2022-09-17 更新
AvatarGen: a 3D Generative Model for Animatable Human Avatars
Authors:Jianfeng Zhang, Zihang Jiang, Dingdong Yang, Hongyi Xu, Yichun Shi, Guoxian Song, Zhongcong Xu, Xinchao Wang, Jiashi Feng
Unsupervised generation of clothed virtual humans with various appearance and animatable poses is important for creating 3D human avatars and other AR/VR applications. Existing methods are either limited to rigid object modeling, or not generative and thus unable to synthesize high-quality virtual humans and animate them. In this work, we propose AvatarGen, the first method that enables not only non-rigid human generation with diverse appearance but also full control over poses and viewpoints, while only requiring 2D images for training. Specifically, it extends the recent 3D GANs to clothed human generation by utilizing a coarse human body model as a proxy to warp the observation space into a standard avatar under a canonical space. To model non-rigid dynamics, it introduces a deformation network to learn pose-dependent deformations in the canonical space. To improve geometry quality of the generated human avatars, it leverages signed distance field as geometric representation, which allows more direct regularization from the body model on the geometry learning. Benefiting from these designs, our method can generate animatable human avatars with high-quality appearance and geometry modeling, significantly outperforming previous 3D GANs. Furthermore, it is competent for many applications, e.g., single-view reconstruction, reanimation, and text-guided synthesis. Code and pre-trained model will be available.
PDF First two authors contributed equally. Code will be available at https://github.com/jfzhang95/AvatarGen
点此查看论文截图
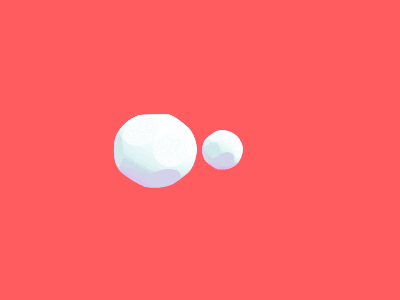
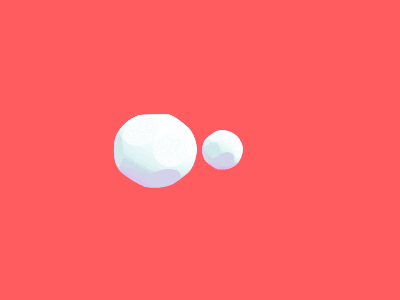
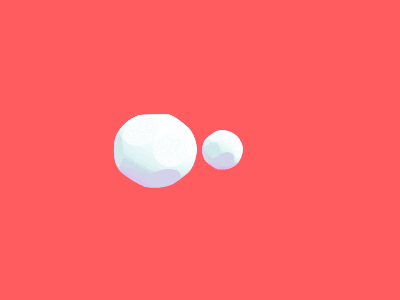
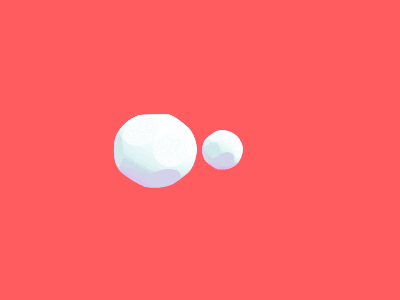
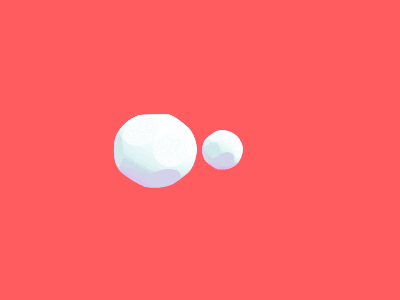
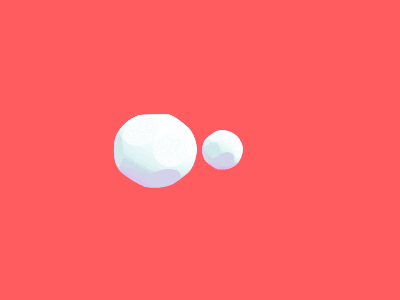
Dual-Space NeRF: Learning Animatable Avatars and Scene Lighting in Separate Spaces
Authors:Yihao Zhi, Shenhan Qian, Xinhao Yan, Shenghua Gao
Modeling the human body in a canonical space is a common practice for capturing and animation. But when involving the neural radiance field (NeRF), learning a static NeRF in the canonical space is not enough because the lighting of the body changes when the person moves even though the scene lighting is constant. Previous methods alleviate the inconsistency of lighting by learning a per-frame embedding, but this operation does not generalize to unseen poses. Given that the lighting condition is static in the world space while the human body is consistent in the canonical space, we propose a dual-space NeRF that models the scene lighting and the human body with two MLPs in two separate spaces. To bridge these two spaces, previous methods mostly rely on the linear blend skinning (LBS) algorithm. However, the blending weights for LBS of a dynamic neural field are intractable and thus are usually memorized with another MLP, which does not generalize to novel poses. Although it is possible to borrow the blending weights of a parametric mesh such as SMPL, the interpolation operation introduces more artifacts. In this paper, we propose to use the barycentric mapping, which can directly generalize to unseen poses and surprisingly achieves superior results than LBS with neural blending weights. Quantitative and qualitative results on the Human3.6M and the ZJU-MoCap datasets show the effectiveness of our method.
