2022-10-15 更新
Rigidity Preserving Image Transformations and Equivariance in Perspective
Authors:Lucas Brynte, Georg Bökman, Axel Flinth, Fredrik Kahl
We characterize the class of image plane transformations which realize rigid camera motions and call these transformations `rigidity preserving’. In particular, 2D translations of pinhole images are not rigidity preserving. Hence, when using CNNs for 3D inference tasks, it can be beneficial to modify the inductive bias from equivariance towards translations to equivariance towards rigidity preserving transformations. We investigate how equivariance with respect to rigidity preserving transformations can be approximated in CNNs, and test our ideas on both 6D object pose estimation and visual localization. Experimentally, we improve on several competitive baselines.
PDF v2: Substantially revised version. Among other things, experiments with the PixLoc model added
点此查看论文截图
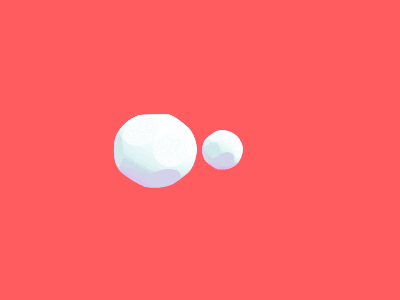
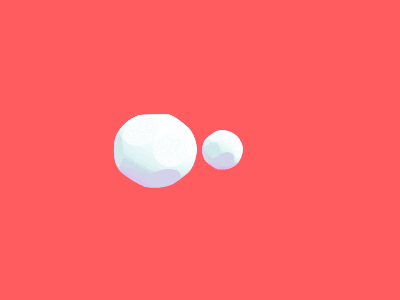
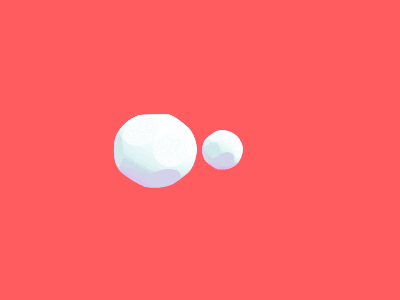
Computational Choreography using Human Motion Synthesis
Authors:Patrick Perrine, Trevor Kirkby
Should deep learning models be trained to analyze human performance art? To help answer this question, we explore an application of deep neural networks to synthesize artistic human motion. Problem tasks in human motion synthesis can include predicting the motions of humans in-the-wild, as well as generating new sequences of motions based on said predictions. We will discuss the potential of a less traditional application, where learning models are applied to predicting dance movements. There have been notable, recent efforts to analyze dance movements in a computational light, such as the Everybody Dance Now (EDN) learning model and a recent Cal Poly master’s thesis, Take The Lead (TTL). We have effectively combined these two works along with our own deep neural network to produce a new system for dance motion prediction, image-to-image translation, and video generation.
