2022-12-08 更新
PointCaM: Cut-and-Mix for Open-Set Point Cloud Analysis
Authors:Jie Hong, Shi Qiu, Weihao Li, Saeed Anwar, Mehrtash Harandi, Nick Barnes, Lars Petersson
Point cloud analysis is receiving increasing attention, however, most existing point cloud models lack the practical ability to deal with the unavoidable presence of unknown objects. This paper mainly discusses point cloud analysis under open-set settings, where we train the model without data from unknown classes and identify them in the inference stage. Basically, we propose to solve open-set point cloud analysis using a novel Point Cut-and-Mix mechanism consisting of Unknown-Point Simulator and Unknown-Point Estimator modules. Specifically, we use the Unknown-Point Simulator to simulate unknown data in the training stage by manipulating the geometric context of partial known data. Based on this, the Unknown-Point Estimator module learns to exploit the point cloud’s feature context for discriminating the known and unknown data. Extensive experiments show the plausibility of open-set point cloud analysis and the effectiveness of our proposed solutions. Our code is available at \url{https://github.com/ShiQiu0419/pointcam}.
PDF
点此查看论文截图
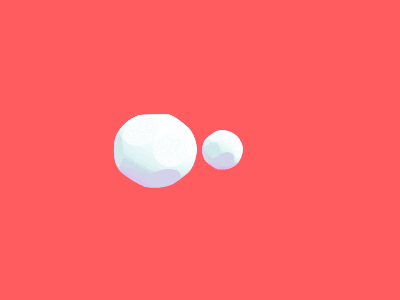
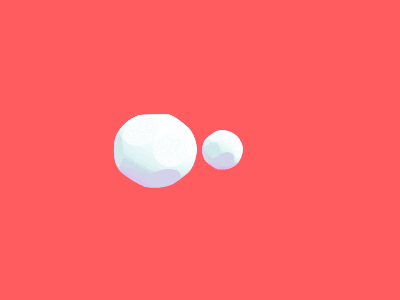
Open Set Recognition For Music Genre Classification
Authors:Kevin Liu, Julien DeMori, Kobi Abayomi
We explore segmentation of known and unknown genre classes using the open source GTZAN and FMA datasets. For each, we begin with best-case closed set genre classification, then we apply open set recognition methods. We offer an algorithm for the music genre classification task using OSR. We demonstrate the ability to retrieve known genres and as well identification of aural patterns for novel genres (not appearing in a training set). We conduct four experiments, each containing a different set of known and unknown classes, using the GTZAN and the FMA datasets to establish a baseline capacity for novel genre detection. We employ grid search on both OpenMax and softmax to determine the optimal total classification accuracy for each experimental setup, and illustrate interaction between genre labelling and open set recognition accuracy.
PDF 9 pages, 5 figures, 4 tables
点此查看论文截图
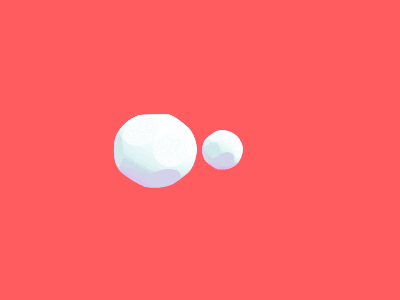
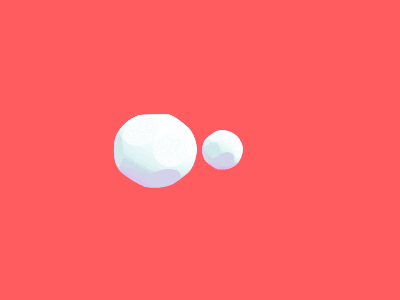
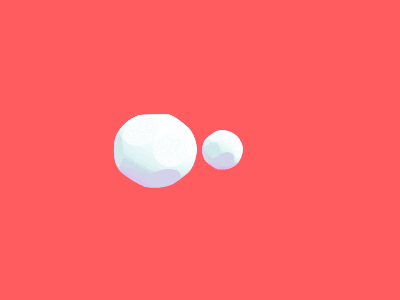
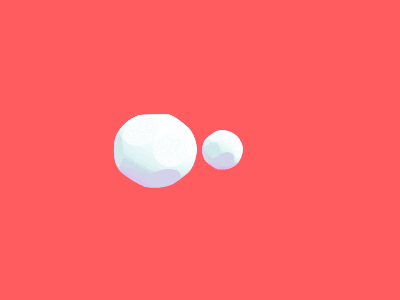
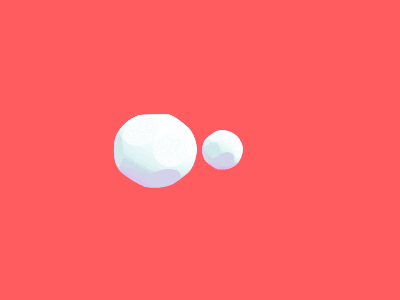
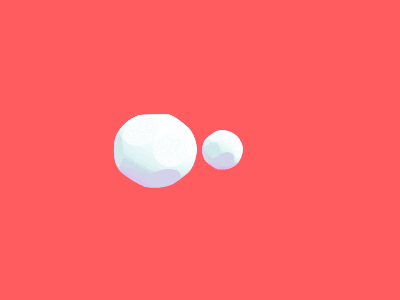
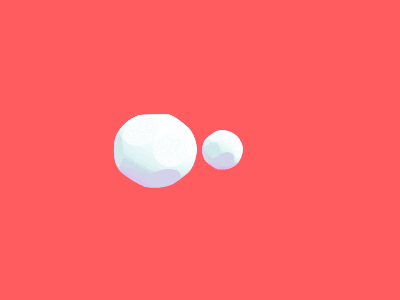
Prompt-driven efficient Open-set Semi-supervised Learning
Authors:Haoran Li, Chun-Mei Feng, Tao Zhou, Yong Xu, Xiaojun Chang
Open-set semi-supervised learning (OSSL) has attracted growing interest, which investigates a more practical scenario where out-of-distribution (OOD) samples are only contained in unlabeled data. Existing OSSL methods like OpenMatch learn an OOD detector to identify outliers, which often update all modal parameters (i.e., full fine-tuning) to propagate class information from labeled data to unlabeled ones. Currently, prompt learning has been developed to bridge gaps between pre-training and fine-tuning, which shows higher computational efficiency in several downstream tasks. In this paper, we propose a prompt-driven efficient OSSL framework, called OpenPrompt, which can propagate class information from labeled to unlabeled data with only a small number of trainable parameters. We propose a prompt-driven joint space learning mechanism to detect OOD data by maximizing the distribution gap between ID and OOD samples in unlabeled data, thereby our method enables the outliers to be detected in a new way. The experimental results on three public datasets show that OpenPrompt outperforms state-of-the-art methods with less than 1% of trainable parameters. More importantly, OpenPrompt achieves a 4% improvement in terms of AUROC on outlier detection over a fully supervised model on CIFAR10.
