2022-10-01 更新
A singular Riemannian geometry approach to Deep Neural Networks I. Theoretical foundations
Authors:Alessandro Benfenati, Alessio Marta
Deep Neural Networks are widely used for solving complex problems in several scientific areas, such as speech recognition, machine translation, image analysis. The strategies employed to investigate their theoretical properties mainly rely on Euclidean geometry, but in the last years new approaches based on Riemannian geometry have been developed. Motivated by some open problems, we study a particular sequence of maps between manifolds, with the last manifold of the sequence equipped with a Riemannian metric. We investigate the structures induced trough pullbacks on the other manifolds of the sequence and on some related quotients. In particular, we show that the pullbacks of the final Riemannian metric to any manifolds of the sequence is a degenerate Riemannian metric inducing a structure of pseudometric space, we show that the Kolmogorov quotient of this pseudometric space yields a smooth manifold, which is the base space of a particular vertical bundle. We investigate the theoretical properties of the maps of such sequence, eventually we focus on the case of maps between manifolds implementing neural networks of practical interest and we present some applications of the geometric framework we introduced in the first part of the paper.
PDF
点此查看论文截图
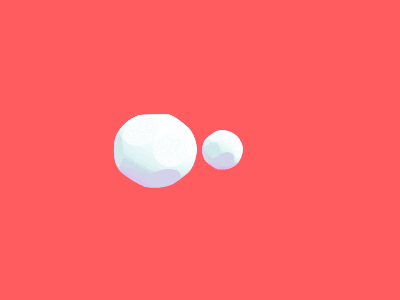
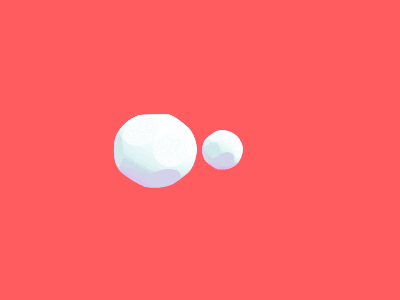
PerSign: Personalized Bangladeshi Sign Letters Synthesis
Authors:Mohammad Imrul Jubair, Ali Ahnaf, Tashfiq Nahiyan Khan, Ullash Bhattacharjee, Tanjila Joti
Bangladeshi Sign Language (BdSL) - like other sign languages - is tough to learn for general people, especially when it comes to expressing letters. In this poster, we propose PerSign, a system that can reproduce a person’s image by introducing sign gestures in it. We make this operation personalized, which means the generated image keeps the person’s initial image profile - face, skin tone, attire, background - unchanged while altering the hand, palm, and finger positions appropriately. We use an image-to-image translation technique and build a corresponding unique dataset to accomplish the task. We believe the translated image can reduce the communication gap between signers (person who uses sign language) and non-signers without having prior knowledge of BdSL.
PDF Accepted at ACM UIST 2022 (poster)
点此查看论文截图
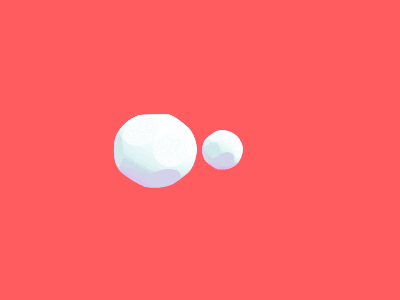
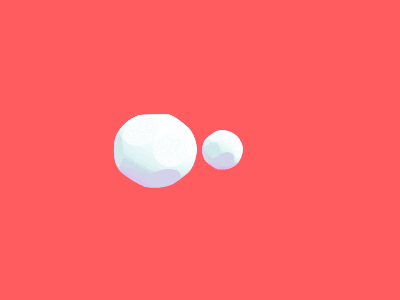
Translation, Scale and Rotation: Cross-Modal Alignment Meets RGB-Infrared Vehicle Detection
Authors:Maoxun Yuan, Yinyan Wang, Xingxing Wei
Integrating multispectral data in object detection, especially visible and infrared images, has received great attention in recent years. Since visible (RGB) and infrared (IR) images can provide complementary information to handle light variations, the paired images are used in many fields, such as multispectral pedestrian detection, RGB-IR crowd counting and RGB-IR salient object detection. Compared with natural RGB-IR images, we find detection in aerial RGB-IR images suffers from cross-modal weakly misalignment problems, which are manifested in the position, size and angle deviations of the same object. In this paper, we mainly address the challenge of cross-modal weakly misalignment in aerial RGB-IR images. Specifically, we firstly explain and analyze the cause of the weakly misalignment problem. Then, we propose a Translation-Scale-Rotation Alignment (TSRA) module to address the problem by calibrating the feature maps from these two modalities. The module predicts the deviation between two modality objects through an alignment process and utilizes Modality-Selection (MS) strategy to improve the performance of alignment. Finally, a two-stream feature alignment detector (TSFADet) based on the TSRA module is constructed for RGB-IR object detection in aerial images. With comprehensive experiments on the public DroneVehicle datasets, we verify that our method reduces the effect of the cross-modal misalignment and achieve robust detection results.