PDF 6 pages, 7 figures, to be submitted to CVPR 2023
点此查看论文截图
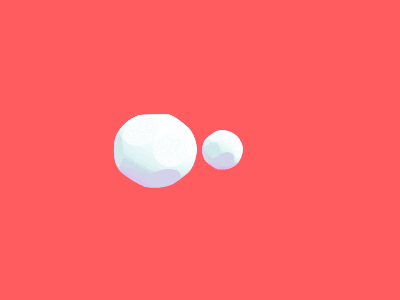
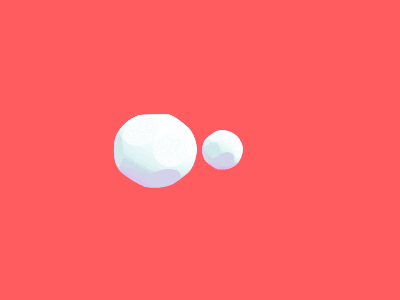
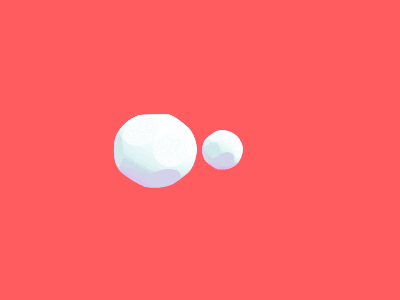
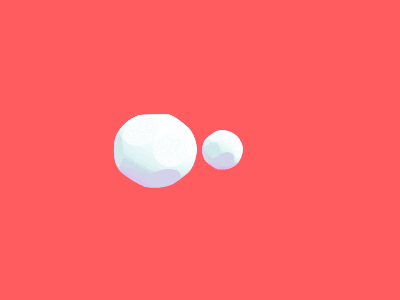
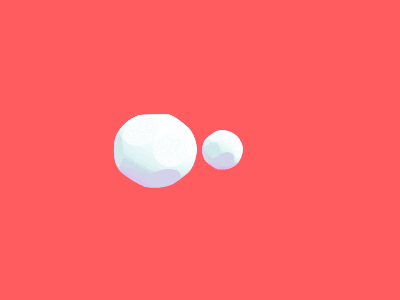
Crossmodal-3600: A Massively Multilingual Multimodal Evaluation Dataset
Authors:Ashish V. Thapliyal, Jordi Pont-Tuset, Xi Chen, Radu Soricut
Research in massively multilingual image captioning has been severely hampered by a lack of high-quality evaluation datasets. In this paper we present the Crossmodal-3600 dataset (XM3600 in short), a geographically diverse set of 3600 images annotated with human-generated reference captions in 36 languages. The images were selected from across the world, covering regions where the 36 languages are spoken, and annotated with captions that achieve consistency in terms of style across all languages, while avoiding annotation artifacts due to direct translation. We apply this benchmark to model selection for massively multilingual image captioning models, and show superior correlation results with human evaluations when using XM3600 as golden references for automatic metrics.
PDF EMNLP 2022
点此查看论文截图
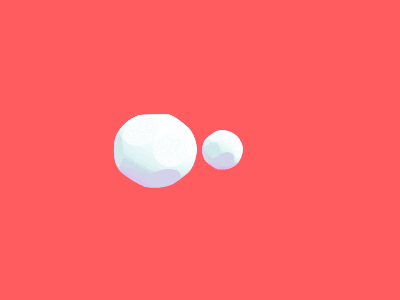
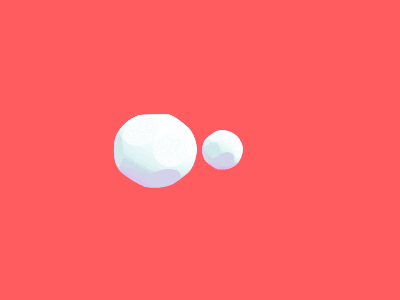
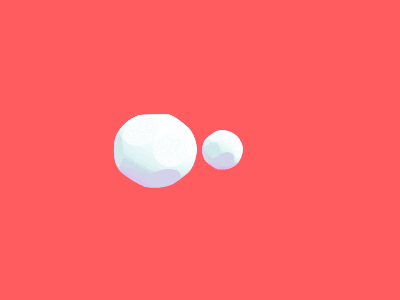
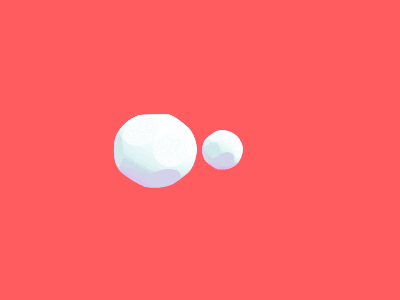
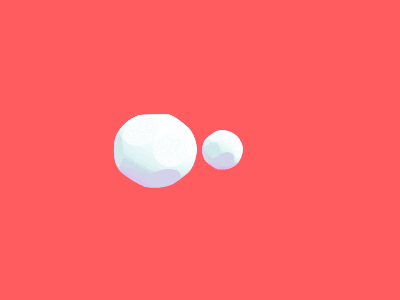
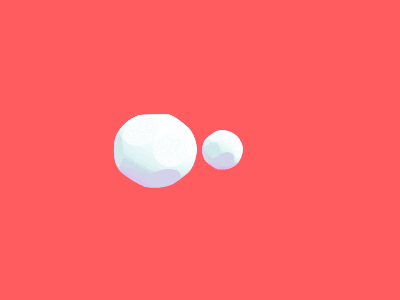
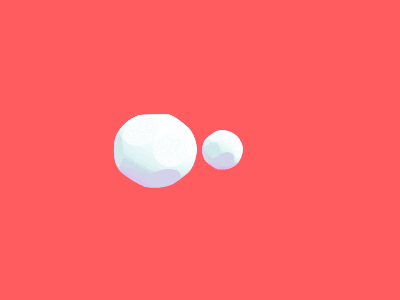
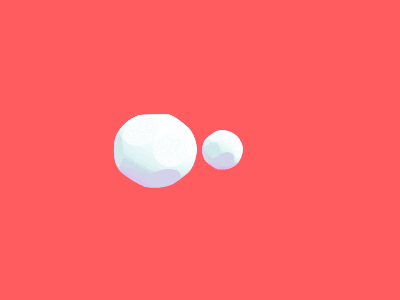
Neuromorphic Visual Scene Understanding with Resonator Networks
Authors:Alpha Renner, Lazar Supic, Andreea Danielescu, Giacomo Indiveri, Bruno A. Olshausen, Yulia Sandamirskaya, Friedrich T. Sommer, E. Paxon Frady