PDF
点此查看论文截图
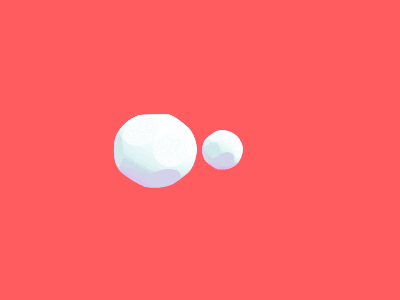
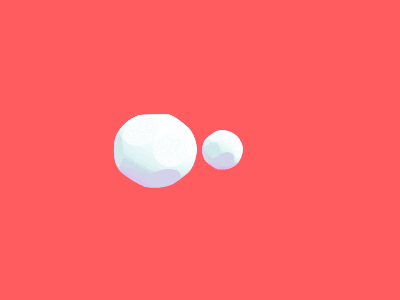
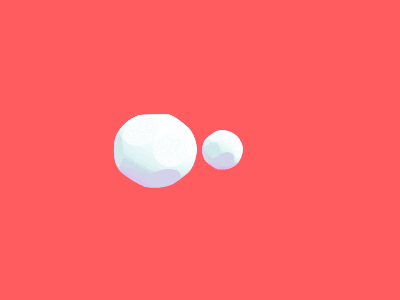
Measuring Human Perception to Improve Open Set Recognition
Authors:Jin Huang, Derek Prijatelj, Justin Dulay, Walter Scheirer
The human ability to recognize when an object is known or novel currently outperforms all open set recognition algorithms. Human perception as measured by the methods and procedures of visual psychophysics from psychology can provide an additional data stream for managing novelty in visual recognition tasks in computer vision. For instance, measured reaction time from human subjects can offer insight as to whether a known class sample may be confused with a novel one. In this work, we designed and performed a large-scale behavioral experiment that collected over 200,000 human reaction time measurements associated with object recognition. The data collected indicated reaction time varies meaningfully across objects at the sample level. We therefore designed a new psychophysical loss function that enforces consistency with human behavior in deep networks which exhibit variable reaction time for different images. As in biological vision, this approach allows us to achieve good open set recognition performance in regimes with limited labeled training data. Through experiments using data from ImageNet, significant improvement is observed when training Multi-Scale DenseNets with this new formulation: models trained with our loss function significantly improved top-1 validation accuracy by 7%, top-1 test accuracy on known samples by 18%, and top-1 test accuracy on unknown samples by 33%. We compared our method to 10 open set recognition methods from the literature, which were all outperformed on multiple metrics.
PDF
点此查看论文截图
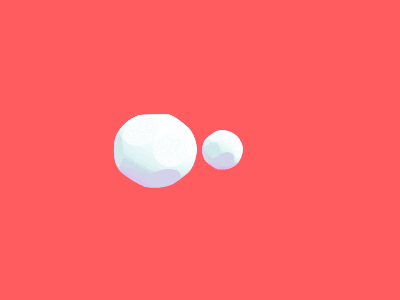
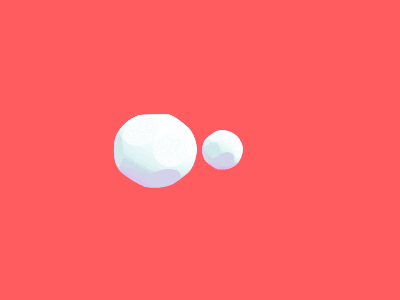
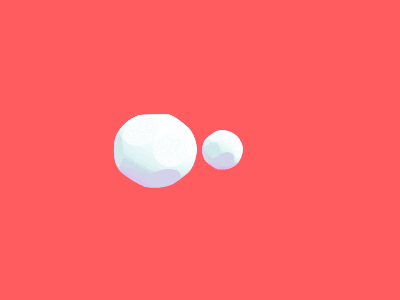
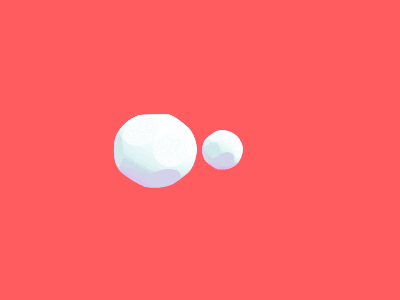