2022-08-27 更新
FairDisCo: Fairer AI in Dermatology via Disentanglement Contrastive Learning
Authors:Siyi Du, Ben Hers, Nourhan Bayasi, Ghassan Hamarneh, Rafeef Garbi
Deep learning models have achieved great success in automating skin lesion diagnosis. However, the ethnic disparity in these models’ predictions, where lesions on darker skin types are usually underrepresented and have lower diagnosis accuracy, receives little attention. In this paper, we propose FairDisCo, a disentanglement deep learning framework with contrastive learning that utilizes an additional network branch to remove sensitive attributes, i.e. skin-type information from representations for fairness and another contrastive branch to enhance feature extraction. We compare FairDisCo to three fairness methods, namely, resampling, reweighting, and attribute-aware, on two newly released skin lesion datasets with different skin types: Fitzpatrick17k and Diverse Dermatology Images (DDI). We adapt two fairness-based metrics DPM and EOM for our multiple classes and sensitive attributes task, highlighting the skin-type bias in skin lesion classification. Extensive experimental evaluation demonstrates the effectiveness of FairDisCo, with fairer and superior performance on skin lesion classification tasks.
PDF 14 pages, 3 figures, accepted by European Conference on Computer Vision (ECCV) ISIC Workshops, 2022
点此查看论文截图
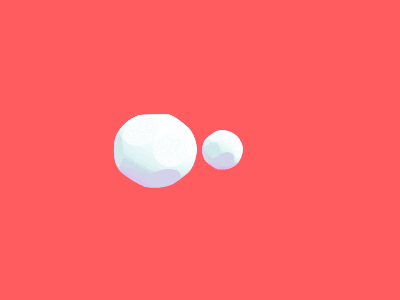
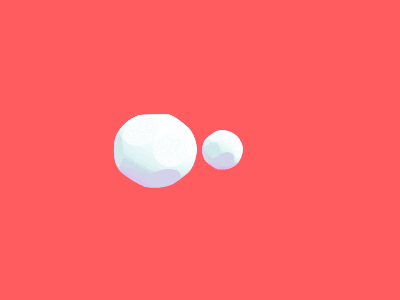
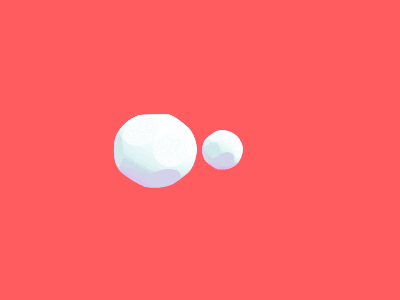
Efficient Self-Supervision using Patch-based Contrastive Learning for Histopathology Image Segmentation
Authors:Nicklas Boserup, Raghavendra Selvan
Learning discriminative representations of unlabelled data is a challenging task. Contrastive self-supervised learning provides a framework to learn meaningful representations using learned notions of similarity measures from simple pretext tasks. In this work, we propose a simple and efficient framework for self-supervised image segmentation using contrastive learning on image patches, without using explicit pretext tasks or any further labeled fine-tuning. A fully convolutional neural network (FCNN) is trained in a self-supervised manner to discern features in the input images and obtain confidence maps which capture the network’s belief about the objects belonging to the same class. Positive- and negative- patches are sampled based on the average entropy in the confidence maps for contrastive learning. Convergence is assumed when the information separation between the positive patches is small, and the positive-negative pairs is large. We evaluate this method for the task of segmenting nuclei from multiple histopathology datasets, and show comparable performance with relevant self-supervised and supervised methods. The proposed model only consists of a simple FCNN with 10.8k parameters and requires about 5 minutes to converge on the high resolution microscopy datasets, which is orders of magnitude smaller than the relevant self-supervised methods to attain similar performance.
