2022-12-01 更新
Attention-based Depth Distillation with 3D-Aware Positional Encoding for Monocular 3D Object Detection
Authors:Zizhang Wu, Yunzhe Wu, Jian Pu, Xianzhi Li, Xiaoquan Wang
Monocular 3D object detection is a low-cost but challenging task, as it requires generating accurate 3D localization solely from a single image input. Recent developed depth-assisted methods show promising results by using explicit depth maps as intermediate features, which are either precomputed by monocular depth estimation networks or jointly evaluated with 3D object detection. However, inevitable errors from estimated depth priors may lead to misaligned semantic information and 3D localization, hence resulting in feature smearing and suboptimal predictions. To mitigate this issue, we propose ADD, an Attention-based Depth knowledge Distillation framework with 3D-aware positional encoding. Unlike previous knowledge distillation frameworks that adopt stereo- or LiDAR-based teachers, we build up our teacher with identical architecture as the student but with extra ground-truth depth as input. Credit to our teacher design, our framework is seamless, domain-gap free, easily implementable, and is compatible with object-wise ground-truth depth. Specifically, we leverage intermediate features and responses for knowledge distillation. Considering long-range 3D dependencies, we propose \emph{3D-aware self-attention} and \emph{target-aware cross-attention} modules for student adaptation. Extensive experiments are performed to verify the effectiveness of our framework on the challenging KITTI 3D object detection benchmark. We implement our framework on three representative monocular detectors, and we achieve state-of-the-art performance with no additional inference computational cost relative to baseline models. Our code is available at https://github.com/rockywind/ADD.
PDF Accepted by AAAI2023
点此查看论文截图
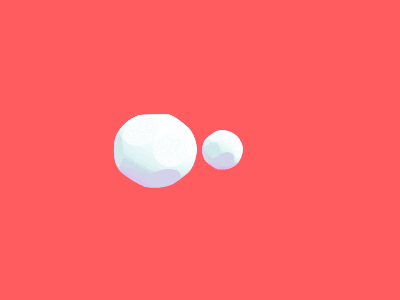
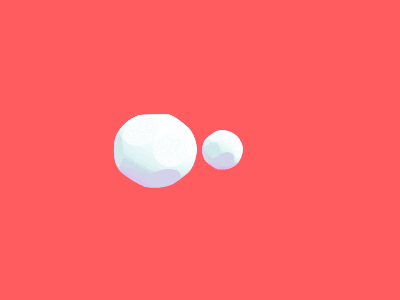
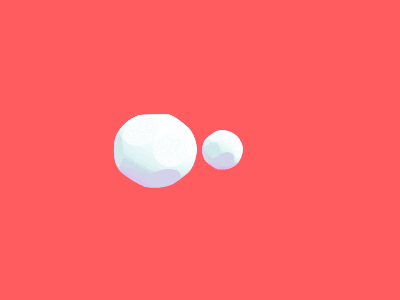
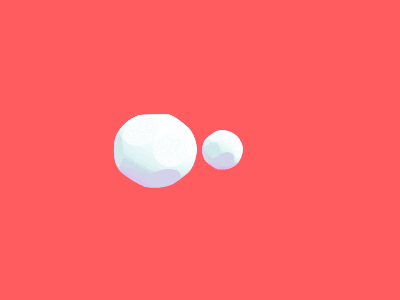
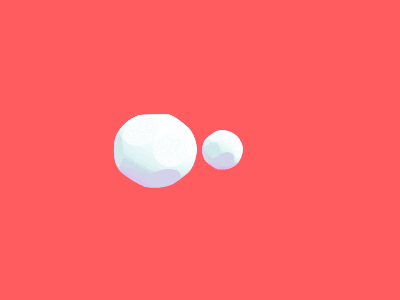
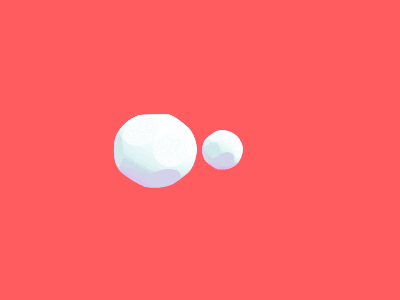
QuadFormer: Quadruple Transformer for Unsupervised Domain Adaptation in Power Line Segmentation of Aerial Images
Authors:Pratyaksh Prabhav Rao, Feng Qiao, Weide Zhang, Yiliang Xu, Yong Deng, Guangbin Wu, Qiang Zhang
Accurate segmentation of power lines in aerial images is essential to ensure the flight safety of aerial vehicles. Acquiring high-quality ground truth annotations for training a deep learning model is a laborious process. Therefore, developing algorithms that can leverage knowledge from labelled synthetic data to unlabelled real images is highly demanded. This process is studied in Unsupervised domain adaptation (UDA). Recent approaches to self-training have achieved remarkable performance in UDA for semantic segmentation, which trains a model with pseudo labels on the target domain. However, the pseudo labels are noisy due to a discrepancy in the two data distributions. We identify that context dependency is important for bridging this domain gap. Motivated by this, we propose QuadFormer, a novel framework designed for domain adaptive semantic segmentation. The hierarchical quadruple transformer combines cross-attention and self-attention mechanisms to adapt transferable context. Based on cross-attentive and self-attentive feature representations, we introduce a pseudo label correction scheme to online denoise the pseudo labels and reduce the domain gap. Additionally, we present two datasets - ARPLSyn and ARPLReal to further advance research in unsupervised domain adaptive powerline segmentation. Finally, experimental results indicate that our method achieves state-of-the-art performance for the domain adaptive power line segmentation on ARPLSyn$\rightarrow$TTTPLA and ARPLSyn$\rightarrow$ARPLReal.
