2022-10-06 更新
ciDATGAN: Conditional Inputs for Tabular GANs
Authors:Gael Lederrey, Tim Hillel, Michel Bierlaire
Conditionality has become a core component for Generative Adversarial Networks (GANs) for generating synthetic images. GANs are usually using latent conditionality to control the generation process. However, tabular data only contains manifest variables. Thus, latent conditionality either restricts the generated data or does not produce sufficiently good results. Therefore, we propose a new methodology to include conditionality in tabular GANs inspired by image completion methods. This article presents ciDATGAN, an evolution of the Directed Acyclic Tabular GAN (DATGAN) that has already been shown to outperform state-of-the-art tabular GAN models. First, we show that the addition of conditional inputs does hinder the model’s performance compared to its predecessor. Then, we demonstrate that ciDATGAN can be used to unbias datasets with the help of well-chosen conditional inputs. Finally, it shows that ciDATGAN can learn the logic behind the data and, thus, be used to complete large synthetic datasets using data from a smaller feeder dataset.
PDF Technical report, 21 pages
点此查看论文截图
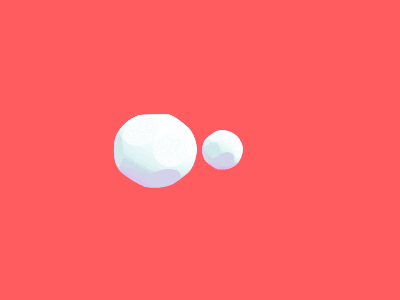
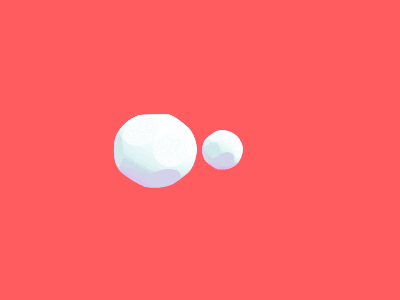
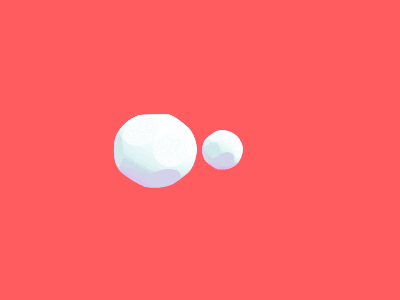
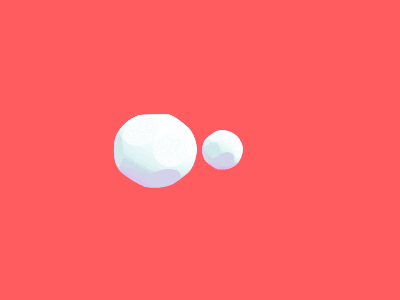
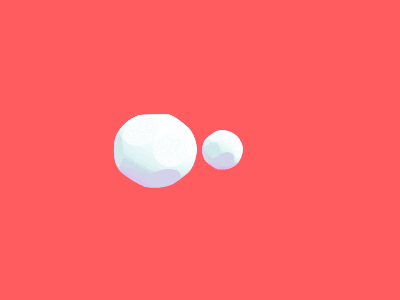
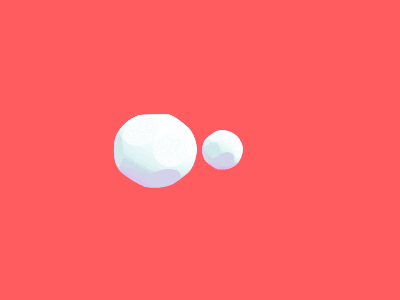
On the Robustness of Deep Clustering Models: Adversarial Attacks and Defenses
Authors:Anshuman Chhabra, Ashwin Sekhari, Prasant Mohapatra
Clustering models constitute a class of unsupervised machine learning methods which are used in a number of application pipelines, and play a vital role in modern data science. With recent advancements in deep learning — deep clustering models have emerged as the current state-of-the-art over traditional clustering approaches, especially for high-dimensional image datasets. While traditional clustering approaches have been analyzed from a robustness perspective, no prior work has investigated adversarial attacks and robustness for deep clustering models in a principled manner. To bridge this gap, we propose a blackbox attack using Generative Adversarial Networks (GANs) where the adversary does not know which deep clustering model is being used, but can query it for outputs. We analyze our attack against multiple state-of-the-art deep clustering models and real-world datasets, and find that it is highly successful. We then employ some natural unsupervised defense approaches, but find that these are unable to mitigate our attack. Finally, we attack Face++, a production-level face clustering API service, and find that we can significantly reduce its performance as well. Through this work, we thus aim to motivate the need for truly robust deep clustering models.
