2022-10-04 更新
Achieving Domain Generalization in Underwater Object Detection by Domain Mixup and Contrastive Learning
Authors:Yang Chen, Hong Liu, Pinhao Song, Linhui Dai, Xiaochuan Zhang, Runwei Ding, Shengquan Li
The performance of existing underwater object detection methods degrades seriously when facing domain shift caused by complicated underwater environments. Due to the limitation of the number of domains in the dataset, deep detectors easily memorize a few seen domains, which leads to low generalization ability. There are two common ideas to improve the domain generalization performance. First, it can be inferred that the detector trained on as many domains as possible is domain-invariant. Second, for the images with the same semantic content in different domains, their hidden features should be equivalent. This paper further excavates these two ideas and proposes a domain generalization framework (named DMC) that learns how to generalize across domains from Domain Mixup and Contrastive Learning. First, based on the formation of underwater images, an image in an underwater environment is the linear transformation of another underwater environment. Thus, a style transfer model, which outputs a linear transformation matrix instead of the whole image, is proposed to transform images from one source domain to another, enriching the domain diversity of the training data. Second, mixup operation interpolates different domains on the feature level, sampling new domains on the domain manifold. Third, contrastive loss is selectively applied to features from different domains to force the model to learn domain invariant features but retain the discriminative capacity. With our method, detectors will be robust to domain shift. Also, a domain generalization benchmark S-UODAC2020 for detection is set up to measure the performance of our method. Comprehensive experiments on S-UODAC2020 and two object recognition benchmarks (PACS and VLCS) demonstrate that the proposed method is able to learn domain-invariant representations, and outperforms other domain generalization methods.
PDF
点此查看论文截图
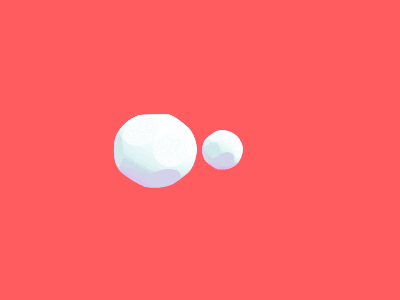
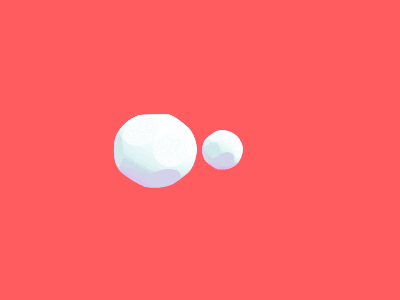
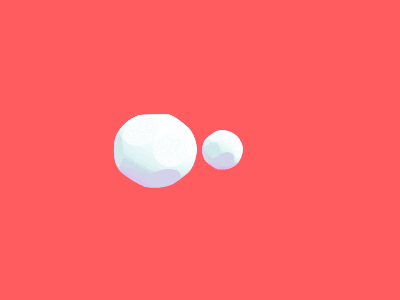
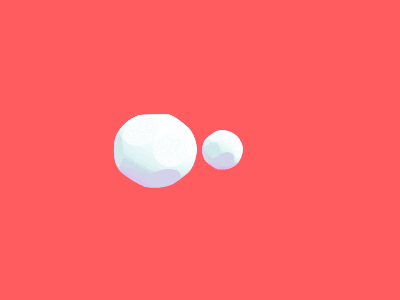
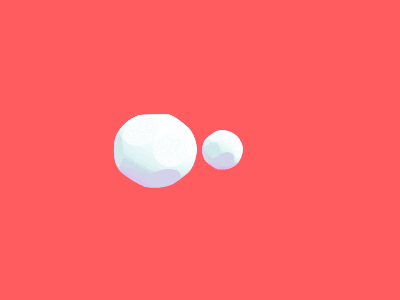
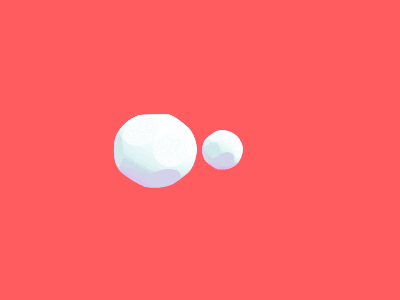
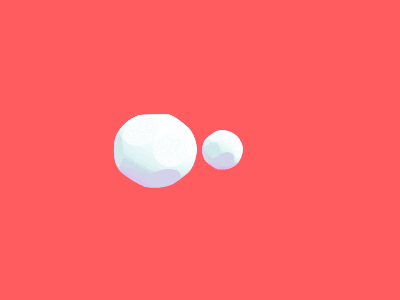
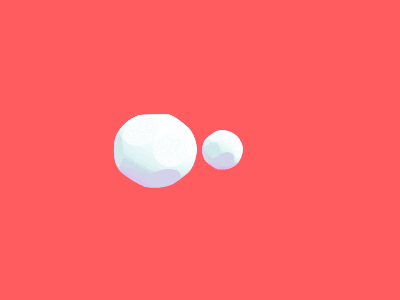
Learning Equivariant Segmentation with Instance-Unique Querying
Authors:Wenguan Wang, James Liang, Dongfang Liu