PDF Accepted by 3DV 2022
点此查看论文截图
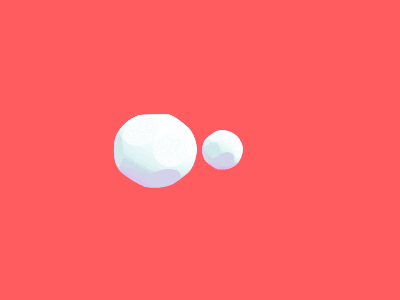
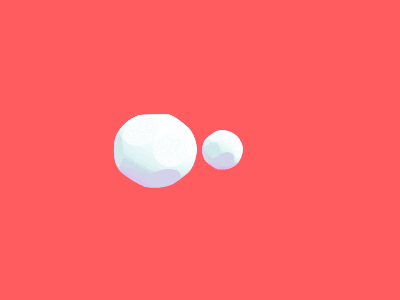
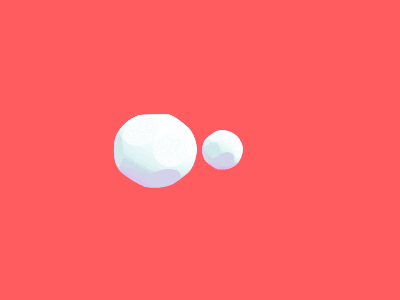
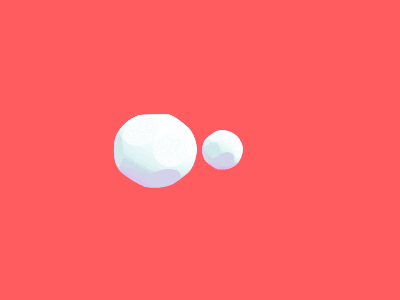
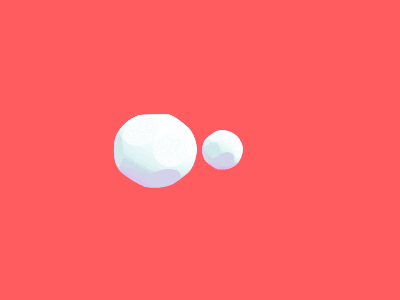
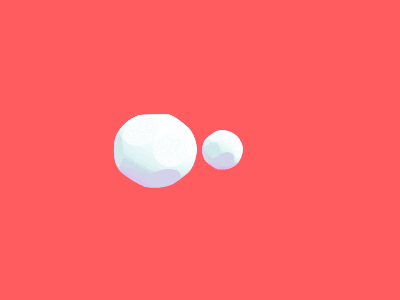
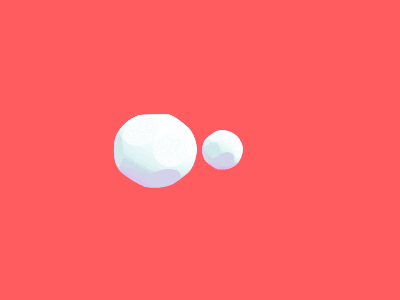
Attention-aware Resource Allocation and QoE Analysis for Metaverse xURLLC Services
Authors:Hongyang Du, Jiazhen Liu, Dusit Niyato, Jiawen Kang, Zehui Xiong, Junshan Zhang, Dong In Kim
Metaverse encapsulates our expectations of the next-generation Internet, while bringing new key performance indicators (KPIs). Although conventional ultra-reliable and low-latency communications (URLLC) can satisfy objective KPIs, it is difficult to provide a personalized immersive experience that is a distinctive feature of the Metaverse. Since the quality of experience (QoE) can be regarded as a comprehensive KPI, the URLLC is evolved towards the next generation URLLC (xURLLC) with a personalized resource allocation scheme to achieve higher QoE. To deploy Metaverse xURLLC services, we study the interaction between the Metaverse service provider (MSP) and the network infrastructure provider (InP), and provide an optimal contract design framework. Specifically, the utility of the MSP, defined as a function of Metaverse users’ QoE, is to be maximized, while ensuring the incentives of the InP. To model the QoE mathematically, we propose a novel metric named Meta-Immersion that incorporates both the objective KPIs and subjective feelings of Metaverse users. Furthermore, we develop an attention-aware rendering capacity allocation scheme to improve QoE in xURLLC. Using a user-object-attention level dataset, we validate that the xURLLC can achieve an average of 20.1% QoE improvement compared to the conventional URLLC with a uniform resource allocation scheme.