PDF 15 pages, 8 figures. Source code at https://github.com/nickeopti/bach-contrastive-segmentation
点此查看论文截图
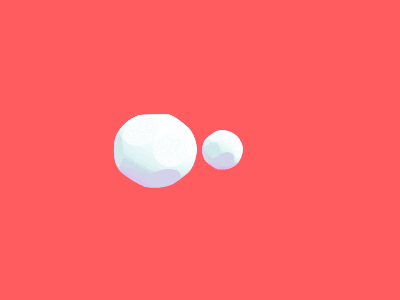
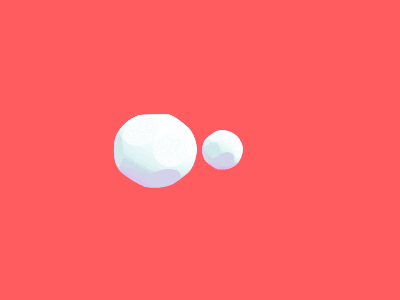
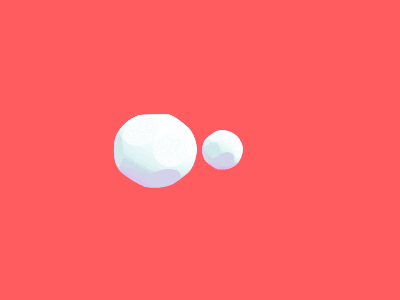
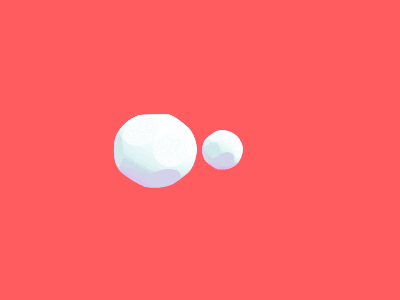
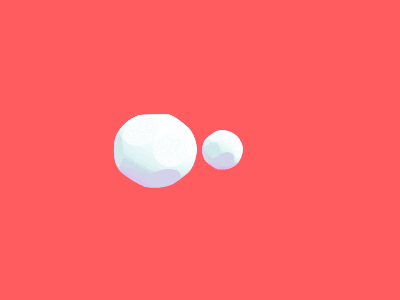
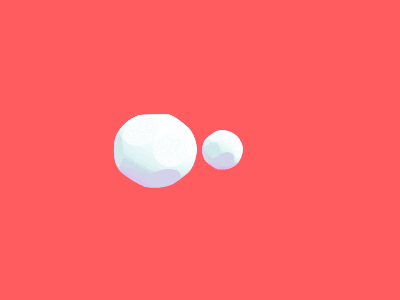
MaskCLIP: Masked Self-Distillation Advances Contrastive Language-Image Pretraining
Authors:Xiaoyi Dong, Yinglin Zheng, Jianmin Bao, Ting Zhang, Dongdong Chen, Hao Yang, Ming Zeng, Weiming Zhang, Lu Yuan, Dong Chen, Fang Wen, Nenghai Yu
This paper presents a simple yet effective framework MaskCLIP, which incorporates a newly proposed masked self-distillation into contrastive language-image pretraining. The core idea of masked self-distillation is to distill representation from a full image to the representation predicted from a masked image. Such incorporation enjoys two vital benefits. First, masked self-distillation targets local patch representation learning, which is complementary to vision-language contrastive focusing on text-related representation.Second, masked self-distillation is also consistent with vision-language contrastive from the perspective of training objective as both utilize the visual encoder for feature aligning, and thus is able to learn local semantics getting indirect supervision from the language. We provide specially designed experiments with a comprehensive analysis to validate the two benefits. Empirically, we show that MaskCLIP, when applied to various challenging downstream tasks, achieves superior results in linear probing, finetuning as well as the zero-shot performance with the guidance of the language encoder.
PDF
点此查看论文截图
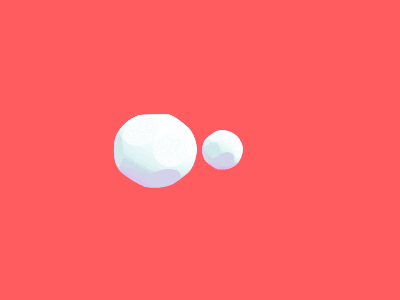