Prevalent state-of-the-art instance segmentation methods fall into a query-based scheme, in which instance masks are derived by querying the image feature using a set of instance-aware embeddings. In this work, we devise a new training framework that boosts query-based models through discriminative query embedding learning. It explores two essential properties, namely dataset-level uniqueness and transformation equivariance, of the relation between queries and instances. First, our algorithm uses the queries to retrieve the corresponding instances from the whole training dataset, instead of only searching within individual scenes. As querying instances across scenes is more challenging, the segmenters are forced to learn more discriminative queries for effective instance separation. Second, our algorithm encourages both image (instance) representations and queries to be equivariant against geometric transformations, leading to more robust, instance-query matching. On top of four famous, query-based models ($i.e.,$ CondInst, SOLOv2, SOTR, and Mask2Former), our training algorithm provides significant performance gains ($e.g.,$ +1.6 - 3.2 AP) on COCO dataset. In addition, our algorithm promotes the performance of SOLOv2 by 2.7 AP, on LVISv1 dataset.
PDF Accepted to NeurIPS 2022; Code: https://github.com/JamesLiang819/Instance_Unique_Querying
点此查看论文截图
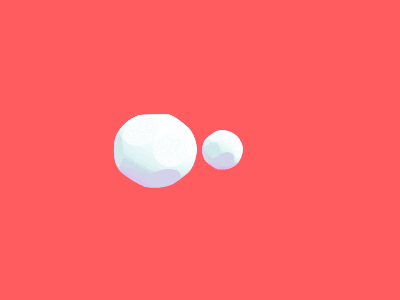
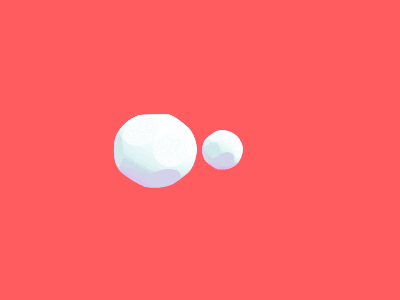
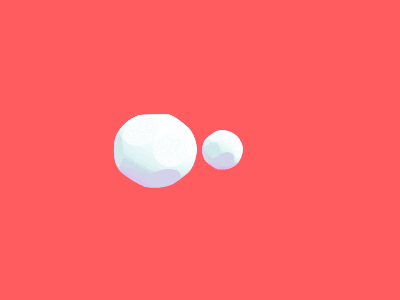
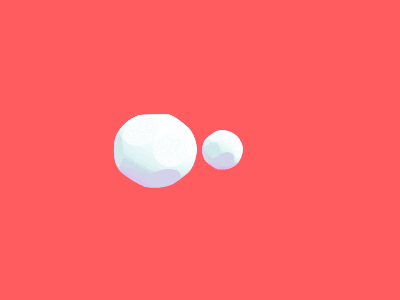
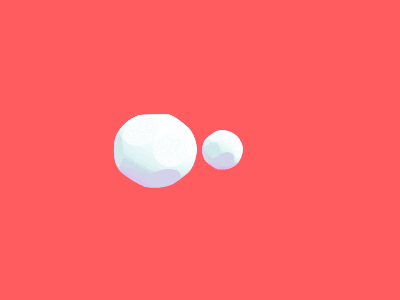
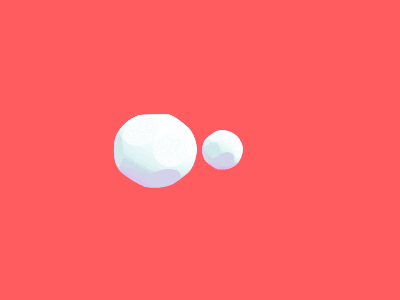
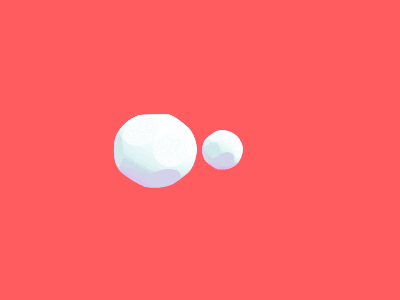
A Multi Camera Unsupervised Domain Adaptation Pipeline for Object Detection in Cultural Sites through Adversarial Learning and Self-Training
Authors:Giovanni Pasqualino, Antonino Furnari, Giovanni Maria Farinella
Object detection algorithms allow to enable many interesting applications which can be implemented in different devices, such as smartphones and wearable devices. In the context of a cultural site, implementing these algorithms in a wearable device, such as a pair of smart glasses, allow to enable the use of augmented reality (AR) to show extra information about the artworks and enrich the visitors’ experience during their tour. However, object detection algorithms require to be trained on many well annotated examples to achieve reasonable results. This brings a major limitation since the annotation process requires human supervision which makes it expensive in terms of time and costs. A possible solution to reduce these costs consist in exploiting tools to automatically generate synthetic labeled images from a 3D model of the site. However, models trained with synthetic