2022-09-14 更新
TPSNet: Reverse Thinking of Thin Plate Splines for Arbitrary Shape Scene Text Representation
Authors:Wei Wang, Yu Zhou, Jiahao Lv, Dayan Wu, Guoqing Zhao, Ning Jiang, Weiping Wang
The research focus of scene text detection and recognition has shifted to arbitrary shape text in recent years, where the text shape representation is a fundamental problem. An ideal representation should be compact, complete, efficient, and reusable for subsequent recognition in our opinion. However, previous representations have flaws in one or more aspects. Thin-Plate-Spline (TPS) transformation has achieved great success in scene text recognition. Inspired by this, we reversely think of its usage and sophisticatedly take TPS as an exquisite representation for arbitrary shape text representation. The TPS representation is compact, complete, and efficient. With the predicted TPS parameters, the detected text region can be directly rectified to a near-horizontal one to assist the subsequent recognition. To further exploit the potential of the TPS representation, the Border Alignment Loss is proposed. Based on these designs, we implement the text detector TPSNet, which can be extended to a text spotter conveniently. Extensive evaluation and ablation of several public benchmarks demonstrate the effectiveness and superiority of the proposed method for text representation and spotting. Particularly, TPSNet achieves the detection F-Measure improvement of 4.4\% (78.4\% vs. 74.0\%) on Art dataset and the end-to-end spotting F-Measure improvement of 5.0\% (78.5\% vs. 73.5\%) on Total-Text, which are large margins with no bells and whistles.
