2022-12-09 更新
Generating and Weighting Semantically Consistent Sample Pairs for Ultrasound Contrastive Learning
Authors:Yixiong Chen, Chunhui Zhang, Chris H. Q. Ding, Li Liu
Well-annotated medical datasets enable deep neural networks (DNNs) to gain strong power in extracting lesion-related features. Building such large and well-designed medical datasets is costly due to the need for high-level expertise. Model pre-training based on ImageNet is a common practice to gain better generalization when the data amount is limited. However, it suffers from the domain gap between natural and medical images. In this work, we pre-train DNNs on ultrasound (US) domains instead of ImageNet to reduce the domain gap in medical US applications. To learn US image representations based on unlabeled US videos, we propose a novel meta-learning-based contrastive learning method, namely Meta Ultrasound Contrastive Learning (Meta-USCL). To tackle the key challenge of obtaining semantically consistent sample pairs for contrastive learning, we present a positive pair generation module along with an automatic sample weighting module based on meta-learning. Experimental results on multiple computer-aided diagnosis (CAD) problems, including pneumonia detection, breast cancer classification, and breast tumor segmentation, show that the proposed self-supervised method reaches state-of-the-art (SOTA). The codes are available at https://github.com/Schuture/Meta-USCL.
PDF
点此查看论文截图
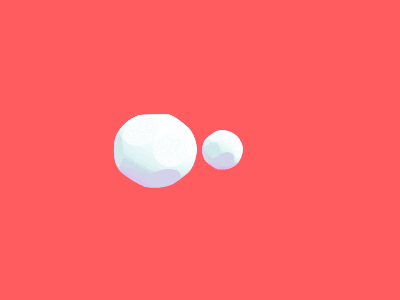
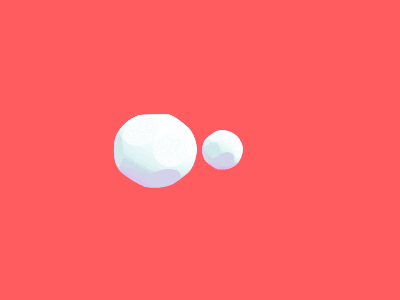
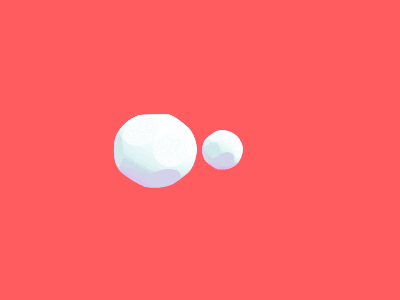
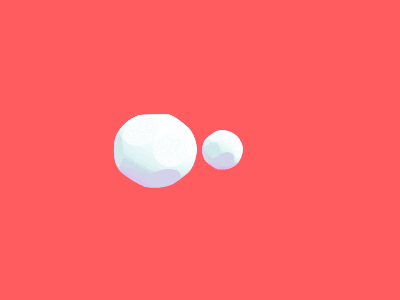
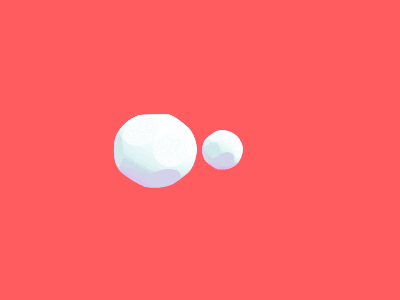