PDF
点此查看论文截图
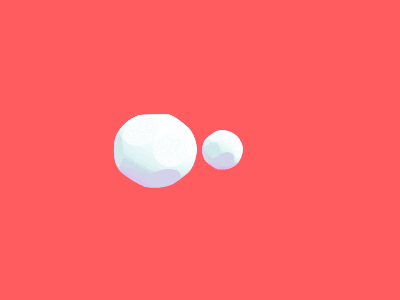
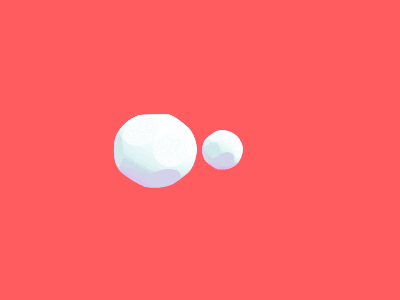
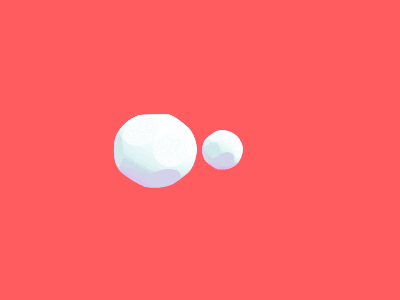
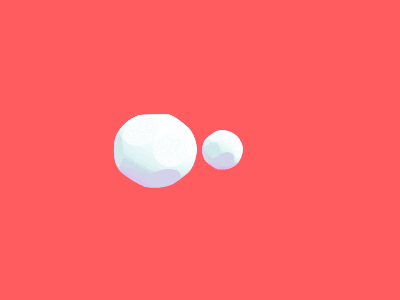
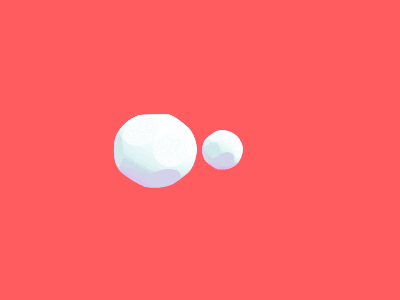
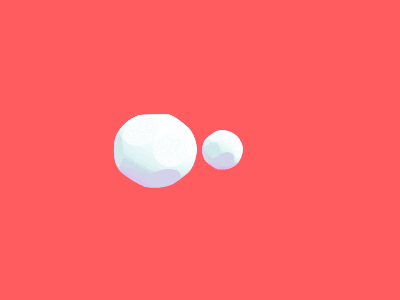
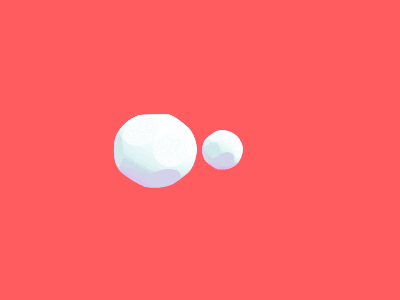
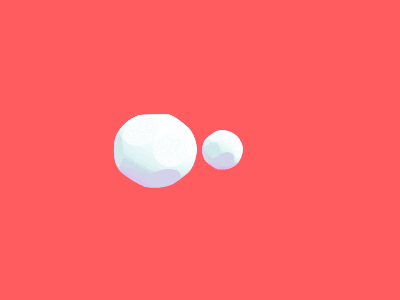
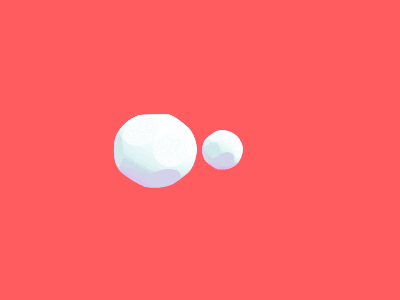
Multi-latent Space Alignments for Unsupervised Domain Adaptation in Multi-view 3D Object Detection
Authors:Jiaming Liu, Rongyu Zhang, Xiaowei Chi, Xiaoqi Li, Ming Lu, Yandong Guo, Shanghang Zhang
Vision-Centric Bird-Eye-View (BEV) perception has shown promising potential and attracted increasing attention in autonomous driving. Recent works mainly focus on improving efficiency or accuracy but neglect the domain shift problem, resulting in severe degradation of transfer performance. With extensive observations, we figure out the significant domain gaps existing in the scene, weather, and day-night changing scenarios and make the first attempt to solve the domain adaption problem for multi-view 3D object detection. Since BEV perception approaches are usually complicated and contain several components, the domain shift accumulation on multi-latent spaces makes BEV domain adaptation challenging. In this paper, we propose a novel Multi-level Multi-space Alignment Teacher-Student ($M^{2}ATS$) framework to ease the domain shift accumulation, which consists of a Depth-Aware Teacher (DAT) and a Multi-space Feature Aligned (MFA) student model. Specifically, DAT model adopts uncertainty guidance to sample reliable depth information in target domain. After constructing domain-invariant BEV perception, it then transfers pixel and instance-level knowledge to student model. To further alleviate the domain shift at the global level, MFA student model is introduced to align task-relevant multi-space features of two domains. To verify the effectiveness of $M^{2}ATS$, we conduct BEV 3D object detection experiments on four cross domain scenarios and achieve state-of-the-art performance (e.g., +12.6% NDS and +9.1% mAP on Day-Night). Code and dataset will be released.
