2022-11-19 更新
Sources of performance variability in deep learning-based polyp detection
Authors:Thuy Nuong Tran, Tim Adler, Amine Yamlahi, Evangelia Christodoulou, Patrick Godau, Annika Reinke, Minu Dietlinde Tizabi, Peter Sauer, Tillmann Persicke, Jörg Gerhard Albert, Lena Maier-Hein
Validation metrics are a key prerequisite for the reliable tracking of scientific progress and for deciding on the potential clinical translation of methods. While recent initiatives aim to develop comprehensive theoretical frameworks for understanding metric-related pitfalls in image analysis problems, there is a lack of experimental evidence on the concrete effects of common and rare pitfalls on specific applications. We address this gap in the literature in the context of colon cancer screening. Our contribution is twofold. Firstly, we present the winning solution of the Endoscopy computer vision challenge (EndoCV) on colon cancer detection, conducted in conjunction with the IEEE International Symposium on Biomedical Imaging (ISBI) 2022. Secondly, we demonstrate the sensitivity of commonly used metrics to a range of hyperparameters as well as the consequences of poor metric choices. Based on comprehensive validation studies performed with patient data from six clinical centers, we found all commonly applied object detection metrics to be subject to high inter-center variability. Furthermore, our results clearly demonstrate that the adaptation of standard hyperparameters used in the computer vision community does not generally lead to the clinically most plausible results. Finally, we present localization criteria that correspond well to clinical relevance. Our work could be a first step towards reconsidering common validation strategies in automatic colon cancer screening applications.
PDF 12 pages, 9 figures, 3 tables. Submitted to IPCAI 2023
点此查看论文截图
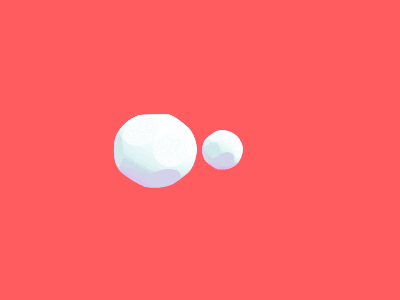
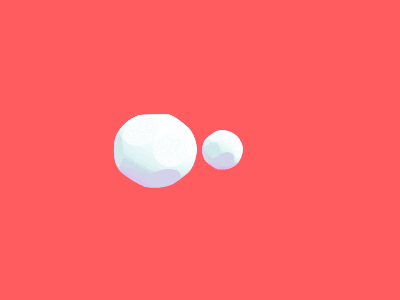
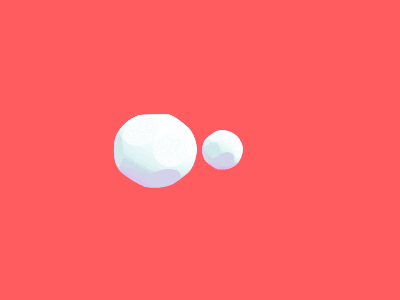
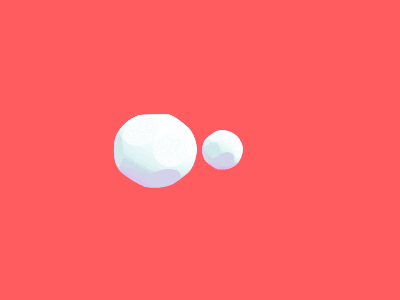
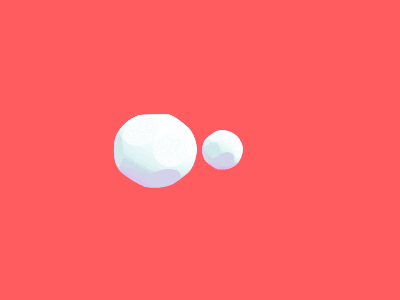
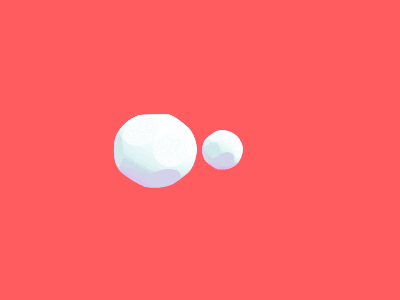
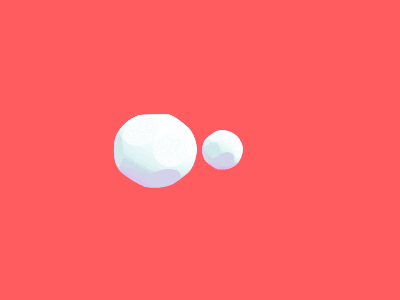
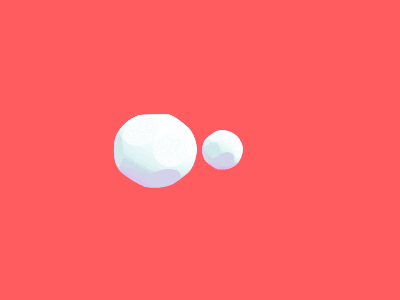
Generalization to translation shifts: a study in architectures and augmentations
Authors:Suriya Gunasekar