PDF
点此查看论文截图
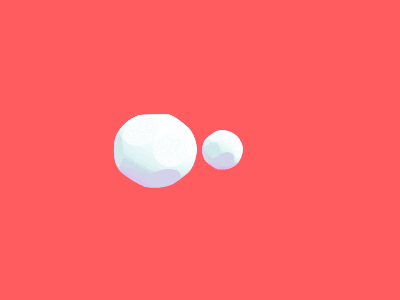
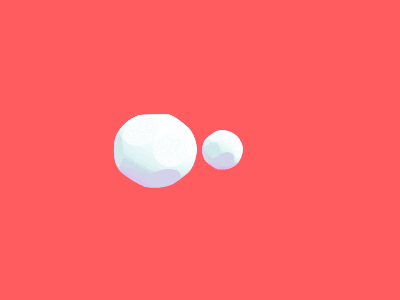
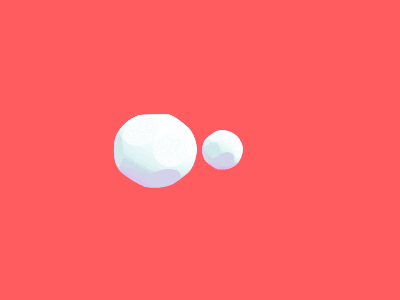
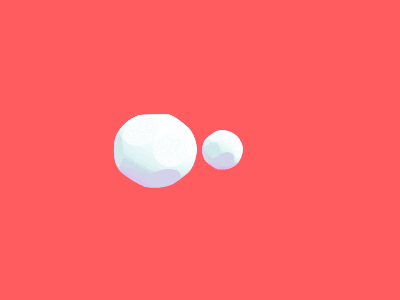
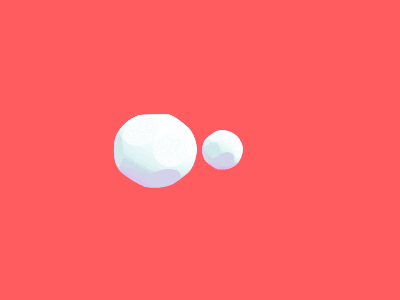
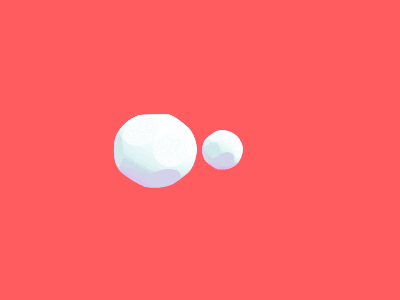
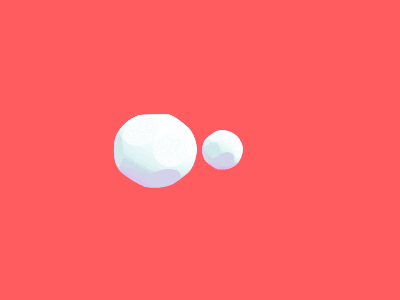
Image-to-Image Translation for Autonomous Driving from Coarsely-Aligned Image Pairs
Authors:Youya Xia, Josephine Monica, Wei-Lun Chao, Bharath Hariharan, Kilian Q Weinberger, Mark Campbell
A self-driving car must be able to reliably handle adverse weather conditions (e.g., snowy) to operate safely. In this paper, we investigate the idea of turning sensor inputs (i.e., images) captured in an adverse condition into a benign one (i.e., sunny), upon which the downstream tasks (e.g., semantic segmentation) can attain high accuracy. Prior work primarily formulates this as an unpaired image-to-image translation problem due to the lack of paired images captured under the exact same camera poses and semantic layouts. While perfectly-aligned images are not available, one can easily obtain coarsely-paired images. For instance, many people drive the same routes daily in both good and adverse weather; thus, images captured at close-by GPS locations can form a pair. Though data from repeated traversals are unlikely to capture the same foreground objects, we posit that they provide rich contextual information to supervise the image translation model. To this end, we propose a novel training objective leveraging coarsely-aligned image pairs. We show that our coarsely-aligned training scheme leads to a better image translation quality and improved downstream tasks, such as semantic segmentation, monocular depth estimation, and visual localization.