PDF
点此查看论文截图
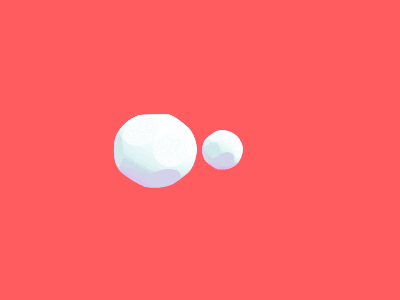
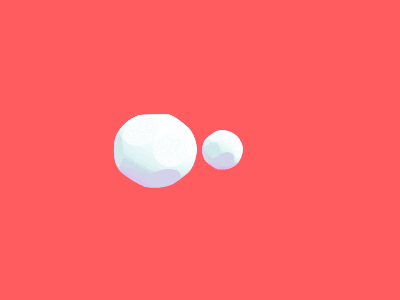
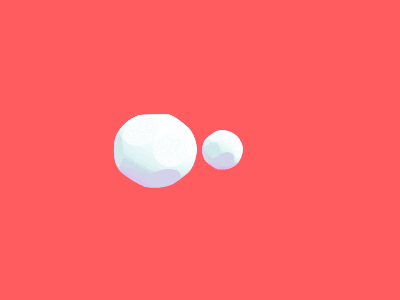
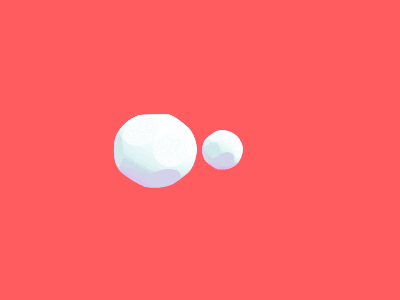
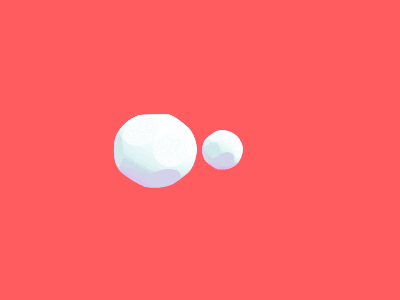
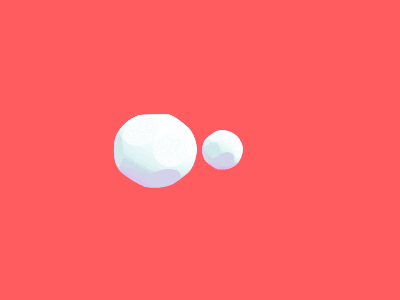
Real Time Egocentric Segmentation for Video-self Avatar in Mixed Reality
Authors:Ester Gonzalez-Sosa, Andrija Gajic, Diego Gonzalez-Morin, Guillermo Robledo, Pablo Perez, Alvaro Villegas
In this work we present our real-time egocentric body segmentation algorithm. Our algorithm achieves a frame rate of 66 fps for an input resolution of 640x480, thanks to our shallow network inspired in Thundernet’s architecture. Besides, we put a strong emphasis on the variability of the training data. More concretely, we describe the creation process of our Egocentric Bodies (EgoBodies) dataset, composed of almost 10,000 images from three datasets, created both from synthetic methods and real capturing. We conduct experiments to understand the contribution of the individual datasets; compare Thundernet model trained with EgoBodies with simpler and more complex previous approaches and discuss their corresponding performance in a real-life setup in terms of segmentation quality and inference times. The described trained semantic segmentation algorithm is already integrated in an end-to-end system for Mixed Reality (MR), making it possible for users to see his/her own body while being immersed in a MR scene.