We study how effective data augmentation is at capturing the inductive bias of carefully designed network architectures for spatial translation invariance. We evaluate various image classification architectures (antialiased, convolutional, vision transformer, and fully connected MLP networks) and data augmentation techniques towards generalization to large translation shifts. We observe that: (a) without data augmentation, all architectures, including convolutional networks with antialiased modification suffer some degradation in performance when evaluated on translated test distributions. Understandably, both the in-distribution accuracy and degradation to shifts is significantly worse for non-convolutional models. (b) The robustness of performance is improved by even a minimal augmentation of $4$ pixel random crop across all architectures. In some instances, even $1-2$ pixel random crop is sufficient. This suggests that there is a form of meta generalization from augmentation. For non-convolutional architectures, while the absolute accuracy is still low with this basic augmentation, we see substantial improvements in robustness to translation shifts. (c) With a sufficiently advanced augmentation pipeline ($4$ pixel crop+RandAugmentation+Erasing+MixUp), all architectures can be trained to have competitive performance in terms of in-distribution accuracy as well as generalization to large translation shifts.
PDF
点此查看论文截图
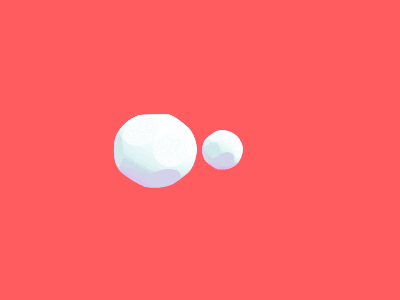
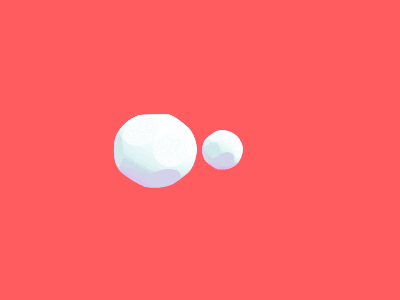
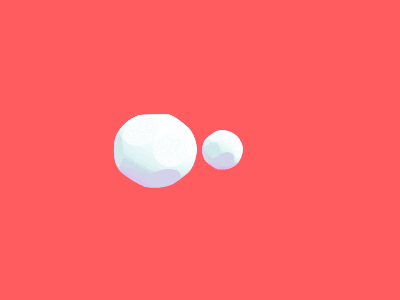
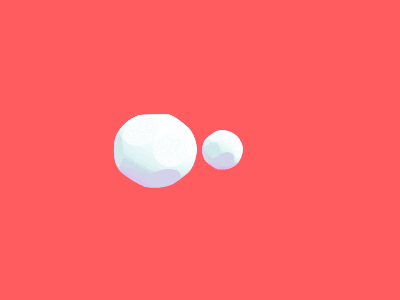
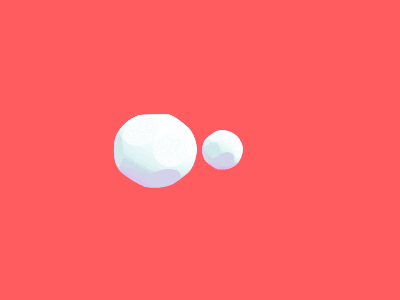
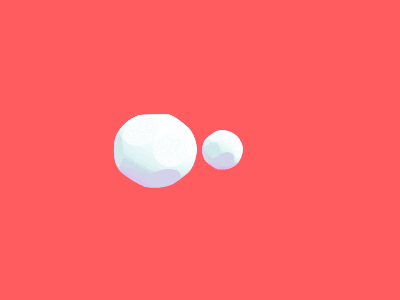
Marine Microalgae Detection in Microscopy Images: A New Dataset
Authors:Shizheng Zhou, Juntao Jiang, Xiaohan Hong, Yajun Fang, Yan Hong, Pengcheng Fu
Marine microalgae are widespread in the ocean and play a crucial role in the ecosystem. Automatic identification and location of marine microalgae in microscopy images would help establish marine ecological environment monitoring and water quality evaluation system. A new dataset for marine microalgae detection is proposed in this paper. Six classes of microalgae commonlyfound in the ocean (Bacillariophyta, Chlorella pyrenoidosa, Platymonas, Dunaliella salina, Chrysophyta, Symbiodiniaceae) are microscopically imaged in real-time. Images of Symbiodiniaceae in three physiological states known as normal, bleaching, and translating are also included. We annotated these images with bounding boxes using Labelme software and split them into the training and testing sets. The total number of images in the dataset is 937 and all the objects in these images were annotated. The total number of annotated objects is 4201. The training set contains 537 images and the testing set contains 430 images. Baselines of different object detection algorithms are trained, validated and tested on this dataset. This data set can be got accessed via tianchi.aliyun.com/competition/entrance/532036/information.