data do not generalize on real images acquired in the target scenario in which they are supposed to be used. Furthermore, object detectors should be able to work with different wearable devices or different mobile devices, which makes generalization even harder. In this paper, we present a new dataset collected in a cultural site to study the problem of domain adaptation for object detection in the presence of multiple unlabeled target domains corresponding to different cameras and a labeled source domain obtained considering synthetic images for training purposes. We present a new domain adaptation method which outperforms current state-of-the-art approaches combining the benefits of aligning the domains at the feature and pixel level with a self-training process. We release the dataset at the following link https://iplab.dmi.unict.it/OBJ-MDA/ and the code of the proposed architecture at https://github.com/fpv-iplab/STMDA-RetinaNet.
PDF
点此查看论文截图
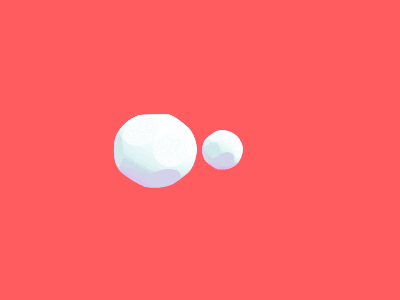
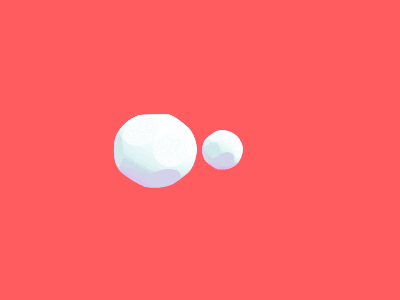
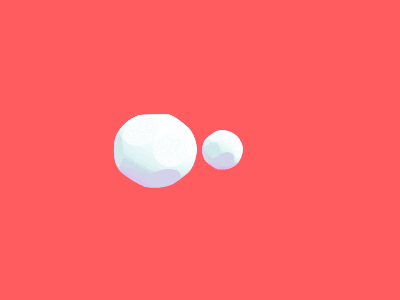
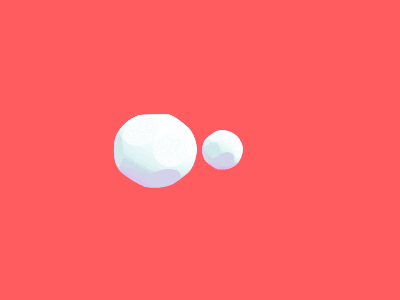
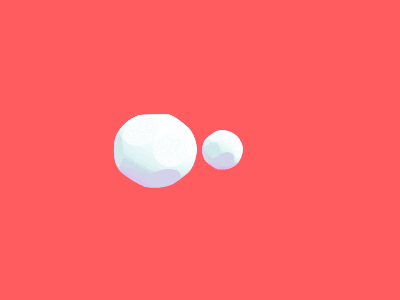
Towards Stable Co-saliency Detection and Object Co-segmentation
Authors:Bo Li, Lv Tang, Senyun Kuang, Mofei Song, Shouhong Ding
In this paper, we present a novel model for simultaneous stable co-saliency detection (CoSOD) and object co-segmentation (CoSEG). To detect co-saliency (segmentation) accurately, the core problem is to well model inter-image relations between an image group. Some methods design sophisticated modules, such as recurrent neural network (RNN), to address this problem. However, order-sensitive problem is the major drawback of RNN, which heavily affects the stability of proposed CoSOD (CoSEG) model. In this paper, inspired by RNN-based model, we first propose a multi-path stable recurrent unit (MSRU), containing dummy orders mechanisms (DOM) and recurrent unit (RU). Our proposed MSRU not only helps CoSOD (CoSEG) model captures robust inter-image relations, but also reduces order-sensitivity, resulting in a more stable inference and training process. { Moreover, we design a cross-order contrastive loss (COCL) that can further address order-sensitive problem by pulling close the feature embedding generated from different input orders.} We validate our model on five widely used CoSOD datasets (CoCA, CoSOD3k, Cosal2015, iCoseg and MSRC), and three widely used datasets (Internet, iCoseg and PASCAL-VOC) for object co-segmentation, the performance demonstrates the superiority of the proposed approach as compared to the state-of-the-art (SOTA) methods.
