2022-09-15 更新
High-resolution semantically-consistent image-to-image translation
Authors:Mikhail Sokolov, Christopher Henry, Joni Storie, Christopher Storie, Victor Alhassan, Mathieu Turgeon-Pelchat
Deep learning has become one of remote sensing scientists’ most efficient computer vision tools in recent years. However, the lack of training labels for the remote sensing datasets means that scientists need to solve the domain adaptation problem to narrow the discrepancy between satellite image datasets. As a result, image segmentation models that are then trained, could better generalize and use an existing set of labels instead of requiring new ones. This work proposes an unsupervised domain adaptation model that preserves semantic consistency and per-pixel quality for the images during the style-transferring phase. This paper’s major contribution is proposing the improved architecture of the SemI2I model, which significantly boosts the proposed model’s performance and makes it competitive with the state-of-the-art CyCADA model. A second contribution is testing the CyCADA model on the remote sensing multi-band datasets such as WorldView-2 and SPOT-6. The proposed model preserves semantic consistency and per-pixel quality for the images during the style-transferring phase. Thus, the semantic segmentation model, trained on the adapted images, shows substantial performance gain compared to the SemI2I model and reaches similar results as the state-of-the-art CyCADA model. The future development of the proposed method could include ecological domain transfer, {\em a priori} evaluation of dataset quality in terms of data distribution, or exploration of the inner architecture of the domain adaptation model.
PDF 25 pages, 7 figures
点此查看论文截图
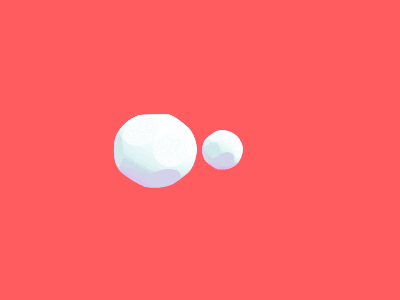