2022-09-29 更新
Contrastive Learning for Unsupervised Domain Adaptation of Time Series
Authors:Yilmazcan Ozyurt, Stefan Feuerriegel, Ce Zhang
Unsupervised domain adaptation (UDA) aims at learning a machine learning model using a labeled source domain that performs well on a similar yet different, unlabeled target domain. UDA is important in many applications such as medicine, where it is used to adapt risk scores across different patient cohorts. In this paper, we develop a novel framework for UDA of time series data, called CLUDA. Specifically, we propose a contrastive learning framework to learn contextual representations in multivariate time series, so that these preserve label information for the prediction task. In our framework, we further capture the variation in the contextual representations between source and target domain via a custom nearest-neighbor contrastive learning. To the best of our knowledge, ours is the first framework to learn domain-invariant, contextual representation for UDA of time series data. We evaluate our framework using a wide range of time series datasets to demonstrate its effectiveness and show that it achieves state-of-the-art performance for time series UDA.
PDF
点此查看论文截图
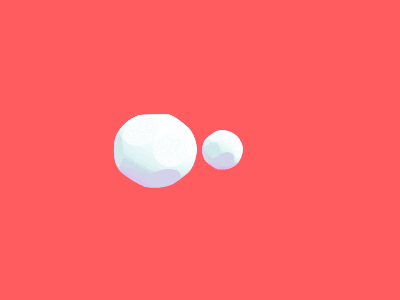
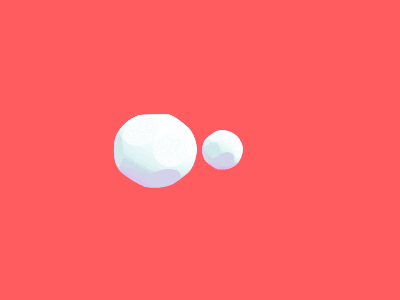
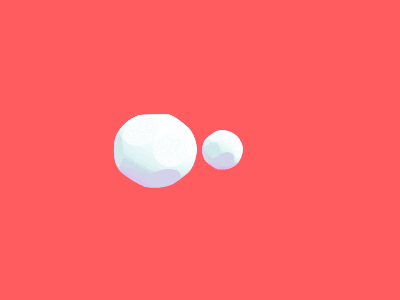
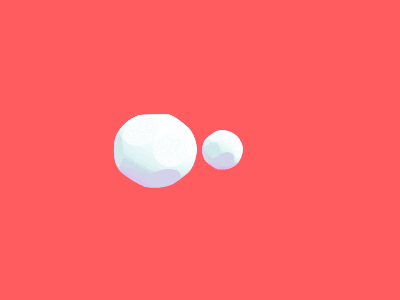
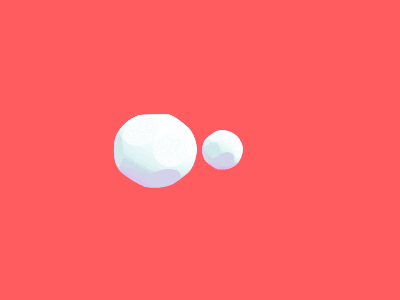
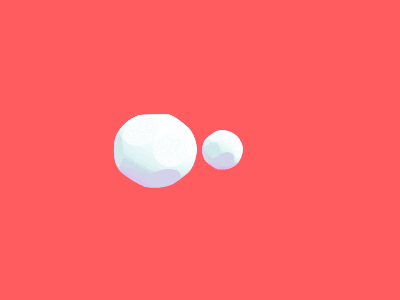
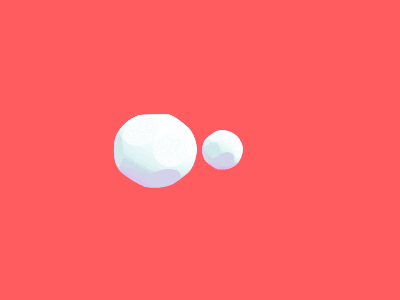
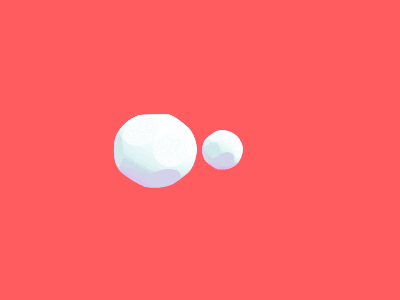
Improving Multilingual Neural Machine Translation System for Indic Languages
Authors:Sudhansu Bala Das, Atharv Biradar, Tapas Kumar Mishra, Bidyut Kumar Patra