PDF 9 pages, 9 figures
点此查看论文截图
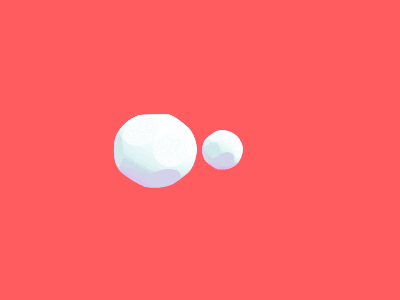
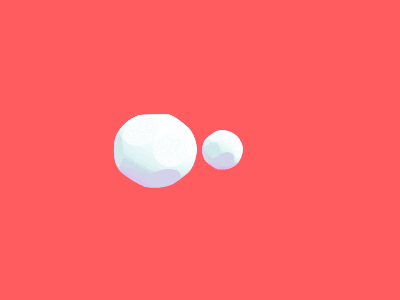
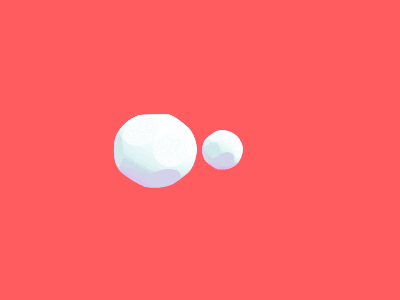
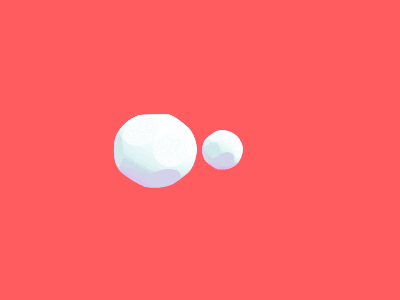
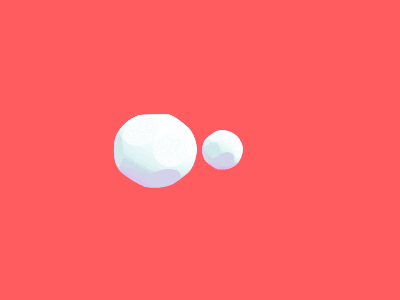
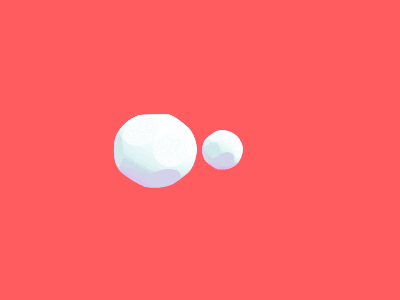
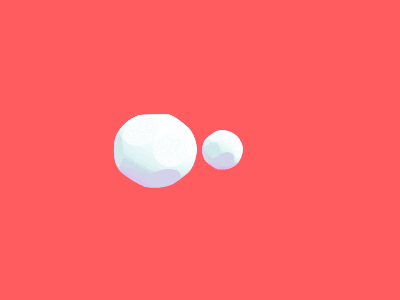
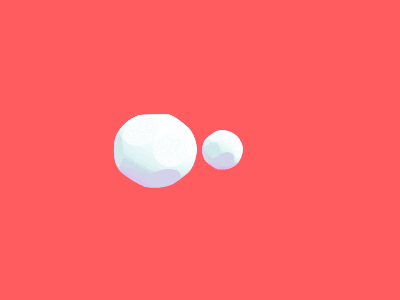
Motion Projection Consistency Based 3D Human Pose Estimation with Virtual Bones from Monocular Videos
Authors:Guangming Wang, Honghao Zeng, Ziliang Wang, Zhe Liu, Hesheng Wang
Real-time 3D human pose estimation is crucial for human-computer interaction. It is cheap and practical to estimate 3D human pose only from monocular video. However, recent bone splicing based 3D human pose estimation method brings about the problem of cumulative error. In this paper, the concept of virtual bones is proposed to solve such a challenge. The virtual bones are imaginary bones between non-adjacent joints. They do not exist in reality, but they bring new loop constraints for the estimation of 3D human joints. The proposed network in this paper predicts real bones and virtual bones, simultaneously. The final length of real bones is constrained and learned by the loop constructed by the predicted real bones and virtual bones. Besides, the motion constraints of joints in consecutive frames are considered. The consistency between the 2D projected position displacement predicted by the network and the captured real 2D displacement by the camera is proposed as a new projection consistency loss for the learning of 3D human pose. The experiments on the Human3.6M dataset demonstrate the good performance of the proposed method. Ablation studies demonstrate the effectiveness of the proposed inter-frame projection consistency constraints and intra-frame loop constraints.
