2022-08-30 更新
Cross-Lingual Cross-Modal Retrieval with Noise-Robust Learning
Authors:Yabing Wang, Jianfeng Dong, Tianxiang Liang, Minsong Zhang, Rui Cai, Xun Wang
Despite the recent developments in the field of cross-modal retrieval, there has been less research focusing on low-resource languages due to the lack of manually annotated datasets. In this paper, we propose a noise-robust cross-lingual cross-modal retrieval method for low-resource languages. To this end, we use Machine Translation (MT) to construct pseudo-parallel sentence pairs for low-resource languages. However, as MT is not perfect, it tends to introduce noise during translation, rendering textual embeddings corrupted and thereby compromising the retrieval performance. To alleviate this, we introduce a multi-view self-distillation method to learn noise-robust target-language representations, which employs a cross-attention module to generate soft pseudo-targets to provide direct supervision from the similarity-based view and feature-based view. Besides, inspired by the back-translation in unsupervised MT, we minimize the semantic discrepancies between origin sentences and back-translated sentences to further improve the noise robustness of the textual encoder. Extensive experiments are conducted on three video-text and image-text cross-modal retrieval benchmarks across different languages, and the results demonstrate that our method significantly improves the overall performance without using extra human-labeled data. In addition, equipped with a pre-trained visual encoder from a recent vision-and-language pre-training framework, i.e., CLIP, our model achieves a significant performance gain, showing that our method is compatible with popular pre-training models. Code and data are available at https://github.com/HuiGuanLab/nrccr.