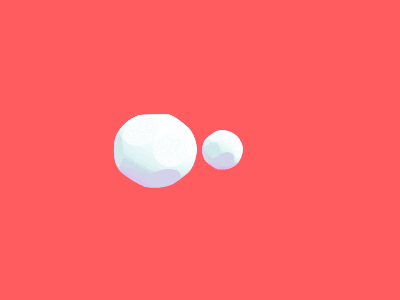
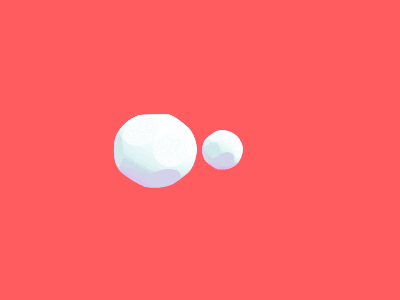
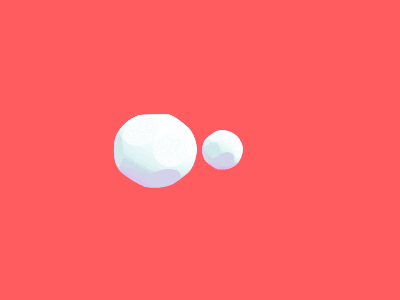
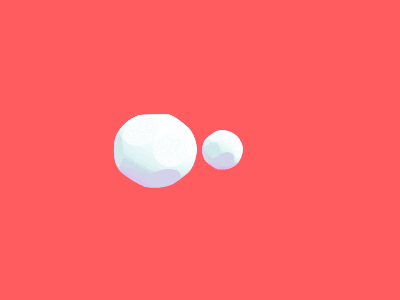
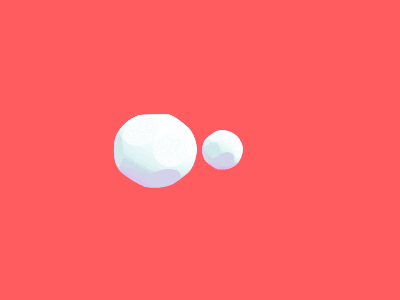
Data Determines Distributional Robustness in Contrastive Language Image Pre-training (CLIP)
Authors:Alex Fang, Gabriel Ilharco, Mitchell Wortsman, Yuhao Wan, Vaishaal Shankar, Achal Dave, Ludwig Schmidt
Contrastively trained language-image models such as CLIP, ALIGN, and BASIC have demonstrated unprecedented robustness to multiple challenging natural distribution shifts. Since these language-image models differ from previous training approaches in several ways, an important question is what causes the large robustness gains. We answer this question via a systematic experimental investigation. Concretely, we study five different possible causes for the robustness gains: (i) the training set size, (ii) the training distribution, (iii) language supervision at training time, (iv) language supervision at test time, and (v) the contrastive loss function. Our experiments show that the more diverse training distribution is the main cause for the robustness gains, with the other factors contributing little to no robustness. Beyond our experimental results, we also introduce ImageNet-Captions, a version of ImageNet with original text annotations from Flickr, to enable further controlled experiments of language-image training.
PDF
点此查看论文截图
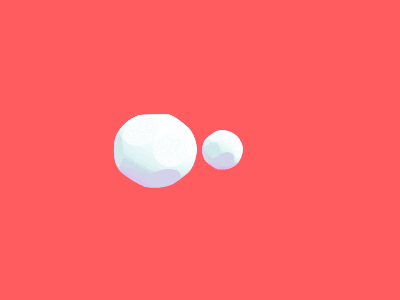
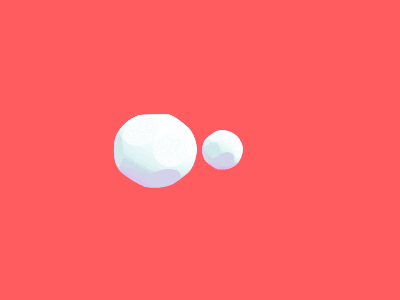
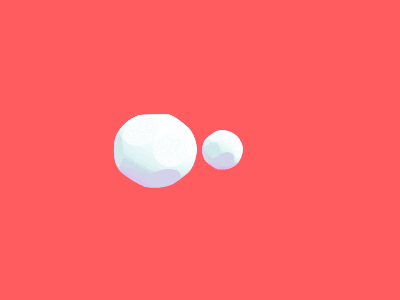
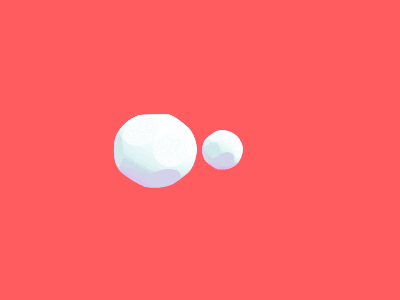
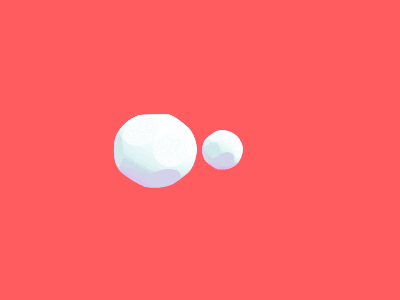
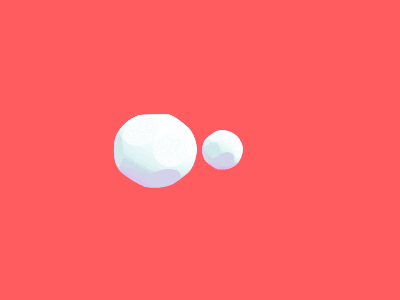
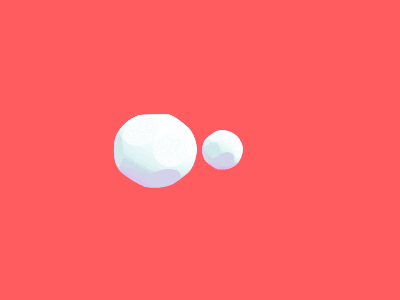