Inferring the position of objects and their rigid transformations is still an open problem in visual scene understanding. Here we propose a neuromorphic solution that utilizes an efficient factorization network based on three key concepts: (1) a computational framework based on Vector Symbolic Architectures (VSA) with complex-valued vectors; (2) the design of Hierarchical Resonator Networks (HRN) to deal with the non-commutative nature of translation and rotation in visual scenes, when both are used in combination; (3) the design of a multi-compartment spiking phasor neuron model for implementing complex-valued vector binding on neuromorphic hardware. The VSA framework uses vector binding operations to produce generative image models in which binding acts as the equivariant operation for geometric transformations. A scene can therefore be described as a sum of vector products, which in turn can be efficiently factorized by a resonator network to infer objects and their poses. The HRN enables the definition of a partitioned architecture in which vector binding is equivariant for horizontal and vertical translation within one partition and for rotation and scaling within the other partition. The spiking neuron model allows mapping the resonator network onto efficient and low-power neuromorphic hardware. In this work, we demonstrate our approach using synthetic scenes composed of simple 2D shapes undergoing rigid geometric transformations and color changes. A companion paper demonstrates this approach in real-world application scenarios for machine vision and robotics.
PDF 15 pages, 6 figures, minor changes
点此查看论文截图
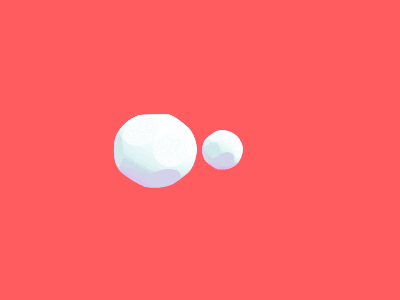
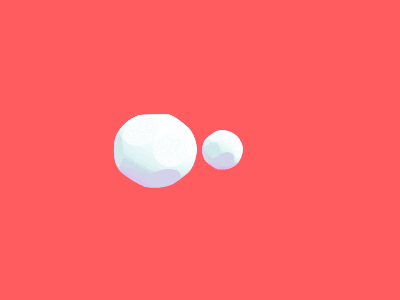
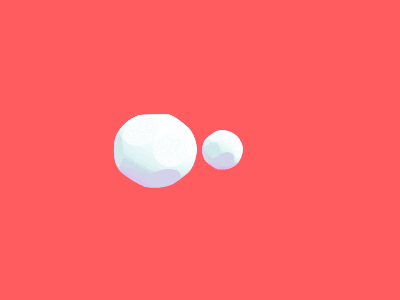
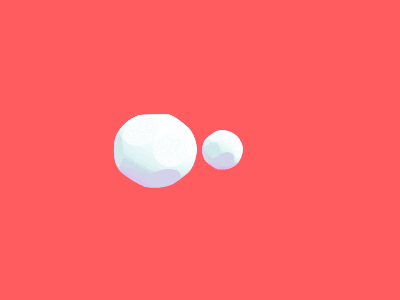
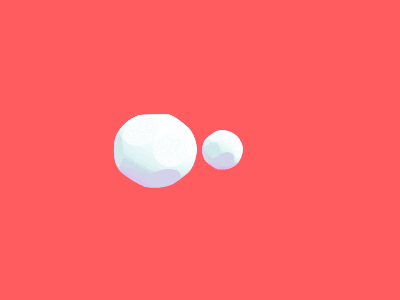
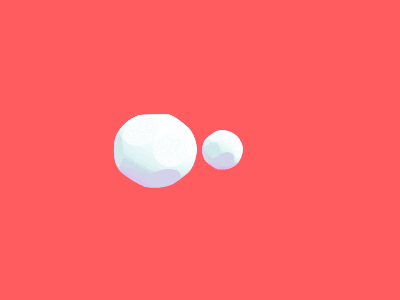
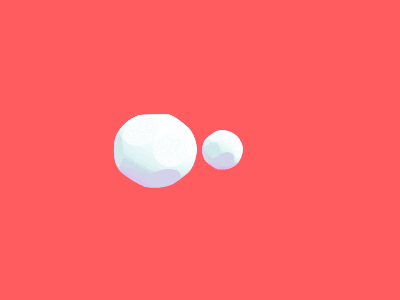
Probabilistic Integration of Object Level Annotations in Chest X-ray Classification