PDF 10 pages, 7 figures. Accepted by TCDS 2022
点此查看论文截图
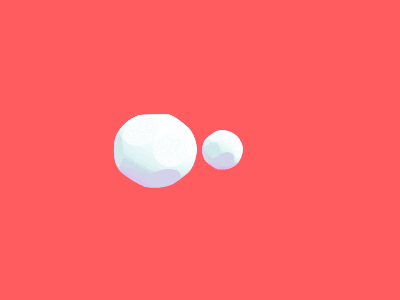
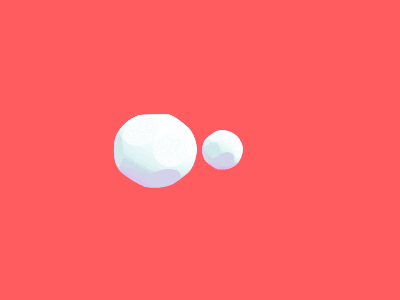
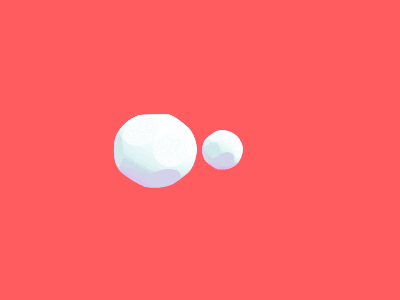
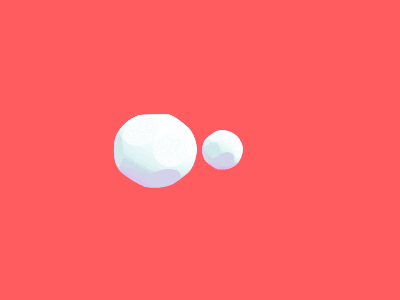
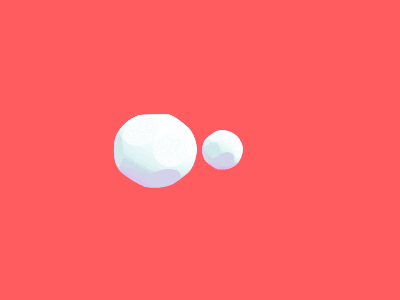
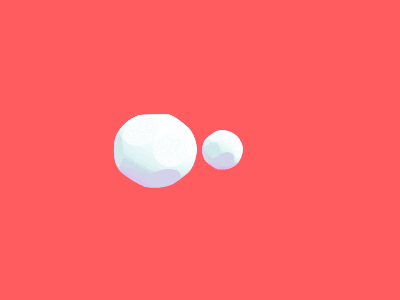
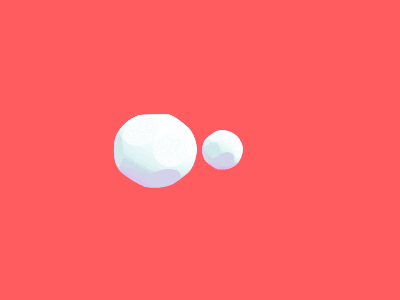
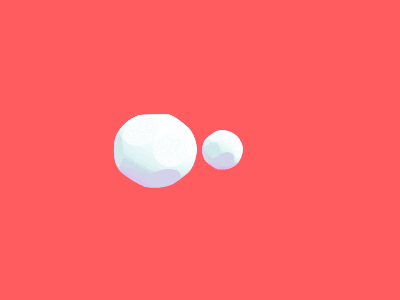
MetaFi: Device-Free Pose Estimation via Commodity WiFi for Metaverse Avatar Simulation
Authors:Jianfei Yang, Yunjiao Zhou, He Huang, Han Zou, Lihua Xie
Avatar refers to a representative of a physical user in the virtual world that can engage in different activities and interact with other objects in metaverse. Simulating the avatar requires accurate human pose estimation. Though camera-based solutions yield remarkable performance, they encounter the privacy issue and degraded performance caused by varying illumination, especially in smart home. In this paper, we propose a WiFi-based IoT-enabled human pose estimation scheme for metaverse avatar simulation, namely MetaFi. Specifically, a deep neural network is designed with customized convolutional layers and residual blocks to map the channel state information to human pose landmarks. It is enforced to learn the annotations from the accurate computer vision model, thus achieving cross-modal supervision. WiFi is ubiquitous and robust to illumination, making it a feasible solution for avatar applications in smart home. The experiments are conducted in the real world, and the results show that the MetaFi achieves very high performance with a PCK@50 of 95.23%.