Machine Translation System (MTS) serves as an effective tool for communication by translating text or speech from one language to another language. The need of an efficient translation system becomes obvious in a large multilingual environment like India, where English and a set of Indian Languages (ILs) are officially used. In contrast with English, ILs are still entreated as low-resource languages due to unavailability of corpora. In order to address such asymmetric nature, multilingual neural machine translation (MNMT) system evolves as an ideal approach in this direction. In this paper, we propose a MNMT system to address the issues related to low-resource language translation. Our model comprises of two MNMT systems i.e. for English-Indic (one-to-many) and the other for Indic-English (many-to-one) with a shared encoder-decoder containing 15 language pairs (30 translation directions). Since most of IL pairs have scanty amount of parallel corpora, not sufficient for training any machine translation model. We explore various augmentation strategies to improve overall translation quality through the proposed model. A state-of-the-art transformer architecture is used to realize the proposed model. Trials over a good amount of data reveal its superiority over the conventional models. In addition, the paper addresses the use of language relationships (in terms of dialect, script, etc.), particularly about the role of high-resource languages of the same family in boosting the performance of low-resource languages. Moreover, the experimental results also show the advantage of backtranslation and domain adaptation for ILs to enhance the translation quality of both source and target languages. Using all these key approaches, our proposed model emerges to be more efficient than the baseline model in terms of evaluation metrics i.e BLEU (BiLingual Evaluation Understudy) score for a set of ILs.
PDF 26 pages, 10 figures, 7 tables
点此查看论文截图
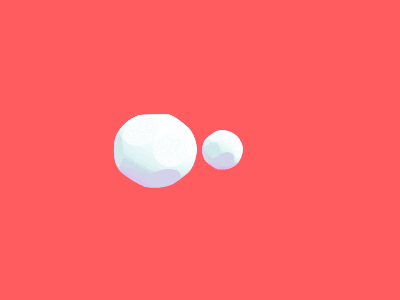
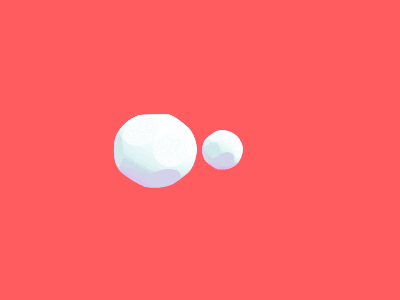
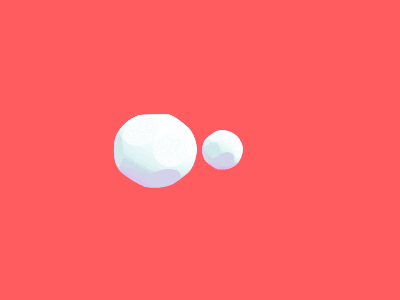
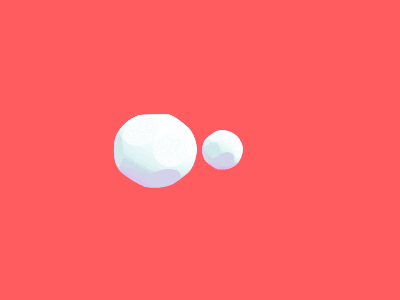