PDF
点此查看论文截图
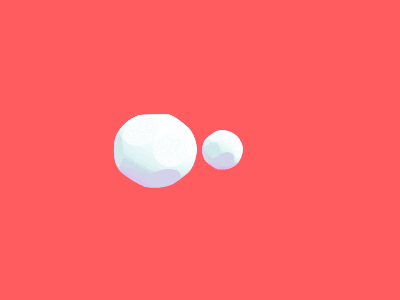
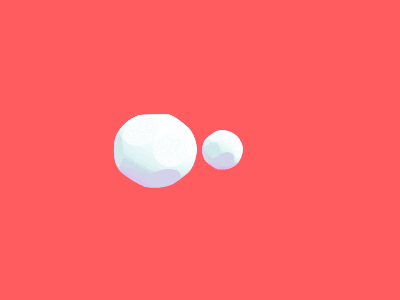
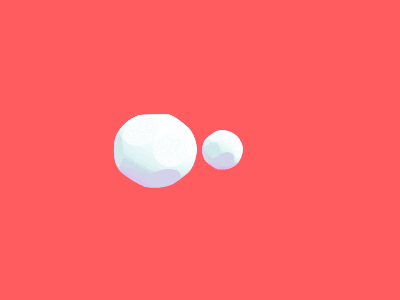
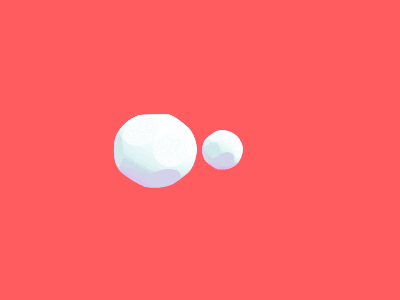
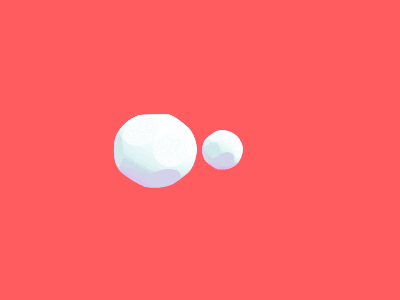
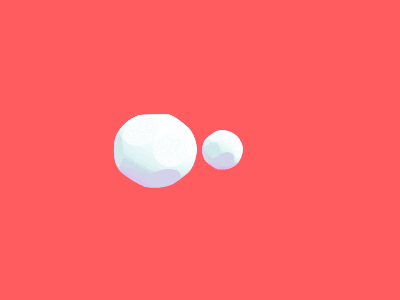
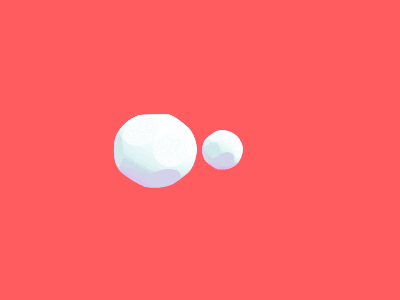
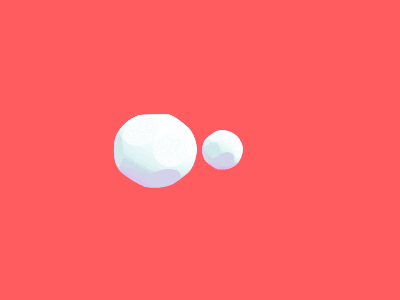
From Actions to Events: A Transfer Learning Approach Using Improved Deep Belief Networks
Authors:Mateus Roder, Jurandy Almeida, Gustavo H. de Rosa, Leandro A. Passos, André L. D. Rossi, João P. Papa
In the last decade, exponential data growth supplied machine learning-based algorithms’ capacity and enabled their usage in daily-life activities. Additionally, such an improvement is partially explained due to the advent of deep learning techniques, i.e., stacks of simple architectures that end up in more complex models. Although both factors produce outstanding results, they also pose drawbacks regarding the learning process as training complex models over large datasets are expensive and time-consuming. Such a problem is even more evident when dealing with video analysis. Some works have considered transfer learning or domain adaptation, i.e., approaches that map the knowledge from one domain to another, to ease the training burden, yet most of them operate over individual or small blocks of frames. This paper proposes a novel approach to map the knowledge from action recognition to event recognition using an energy-based model, denoted as Spectral Deep Belief Network. Such a model can process all frames simultaneously, carrying spatial and temporal information through the learning process. The experimental results conducted over two public video dataset, the HMDB-51 and the UCF-101, depict the effectiveness of the proposed model and its reduced computational burden when compared to traditional energy-based models, such as Restricted Boltzmann Machines and Deep Belief Networks.