PDF Accepted to the 36th Conference on Neural Information Processing Systems (NeurIPS 2022)
点此查看论文截图
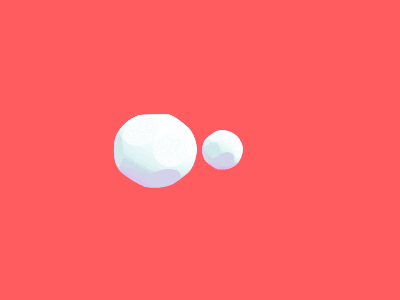
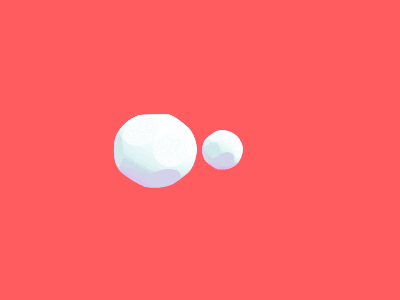
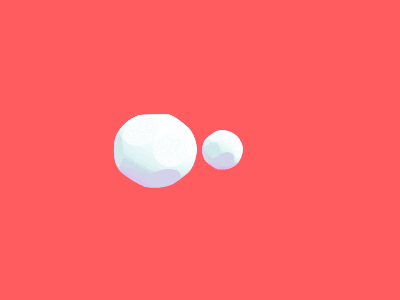
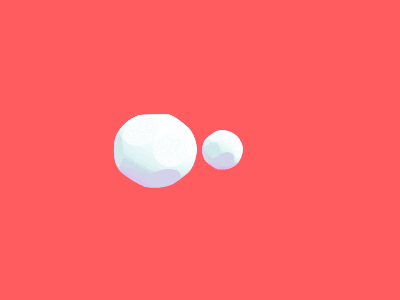
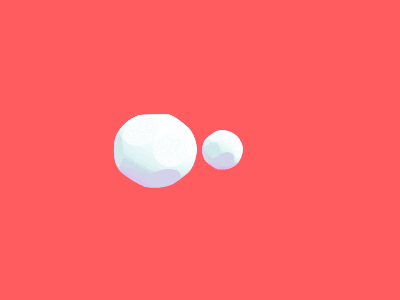
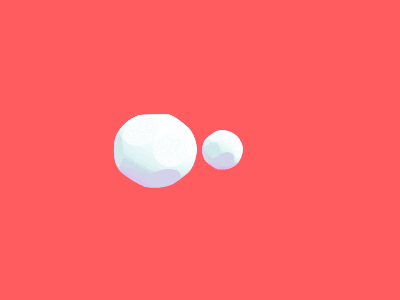
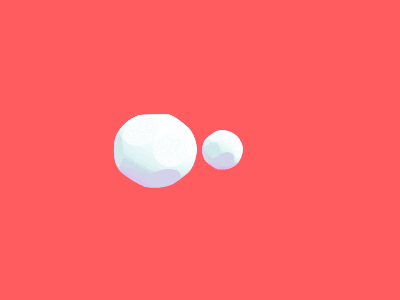
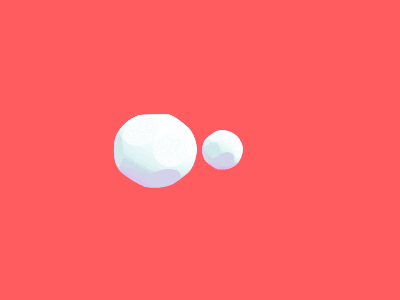