PDF
点此查看论文截图
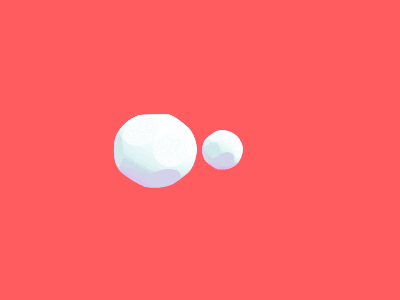
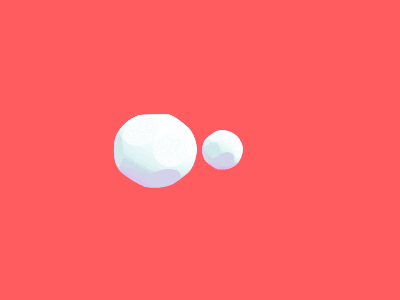
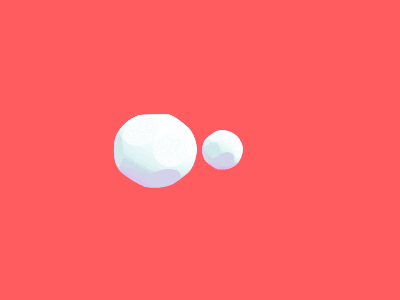
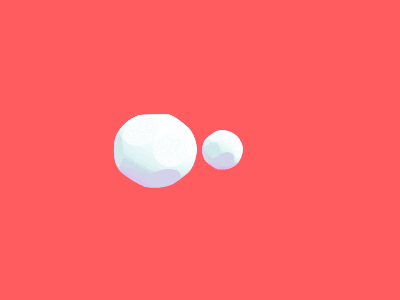
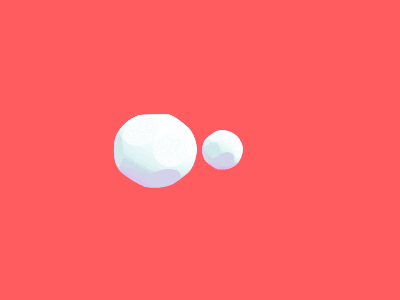
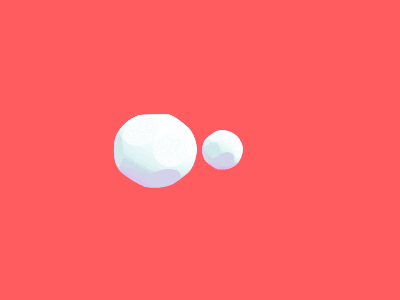
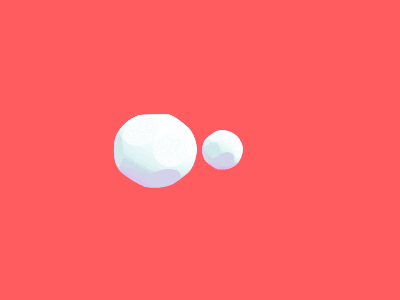
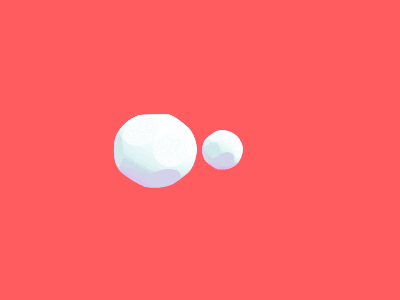