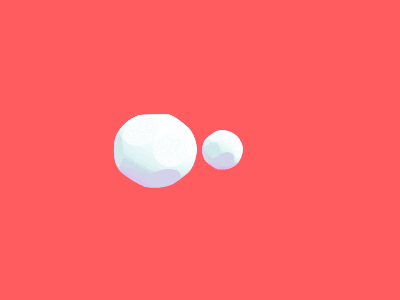
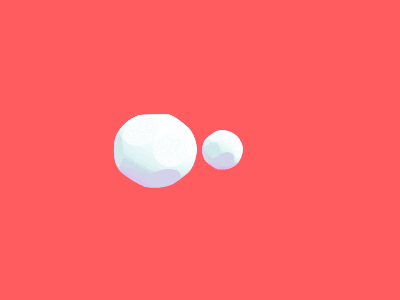
Consistency Models
Authors:Yang Song, Prafulla Dhariwal, Mark Chen, Ilya Sutskever
Diffusion models have made significant breakthroughs in image, audio, and video generation, but they depend on an iterative generation process that causes slow sampling speed and caps their potential for real-time applications. To overcome this limitation, we propose consistency models, a new family of generative models that achieve high sample quality without adversarial training. They support fast one-step generation by design, while still allowing for few-step sampling to trade compute for sample quality. They also support zero-shot data editing, like image inpainting, colorization, and super-resolution, without requiring explicit training on these tasks. Consistency models can be trained either as a way to distill pre-trained diffusion models, or as standalone generative models. Through extensive experiments, we demonstrate that they outperform existing distillation techniques for diffusion models in one- and few-step generation. For example, we achieve the new state-of-the-art FID of 3.55 on CIFAR-10 and 6.20 on ImageNet 64x64 for one-step generation. When trained as standalone generative models, consistency models also outperform single-step, non-adversarial generative models on standard benchmarks like CIFAR-10, ImageNet 64x64 and LSUN 256x256.
PDF
点此查看论文截图
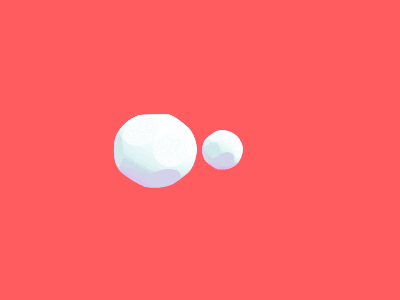
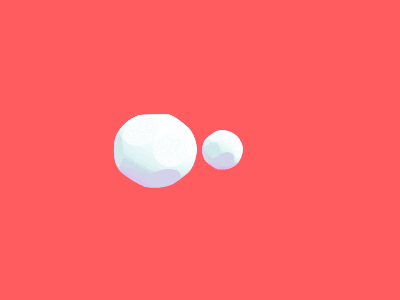
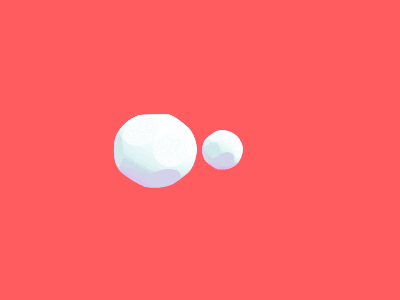
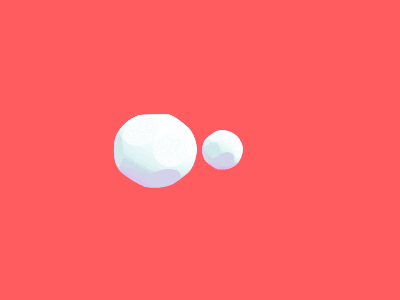