PDF
点此查看论文截图
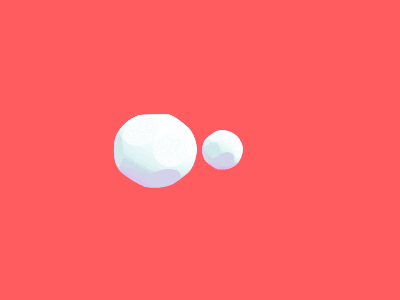
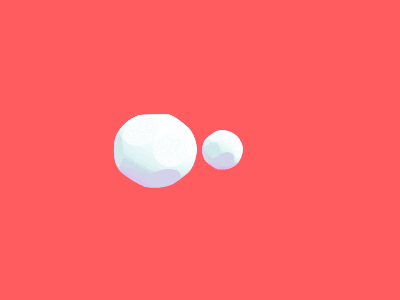
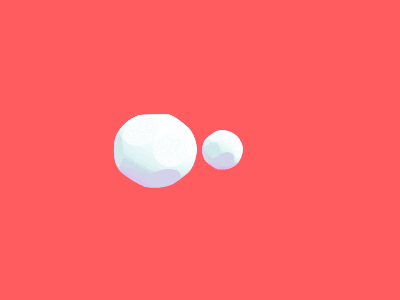
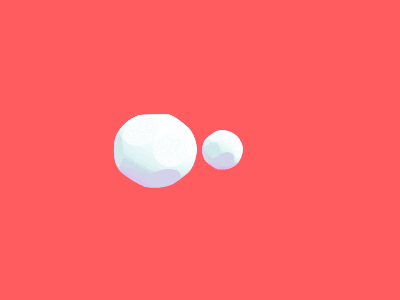
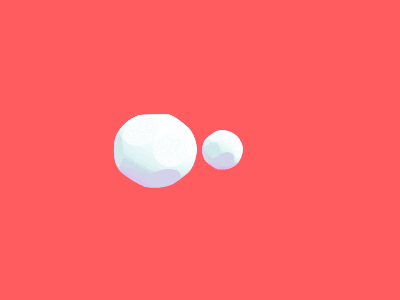
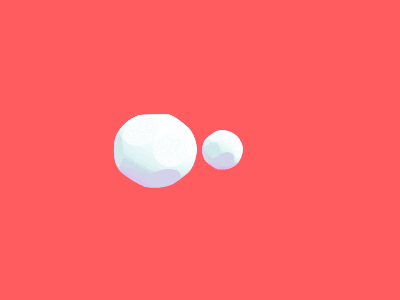
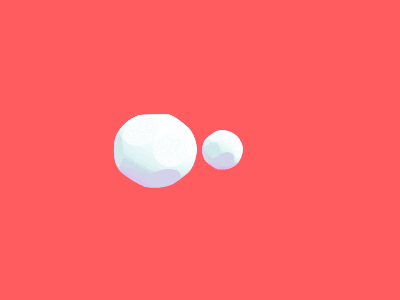
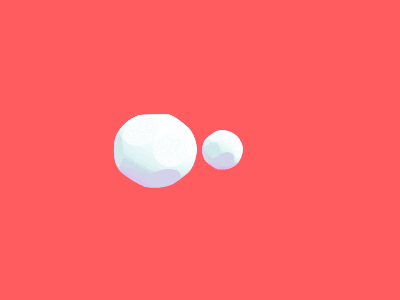