PDF 6 pages, 3 figures, 3 tables
点此查看论文截图
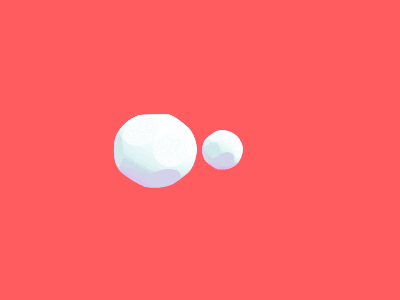
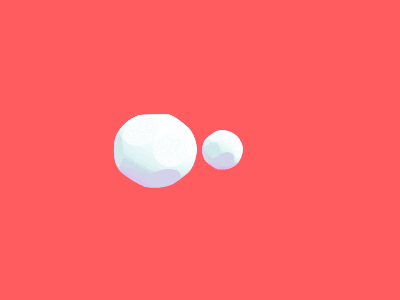
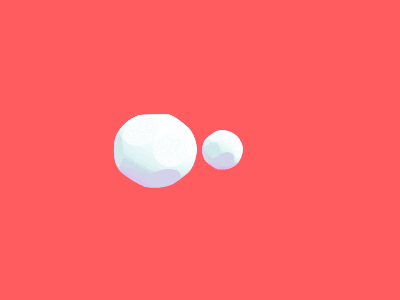
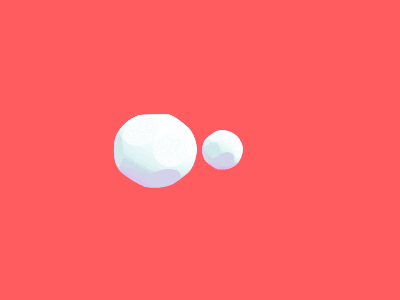
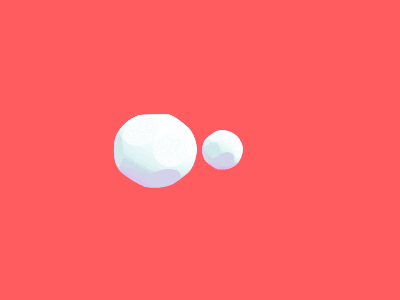
MegaPortraits: One-shot Megapixel Neural Head Avatars
Authors:Nikita Drobyshev, Jenya Chelishev, Taras Khakhulin, Aleksei Ivakhnenko, Victor Lempitsky, Egor Zakharov
In this work, we advance the neural head avatar technology to the megapixel resolution while focusing on the particularly challenging task of cross-driving synthesis, i.e., when the appearance of the driving image is substantially different from the animated source image. We propose a set of new neural architectures and training methods that can leverage both medium-resolution video data and high-resolution image data to achieve the desired levels of rendered image quality and generalization to novel views and motion. We demonstrate that suggested architectures and methods produce convincing high-resolution neural avatars, outperforming the competitors in the cross-driving scenario. Lastly, we show how a trained high-resolution neural avatar model can be distilled into a lightweight student model which runs in real-time and locks the identities of neural avatars to several dozens of pre-defined source images. Real-time operation and identity lock are essential for many practical applications head avatar systems.