PDF Submitted to the International Conference on Robotics and Automation (ICRA) 2023
点此查看论文截图
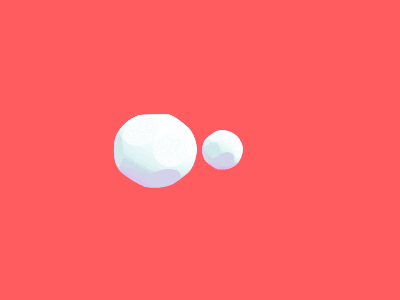
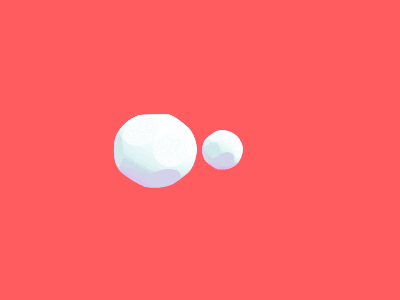
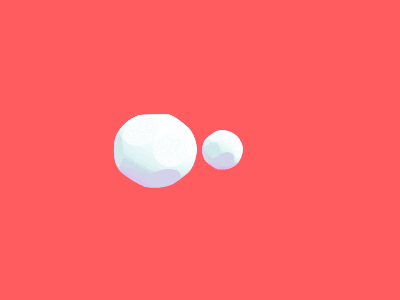
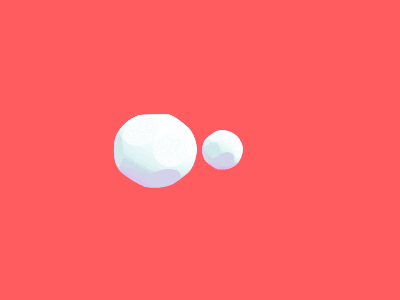
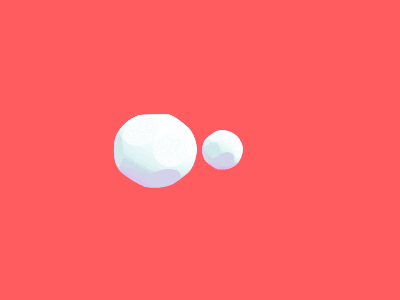
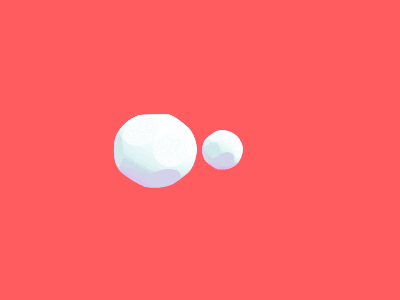
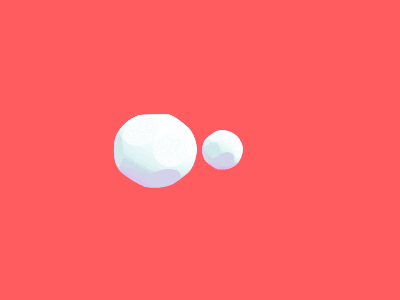
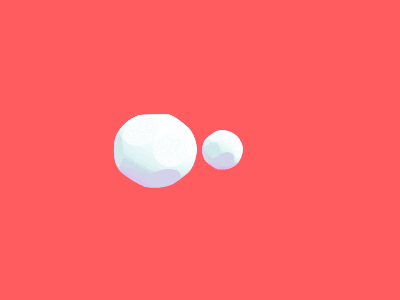
Enhancing Data Diversity for Self-training Based Unsupervised Cross-modality Vestibular Schwannoma and Cochlea Segmentation
Authors:Han Liu, Yubo Fan, Benoit M. Dawant
Automatic segmentation of vestibular schwannoma (VS) and the cochlea from magnetic resonance imaging (MRI) can facilitate VS treatment planning. Unsupervised segmentation methods have shown promising results without requiring the time-consuming and laborious manual labeling process. In this paper, we present an approach for VS and cochlea segmentation in an unsupervised domain adaptation setting. Specifically, we first develop a cross-site cross-modality unpaired image translation strategy to enrich the diversity of the synthesized data. Then, we devise a rule-based offline augmentation technique to further minimize the domain gap. Lastly, we adopt a self-configuring segmentation framework empowered by self-training to obtain the final results. On the CrossMoDA 2022 validation leaderboard, our method has achieved competitive VS and cochlea segmentation performance with mean dice scores of 0.8178 $\pm$ 0.0803 and 0.8433 $\pm$ 0.0293, respectively.