PDF Accepted by ACM MM 2022. Code and data are available at https://github.com/HuiGuanLab/nrccr
点此查看论文截图
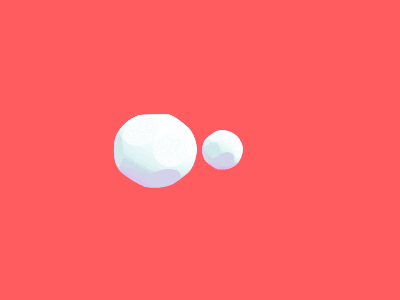
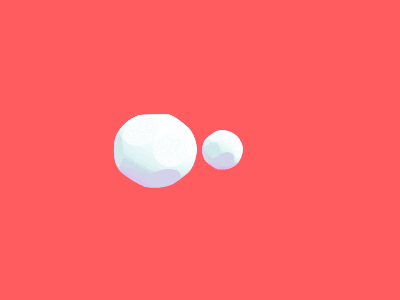
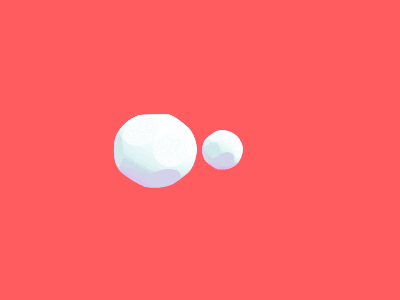
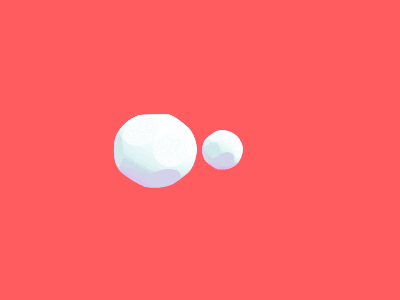
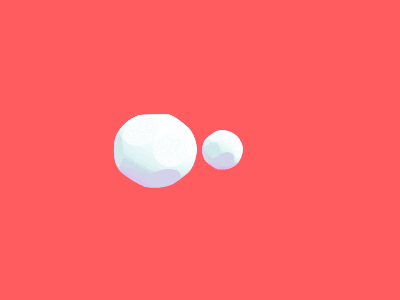
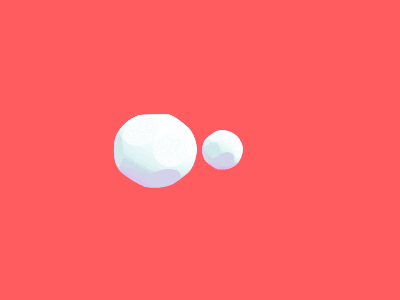
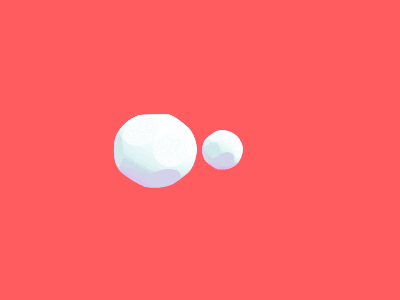
Rethinking and Improving Natural Language Generation with Layer-Wise Multi-View Decoding
Authors:Fenglin Liu, Xuancheng Ren, Guangxiang Zhao, Chenyu You, Xuewei Ma, Xian Wu, Xu Sun
In sequence-to-sequence learning, e.g., natural language generation, the decoder relies on the attention mechanism to efficiently extract information from the encoder. While it is common practice to draw information from only the last encoder layer, recent work has proposed to use representations from different encoder layers for diversified levels of information. Nonetheless, the decoder still obtains only a single view of the source sequences, which might lead to insufficient training of the encoder layer stack due to the hierarchy bypassing problem. In this work, we propose layer-wise multi-view decoding, where for each decoder layer, together with the representations from the last encoder layer, which serve as a global view, those from other encoder layers are supplemented for a stereoscopic view of the source sequences. Systematic experiments and analyses show that we successfully address the hierarchy bypassing problem, require almost negligible parameter increase, and substantially improve the performance of sequence-to-sequence learning with deep representations on five diverse tasks, i.e., machine translation, abstractive summarization, image captioning, video captioning, medical report generation, and paraphrase generation. In particular, our approach achieves new state-of-the-art results on ten benchmark datasets, including a low-resource machine translation dataset and two low-resource medical report generation datasets.
