2022-10-11 更新
Learning Fine-Grained Visual Understanding for Video Question Answering via Decoupling Spatial-Temporal Modeling
Authors:Hsin-Ying Lee, Hung-Ting Su, Bing-Chen Tsai, Tsung-Han Wu, Jia-Fong Yeh, Winston H. Hsu
While recent large-scale video-language pre-training made great progress in video question answering, the design of spatial modeling of video-language models is less fine-grained than that of image-language models; existing practices of temporal modeling also suffer from weak and noisy alignment between modalities. To learn fine-grained visual understanding, we decouple spatial-temporal modeling and propose a hybrid pipeline, Decoupled Spatial-Temporal Encoders, integrating an image- and a video-language encoder. The former encodes spatial semantics from larger but sparsely sampled frames independently of time, while the latter models temporal dynamics at lower spatial but higher temporal resolution. To help the video-language model learn temporal relations for video QA, we propose a novel pre-training objective, Temporal Referring Modeling, which requires the model to identify temporal positions of events in video sequences. Extensive experiments demonstrate that our model outperforms previous work pre-trained on orders of magnitude larger datasets.
PDF BMVC 2022. Code is available at https://github.com/shinying/dest
点此查看论文截图
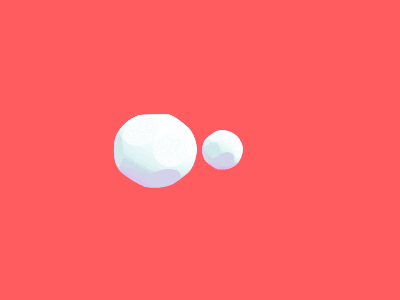
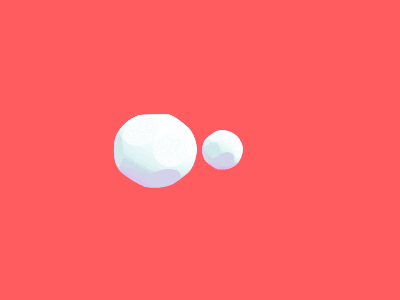
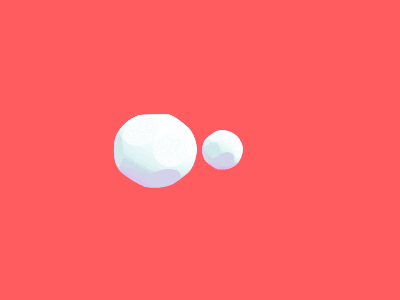
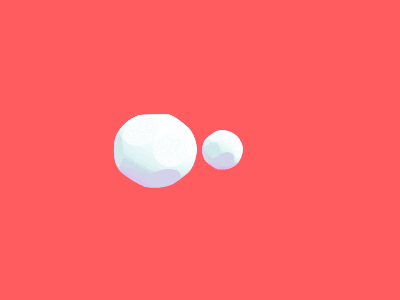
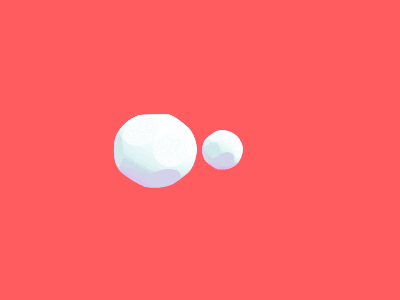
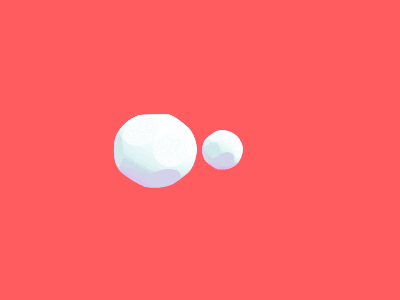
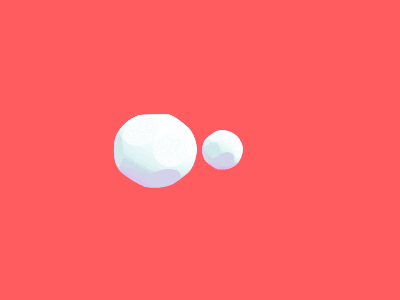
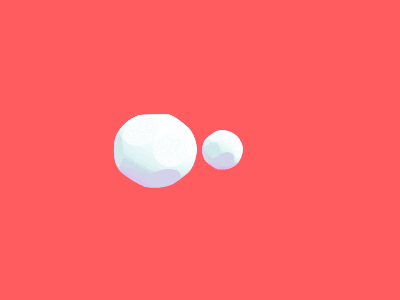
EgoTaskQA: Understanding Human Tasks in Egocentric Videos
Authors:Baoxiong Jia, Ting Lei, Song-Chun Zhu, Siyuan Huang
Understanding human tasks through video observations is an essential capability of intelligent agents. The challenges of such capability lie in the difficulty of generating a detailed understanding of situated actions, their effects on object states (i.e., state changes), and their causal dependencies. These challenges are further aggravated by the natural parallelism from multi-tasking and partial observations in multi-agent collaboration. Most prior works leverage action localization or future prediction as an indirect metric for evaluating such task understanding from videos. To make a direct evaluation, we introduce the EgoTaskQA benchmark that provides a single home for the crucial dimensions of task understanding through question-answering on real-world egocentric videos. We meticulously design questions that target the understanding of (1) action dependencies and effects, (2) intents and goals, and (3) agents’ beliefs about others. These questions are divided into four types, including descriptive (what status?), predictive (what will?), explanatory (what caused?), and counterfactual (what if?) to provide diagnostic analyses on spatial, temporal, and causal understandings of goal-oriented tasks. We evaluate state-of-the-art video reasoning models on our benchmark and show their significant gaps between humans in understanding complex goal-oriented egocentric videos. We hope this effort will drive the vision community to move onward with goal-oriented video understanding and reasoning.