Authors:Tom van Sonsbeek, Xiantong Zhen, Dwarikanath Mahapatra, Marcel Worring
Medical image datasets and their annotations are not growing as fast as their equivalents in the general domain. This makes translation from the newest, more data-intensive methods that have made a large impact on the vision field increasingly more difficult and less efficient. In this paper, we propose a new probabilistic latent variable model for disease classification in chest X-ray images. Specifically we consider chest X-ray datasets that contain global disease labels, and for a smaller subset contain object level expert annotations in the form of eye gaze patterns and disease bounding boxes. We propose a two-stage optimization algorithm which is able to handle these different label granularities through a single training pipeline in a two-stage manner. In our pipeline global dataset features are learned in the lower level layers of the model. The specific details and nuances in the fine-grained expert object-level annotations are learned in the final layers of the model using a knowledge distillation method inspired by conditional variational inference. Subsequently, model weights are frozen to guide this learning process and prevent overfitting on the smaller richly annotated data subsets. The proposed method yields consistent classification improvement across different backbones on the common benchmark datasets Chest X-ray14 and MIMIC-CXR. This shows how two-stage learning of labels from coarse to fine-grained, in particular with object level annotations, is an effective method for more optimal annotation usage.
PDF WACV 2023
点此查看论文截图
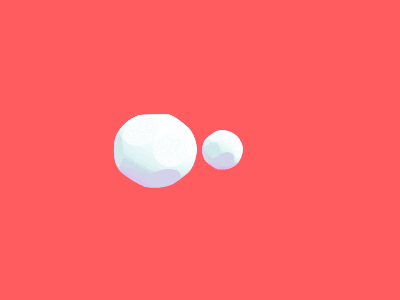
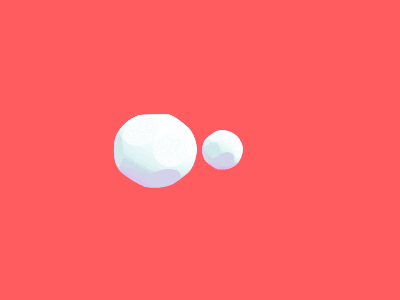
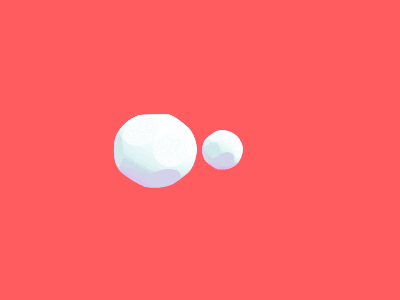
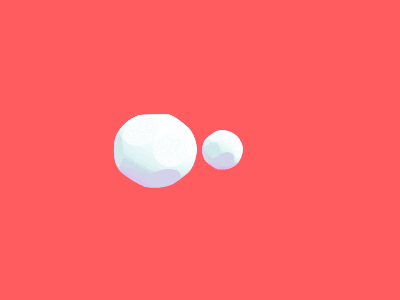
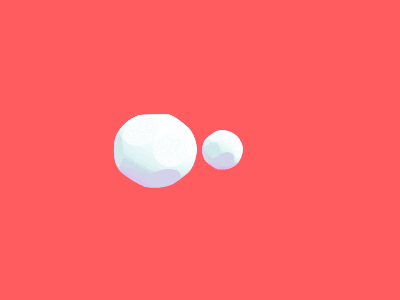
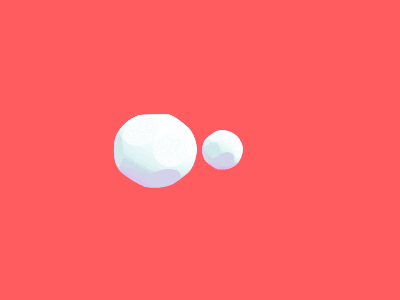
What can we learn about a generated image corrupting its latent representation?