PDF
点此查看论文截图
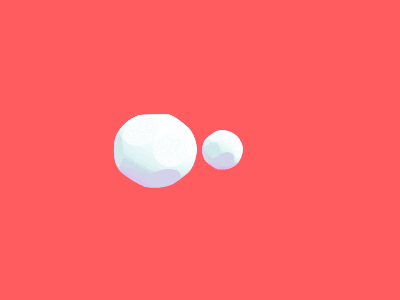
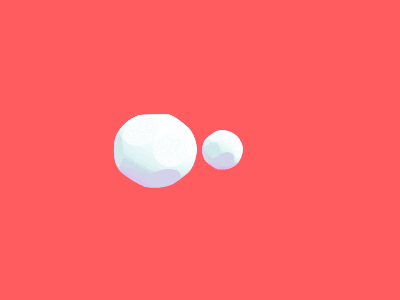
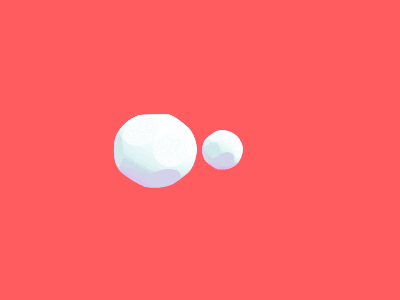
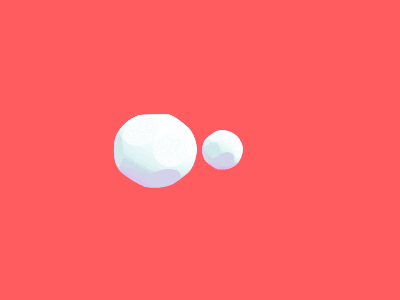
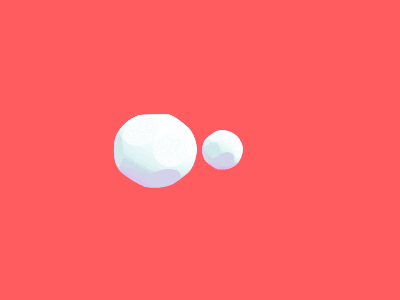
Learning Multi-Modal Brain Tumor Segmentation from Privileged Semi-Paired MRI Images with Curriculum Disentanglement Learning
Authors:Zecheng Liu, Jia Wei, Rui Li
Due to the difficulties of obtaining multimodal paired images in clinical practice, recent studies propose to train brain tumor segmentation models with unpaired images and capture complementary information through modality translation. However, these models cannot fully exploit the complementary information from different modalities. In this work, we thus present a novel two-step (intra-modality and inter-modality) curriculum disentanglement learning framework to effectively utilize privileged semi-paired images, i.e. limited paired images that are only available in training, for brain tumor segmentation. Specifically, in the first step, we propose to conduct reconstruction and segmentation with augmented intra-modality style-consistent images. In the second step, the model jointly performs reconstruction, unsupervised/supervised translation, and segmentation for both unpaired and paired inter-modality images. A content consistency loss and a supervised translation loss are proposed to leverage complementary information from different modalities in this step. Through these two steps, our method effectively extracts modality-specific style codes describing the attenuation of tissue features and image contrast, and modality-invariant content codes containing anatomical and functional information from the input images. Experiments on three brain tumor segmentation tasks show that our model outperforms competing segmentation models based on unpaired images.