PDF
点此查看论文截图
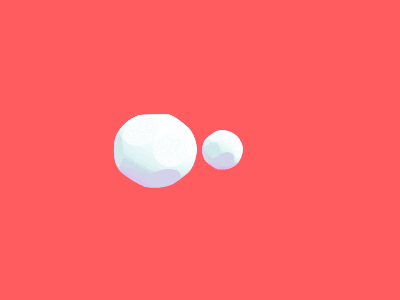
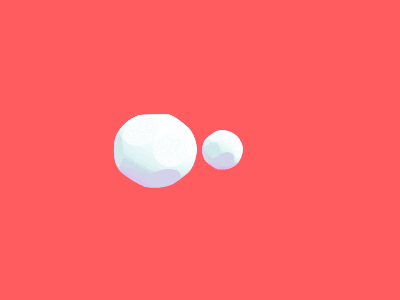
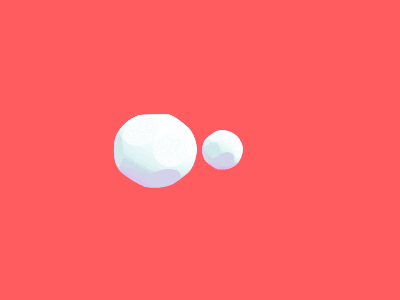
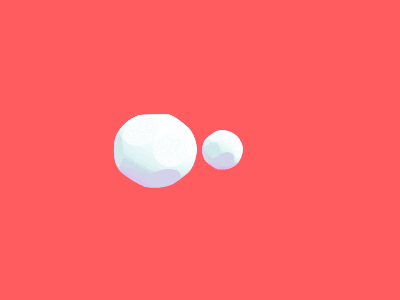
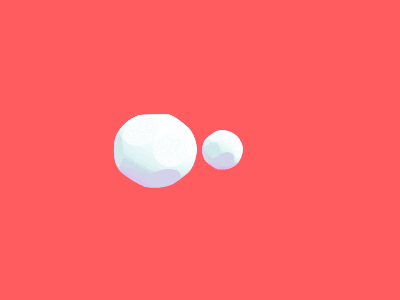
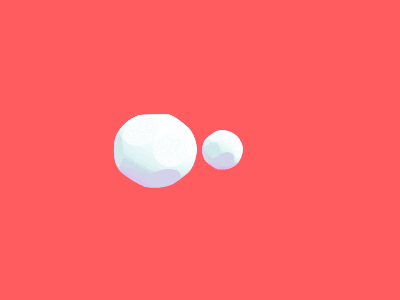
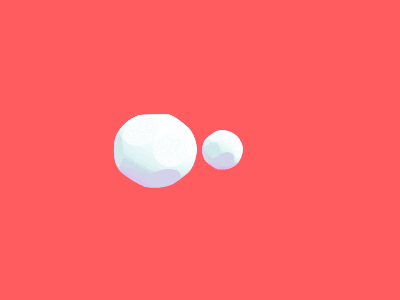
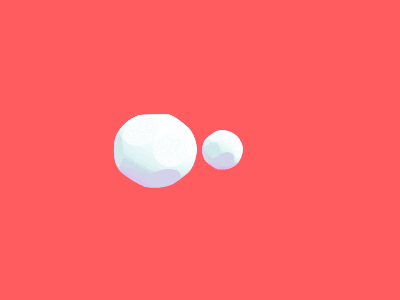