Authors:Agnieszka Tomczak, Aarushi Gupta, Slobodan Ilic, Nassir Navab, Shadi Albarqouni
Generative adversarial networks (GANs) offer an effective solution to the image-to-image translation problem, thereby allowing for new possibilities in medical imaging. They can translate images from one imaging modality to another at a low cost. For unpaired datasets, they rely mostly on cycle loss. Despite its effectiveness in learning the underlying data distribution, it can lead to a discrepancy between input and output data. The purpose of this work is to investigate the hypothesis that we can predict image quality based on its latent representation in the GANs bottleneck. We achieve this by corrupting the latent representation with noise and generating multiple outputs. The degree of differences between them is interpreted as the strength of the representation: the more robust the latent representation, the fewer changes in the output image the corruption causes. Our results demonstrate that our proposed method has the ability to i) predict uncertain parts of synthesized images, and ii) identify samples that may not be reliable for downstream tasks, e.g., liver segmentation task.
PDF
点此查看论文截图
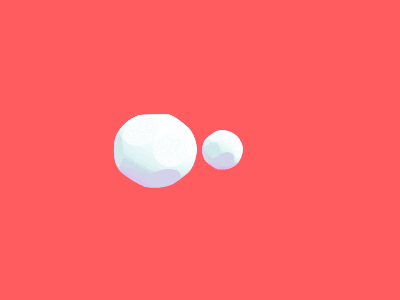
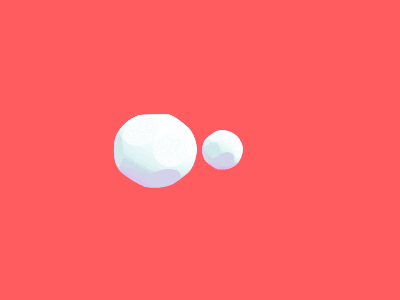
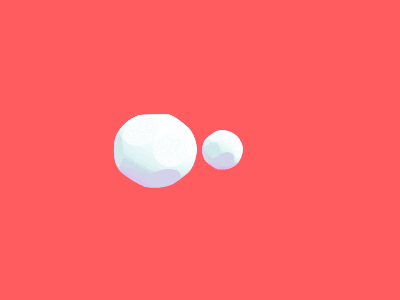
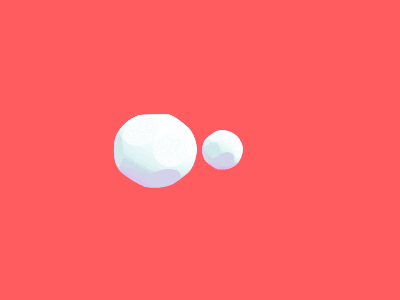
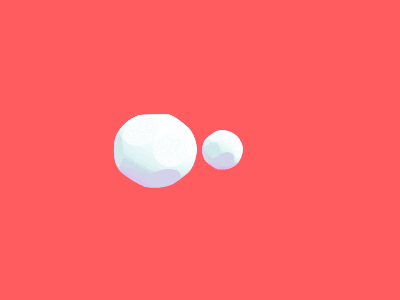
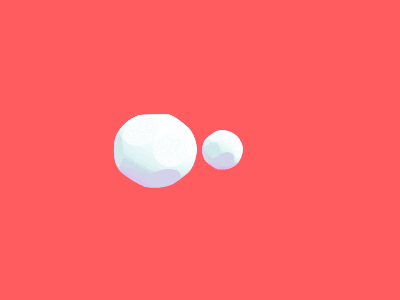