PDF 8 pages, 10 figures
点此查看论文截图
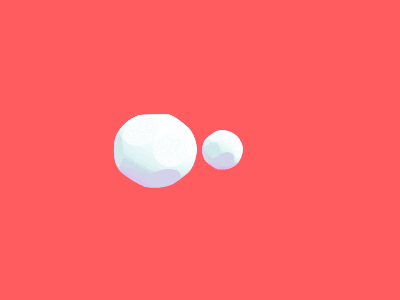
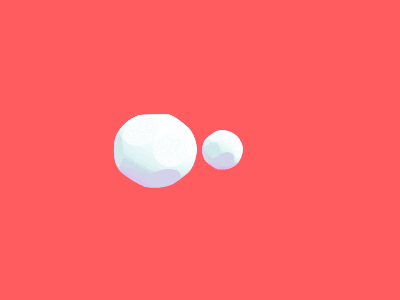
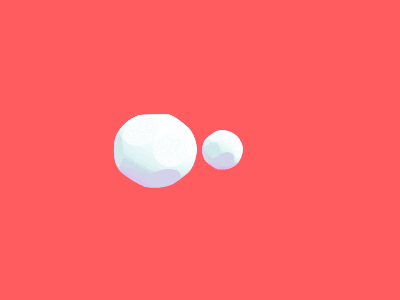
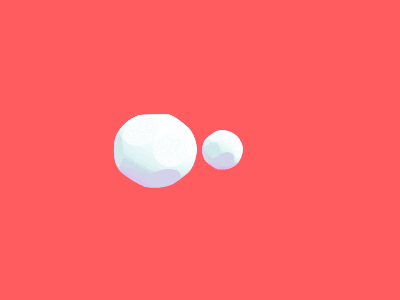
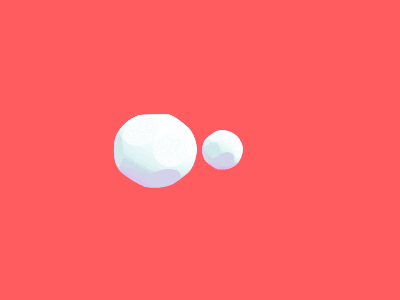
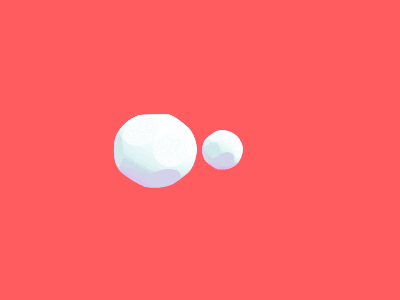
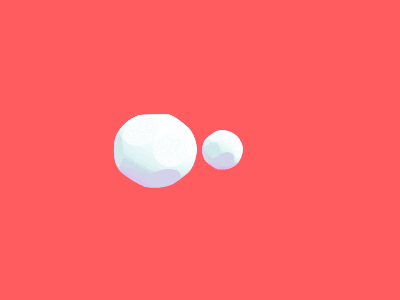
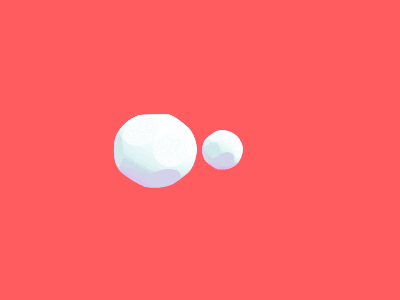
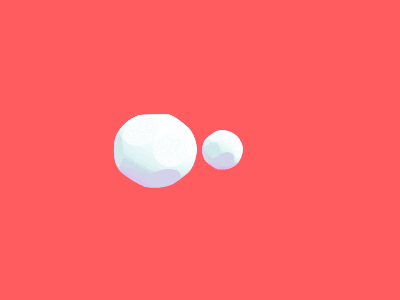
Adaptively-Realistic Image Generation from Stroke and Sketch with Diffusion Model
Authors:Shin-I Cheng, Yu-Jie Chen, Wei-Chen Chiu, Hsin-Ying Lee, Hung-Yu Tseng
Generating images from hand-drawings is a crucial and fundamental task in content creation. The translation is difficult as there exist infinite possibilities and the different users usually expect different outcomes. Therefore, we propose a unified framework supporting a three-dimensional control over the image synthesis from sketches and strokes based on diffusion models. Users can not only decide the level of faithfulness to the input strokes and sketches, but also the degree of realism, as the user inputs are usually not consistent with the real images. Qualitative and quantitative experiments demonstrate that our framework achieves state-of-the-art performance while providing flexibility in generating customized images with control over shape, color, and realism. Moreover, our method unleashes applications such as editing on real images, generation with partial sketches and strokes, and multi-domain multi-modal synthesis.
PDF
点此查看论文截图
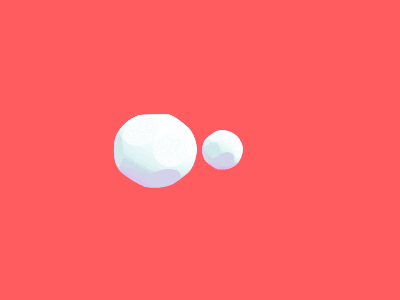