PDF CrossMoDA 2022
点此查看论文截图
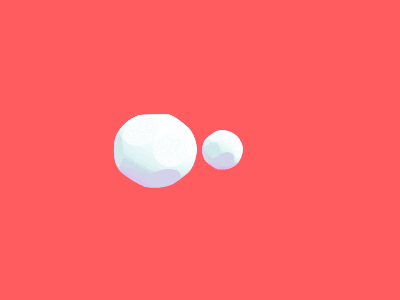
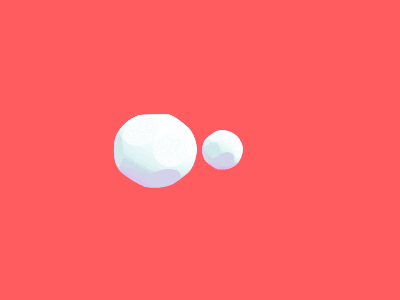
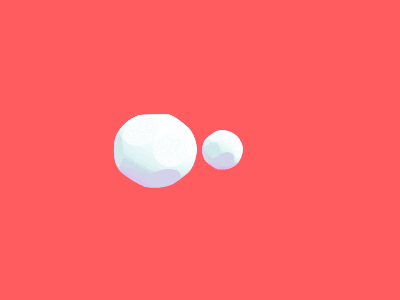
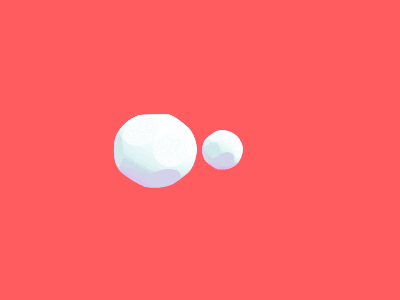
Unsupervised Domain Adaptation with Histogram-gated Image Translation for Delayered IC Image Analysis
Authors:Yee-Yang Tee, Deruo Cheng, Chye-Soon Chee, Tong Lin, Yiqiong Shi, Bah-Hwee Gwee
Deep learning has achieved great success in the challenging circuit annotation task by employing Convolutional Neural Networks (CNN) for the segmentation of circuit structures. The deep learning approaches require a large amount of manually annotated training data to achieve a good performance, which could cause a degradation in performance if a deep learning model trained on a given dataset is applied to a different dataset. This is commonly known as the domain shift problem for circuit annotation, which stems from the possibly large variations in distribution across different image datasets. The different image datasets could be obtained from different devices or different layers within a single device. To address the domain shift problem, we propose Histogram-gated Image Translation (HGIT), an unsupervised domain adaptation framework which transforms images from a given source dataset to the domain of a target dataset, and utilize the transformed images for training a segmentation network. Specifically, our HGIT performs generative adversarial network (GAN)-based image translation and utilizes histogram statistics for data curation. Experiments were conducted on a single labeled source dataset adapted to three different target datasets (without labels for training) and the segmentation performance was evaluated for each target dataset. We have demonstrated that our method achieves the best performance compared to the reported domain adaptation techniques, and is also reasonably close to the fully supervised benchmark.
PDF 7 pages, 4 figures, To be presented at IEEE PAINE 2022 (oral)
点此查看论文截图
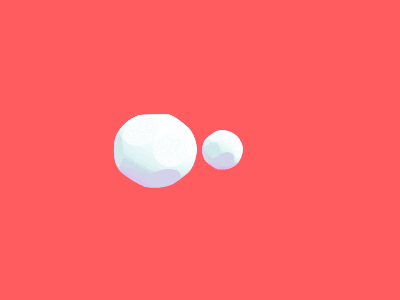
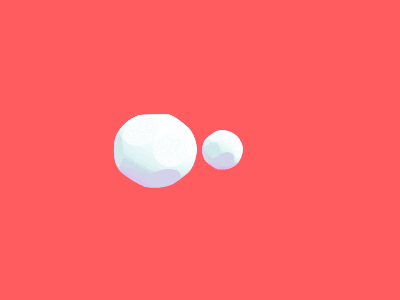
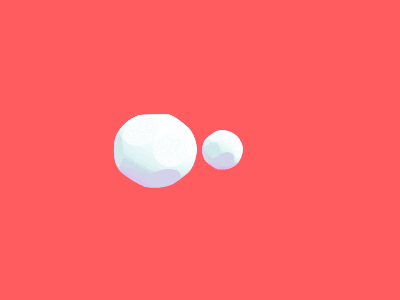
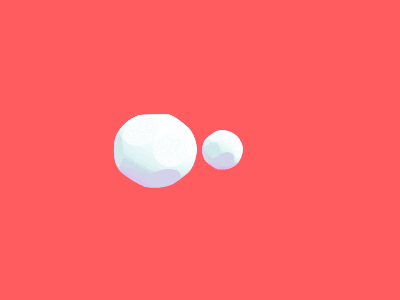
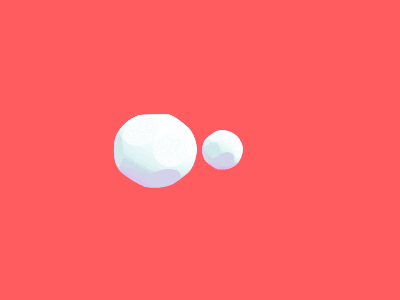