PDF
点此查看论文截图
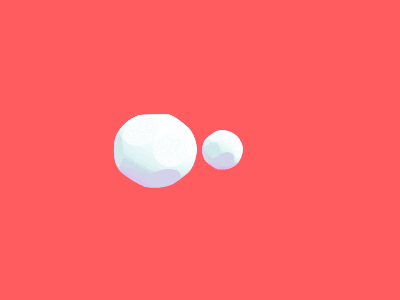
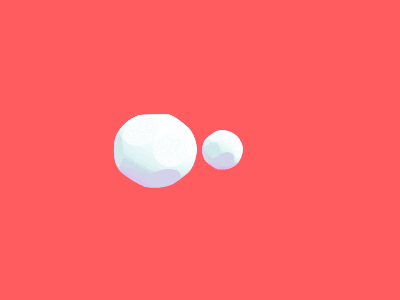
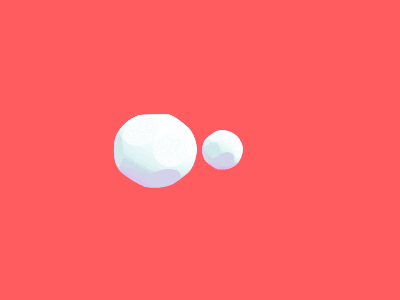
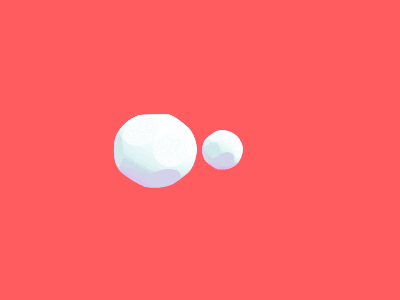
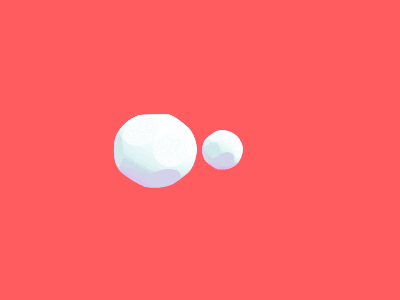
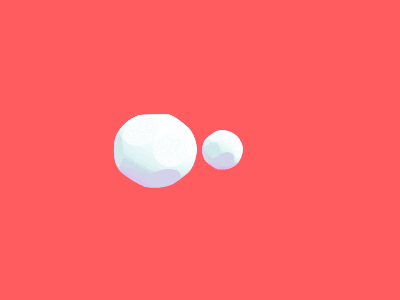
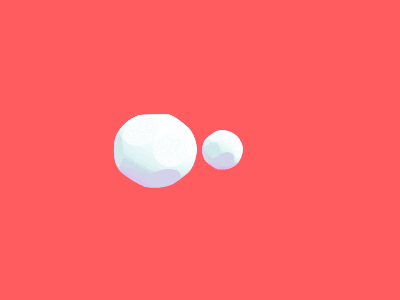
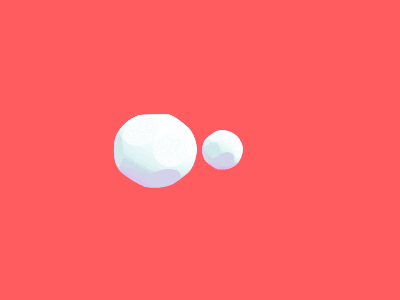
Efficient Customer Service Combining Human Operators and Virtual Agents
Authors:Yaniv Oshrat, Yonatan Aumann, Tal Hollander, Oleg Maksimov, Anita Ostroumov, Natali Shechtman, Sarit Kraus
The prospect of combining human operators and virtual agents (bots) into an effective hybrid system that provides proper customer service to clients is promising yet challenging. The hybrid system decreases the customers’ frustration when bots are unable to provide appropriate service and increases their satisfaction when they prefer to interact with human operators. Furthermore, we show that it is possible to decrease the cost and efforts of building and maintaining such virtual agents by enabling the virtual agent to incrementally learn from the human operators. We employ queuing theory to identify the key parameters that govern the behavior and efficiency of such hybrid systems and determine the main parameters that should be optimized in order to improve the service. We formally prove, and demonstrate in extensive simulations and in a user study, that with the proper choice of parameters, such hybrid systems are able to increase the number of served clients while simultaneously decreasing their expected waiting time and increasing satisfaction.