PDF Published at NeurIPS Track on Datasets and Benchmarks 2022
点此查看论文截图
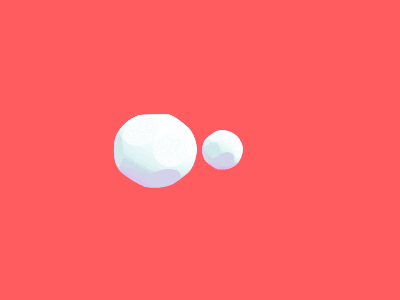
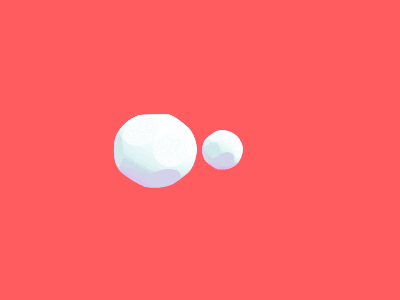
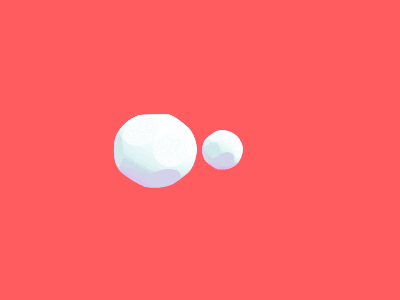
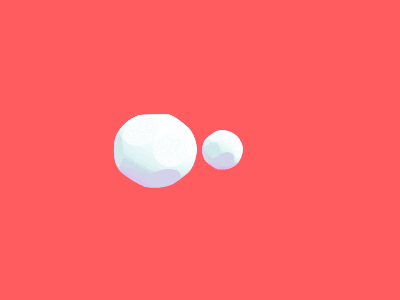
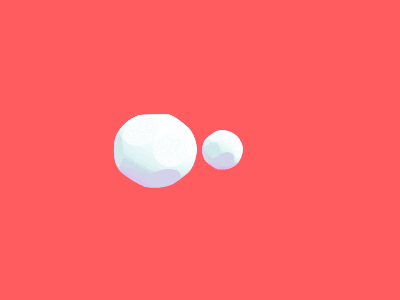
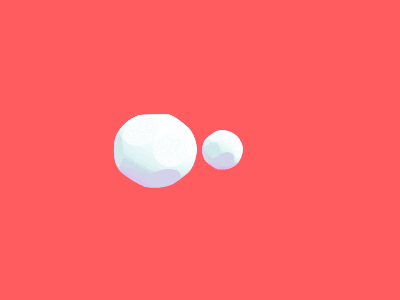
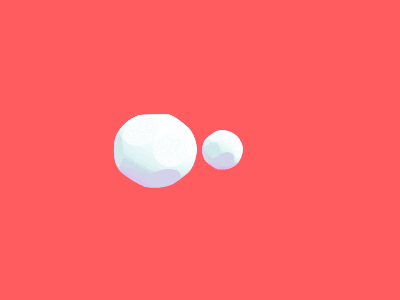
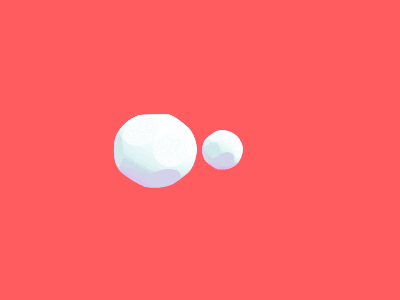
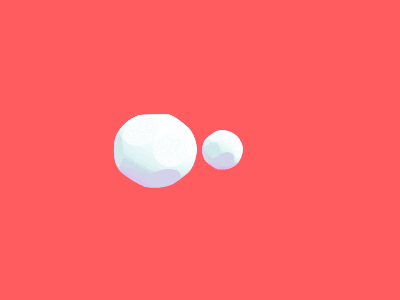