2022-11-21 更新
Video Unsupervised Domain Adaptation with Deep Learning: A Comprehensive Survey
Authors:Yuecong Xu, Haozhi Cao, Zhenghua Chen, Xiaoli Li, Lihua Xie, Jianfei Yan
Video analysis tasks such as action recognition have received increasing research interest with growing applications in fields such as smart healthcare, thanks to the introduction of large-scale datasets and deep learning-based representations. However, video models trained on existing datasets suffer from significant performance degradation when deployed directly to real-world applications due to domain shifts between the training public video datasets (source video domains) and real-world videos (target video domains). Further, with the high cost of video annotation, it is more practical to use unlabeled videos for training. To tackle performance degradation and address concerns in high video annotation cost uniformly, the video unsupervised domain adaptation (VUDA) is introduced to adapt video models from the labeled source domain to the unlabeled target domain by alleviating video domain shift, improving the generalizability and portability of video models. This paper surveys recent progress in VUDA with deep learning. We begin with the motivation of VUDA, followed by its definition, and recent progress of methods for both closed-set VUDA and VUDA under different scenarios, and current benchmark datasets for VUDA research. Eventually, future directions are provided to promote further VUDA research.
PDF Survey on Video Unsupervised Domain Adaptation (VUDA), 16 pages, 1 figure, 8 tables
点此查看论文截图
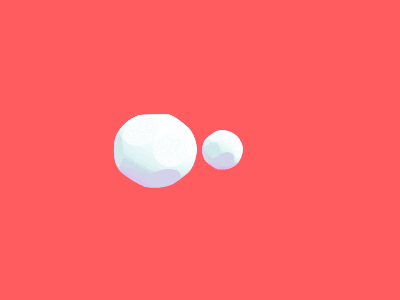
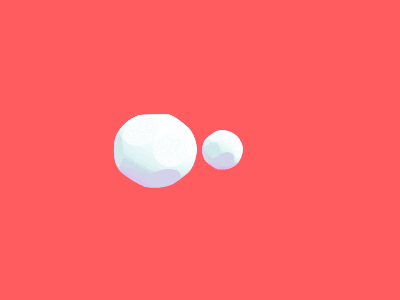
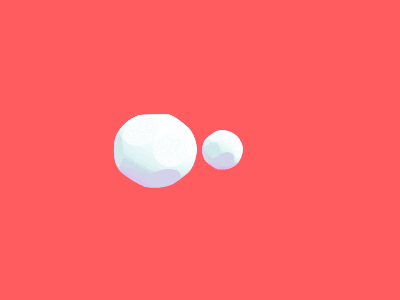
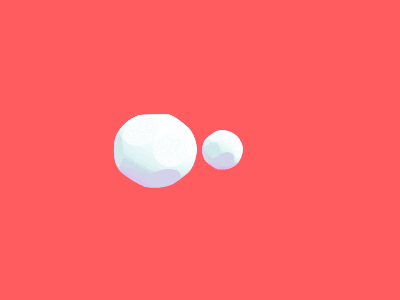
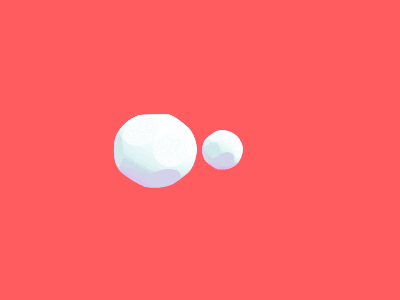
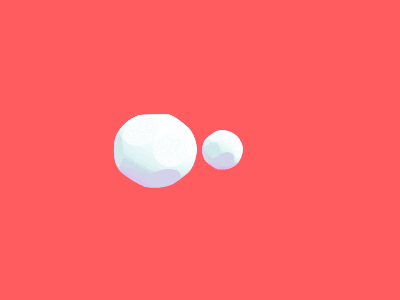
Mixture Domain Adaptation to Improve Semantic Segmentation in Real-World Surveillance
Authors:Sébastien Piérard, Anthony Cioppa, Anaïs Halin, Renaud Vandeghen, Maxime Zanella, Benoît Macq, Saïd Mahmoudi, Marc Van Droogenbroeck
Various tasks encountered in real-world surveillance can be addressed by determining posteriors (e.g. by Bayesian inference or machine learning), based on which critical decisions must be taken. However, the surveillance domain (acquisition device, operating conditions, etc.) is often unknown, which prevents any possibility of scene-specific optimization. In this paper, we define a probabilistic framework and present a formal proof of an algorithm for the unsupervised many-to-infinity domain adaptation of posteriors. Our proposed algorithm is applicable when the probability measure associated with the target domain is a convex combination of the probability measures of the source domains. It makes use of source models and a domain discriminator model trained off-line to compute posteriors adapted on the fly to the target domain. Finally, we show the effectiveness of our algorithm for the task of semantic segmentation in real-world surveillance. The code is publicly available at https://github.com/rvandeghen/MDA.