PDF
点此查看论文截图
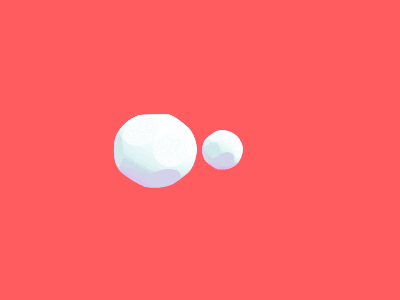
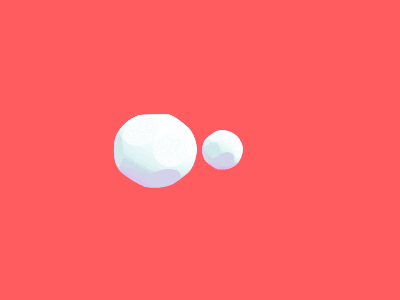
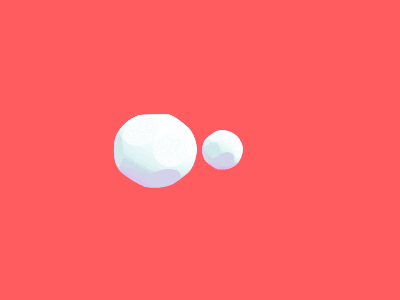
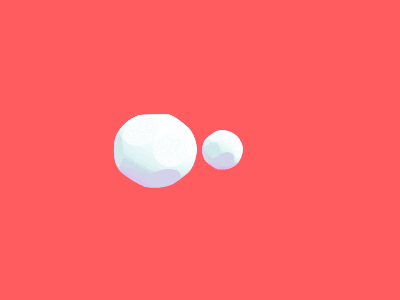
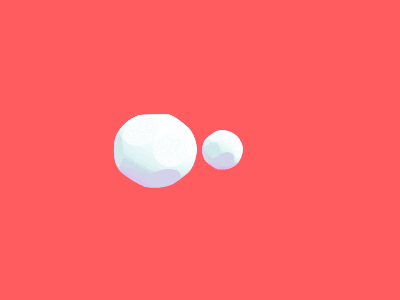
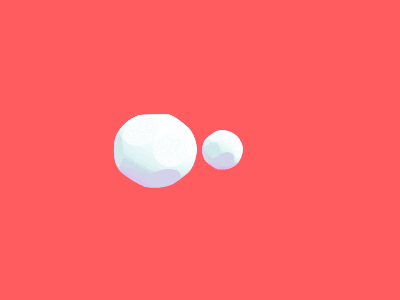
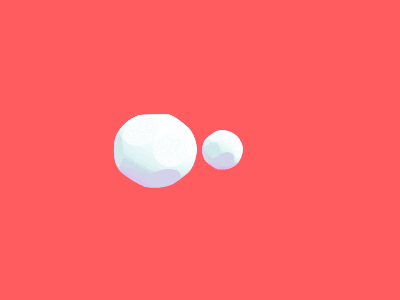
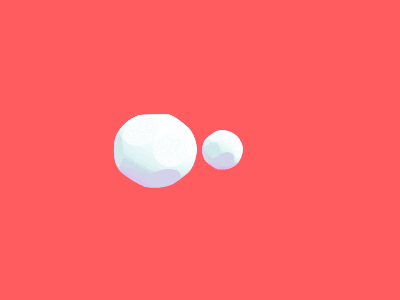
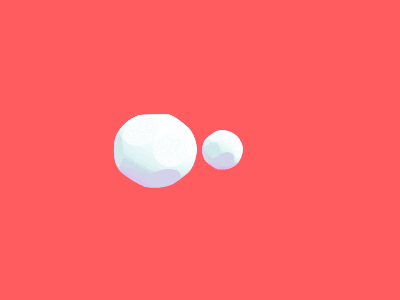