PDF
点此查看论文截图
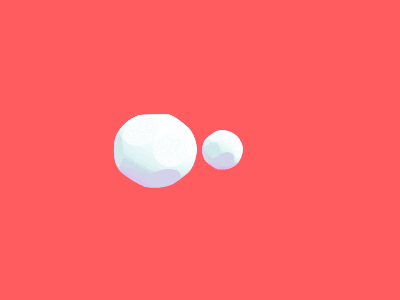
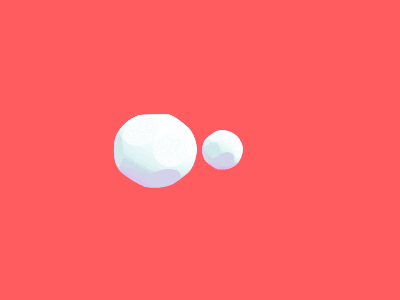
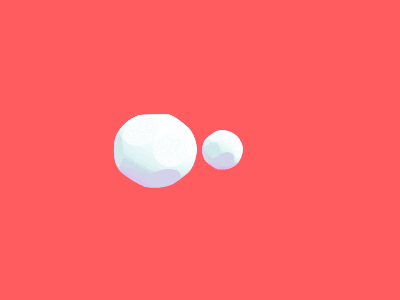
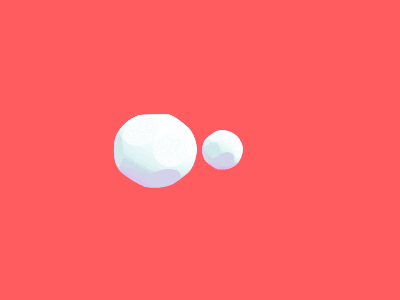
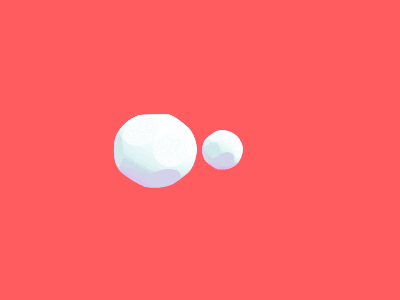
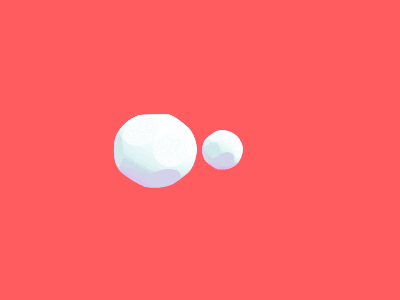
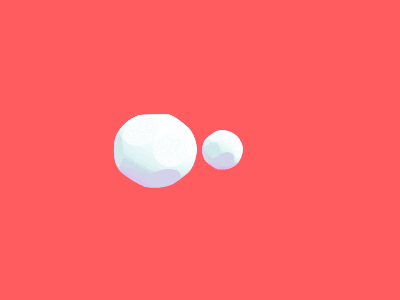
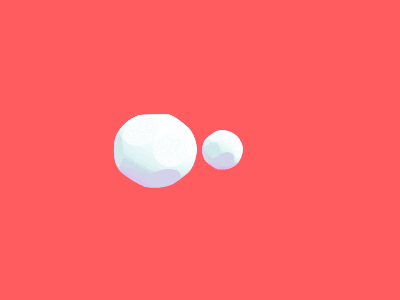
PreSTU: Pre-Training for Scene-Text Understanding
Authors:Jihyung Kil, Soravit Changpinyo, Xi Chen, Hexiang Hu, Sebastian Goodman, Wei-Lun Chao, Radu Soricut
The ability to read and reason about texts in an image is often lacking in vision-and-language (V&L) models. How can we learn V&L models that exhibit strong scene-text understanding (STU)? In this paper, we propose PreSTU, a simple pre-training recipe specifically designed for scene-text understanding. PreSTU combines a simple OCR-aware pre-training objective with a large-scale image-text dataset with off-the-shelf OCR signals. We empirically demonstrate the superiority of this pre-training objective on TextVQA, TextCaps, ST-VQA, and VizWiz-VQA. We also study which factors affect STU performance, where we highlight the importance of image resolution and dataset scale during pre-training.
PDF
点此查看论文截图
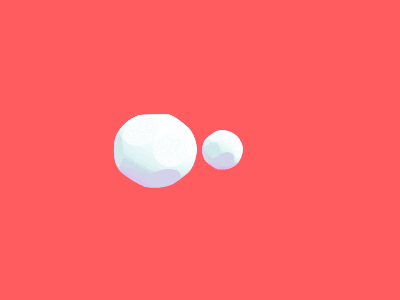
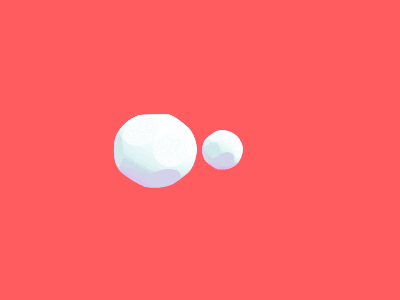
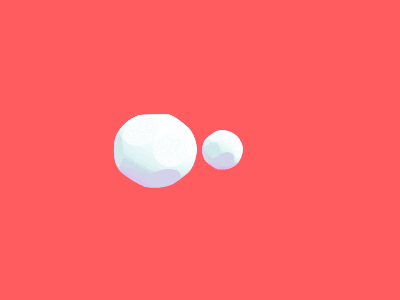
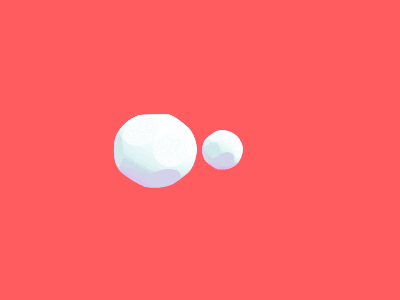
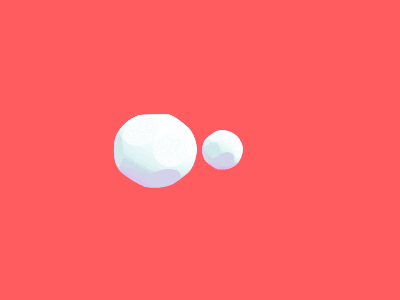
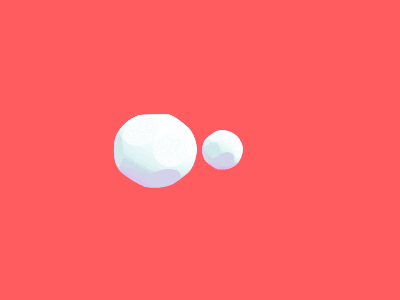
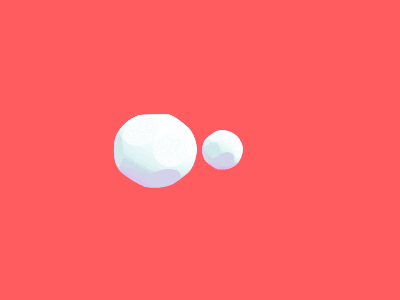