PDF
点此查看论文截图
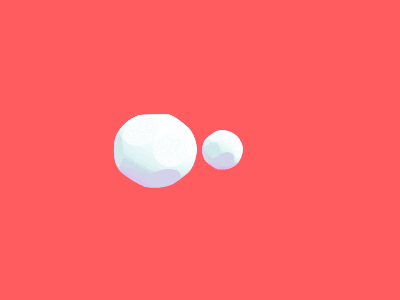
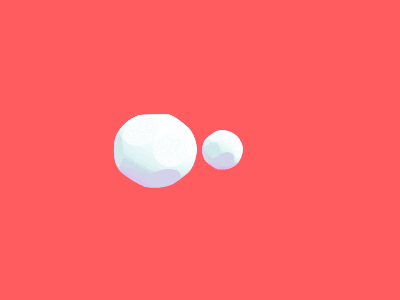
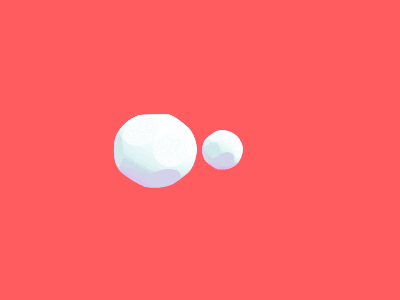
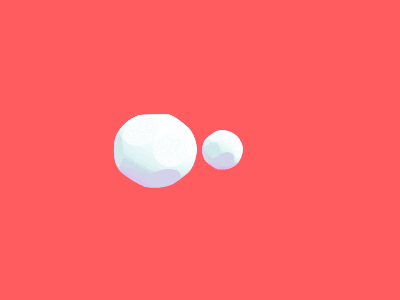
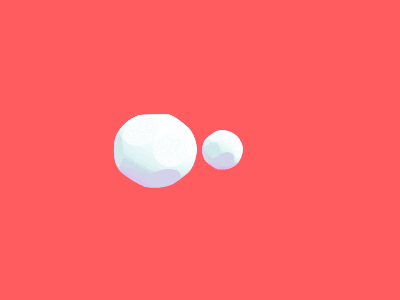
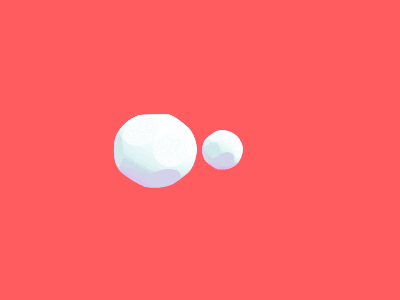
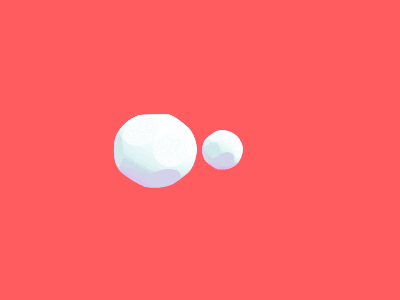