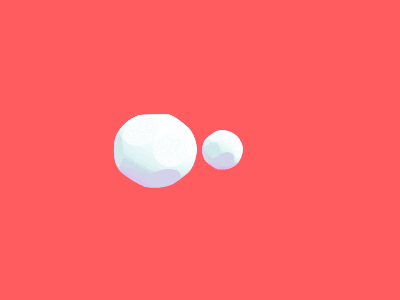
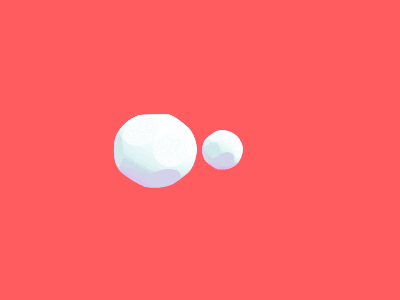
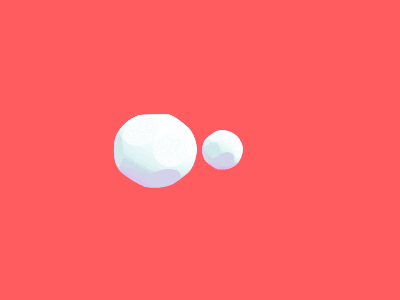
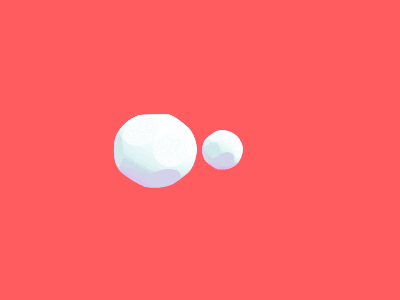
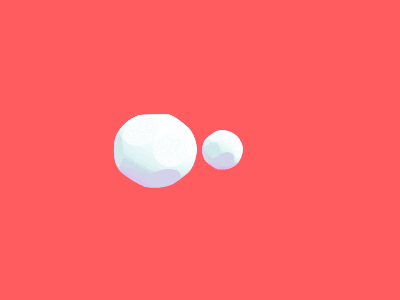
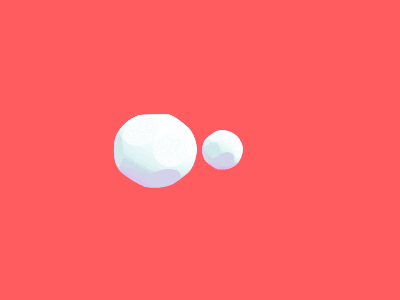
Comparison and Analysis of Image-to-Image Generative Adversarial Networks: A Survey
Authors:Sagar Saxena, Mohammad Nayeem Teli
Generative Adversarial Networks (GANs) have recently introduced effective methods of performing Image-to-Image translations. These models can be applied and generalized to a variety of domains in Image-to-Image translation without changing any parameters. In this paper, we survey and analyze eight Image-to-Image Generative Adversarial Networks: Pix2Pix, CycleGAN, CoGAN, StarGAN, MUNIT, StarGAN2, DA-GAN, and Self Attention GAN. Each of these models presented state-of-the-art results and introduced new techniques to build Image-to-Image GANs. In addition to a survey of the models, we also survey the 18 datasets they were trained on and the 9 metrics they were evaluated on. Finally, we present results of a controlled experiment for 6 of these models on a common set of metrics and datasets. The results were mixed and showed that, on certain datasets, tasks, and metrics, some models outperformed others. The last section of this paper discusses those results and establishes areas of future research. As researchers continue to innovate new Image-to-Image GANs, it is important to gain a good understanding of the existing methods, datasets, and metrics. This paper provides a comprehensive overview and discussion to help build this foundation.
PDF 36 pages, 22 figures, Preprint; format changed, typos corrected
点此查看论文截图
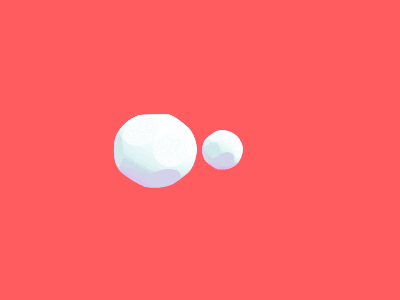
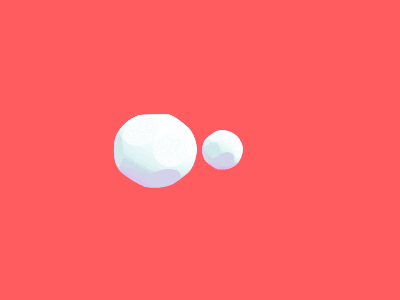
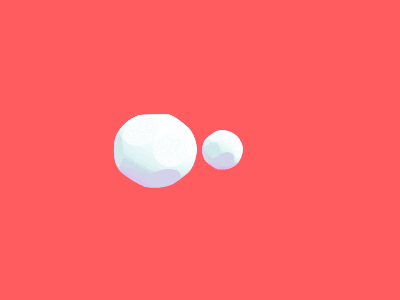