2022-08-19 更新
Semi-supervised domain adaptation with CycleGAN guided by a downstream task loss
Authors:Annika Mütze, Matthias Rottmann, Hanno Gottschalk
Domain adaptation is of huge interest as labeling is an expensive and error-prone task, especially when labels are needed on pixel-level like in semantic segmentation. Therefore, one would like to be able to train neural networks on synthetic domains, where data is abundant and labels are precise. However, these models often perform poorly on out-of-domain images. To mitigate the shift in the input, image-to-image approaches can be used. Nevertheless, standard image-to-image approaches that bridge the domain of deployment with the synthetic training domain do not focus on the downstream task but only on the visual inspection level. We therefore propose a “task aware” version of a GAN in an image-to-image domain adaptation approach. With the help of a small amount of labeled ground truth data, we guide the image-to-image translation to a more suitable input image for a semantic segmentation network trained on synthetic data (synthetic-domain expert). The main contributions of this work are 1) a modular semi-supervised domain adaptation method for semantic segmentation by training a downstream task aware CycleGAN while refraining from adapting the synthetic semantic segmentation expert 2) the demonstration that the method is applicable to complex domain adaptation tasks and 3) a less biased domain gap analysis by using from scratch networks. We evaluate our method on a classification task as well as on semantic segmentation. Our experiments demonstrate that our method outperforms CycleGAN - a standard image-to-image approach - by 7 percent points in accuracy in a classification task using only 70 (10%) ground truth images. For semantic segmentation we can show an improvement of about 4 to 7 percent points in mean Intersection over union on the Cityscapes evaluation dataset with only 14 ground truth images during training.
PDF 11pages, 11figures
点此查看论文截图
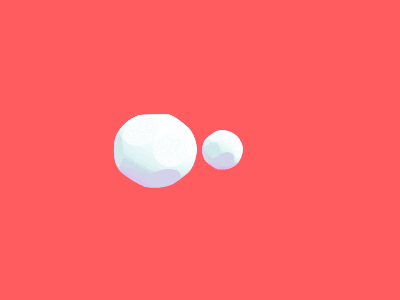
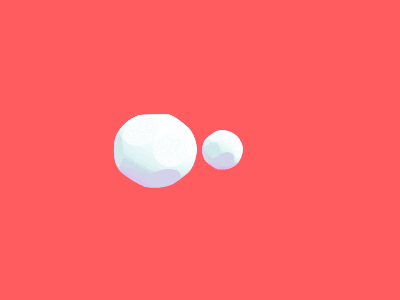
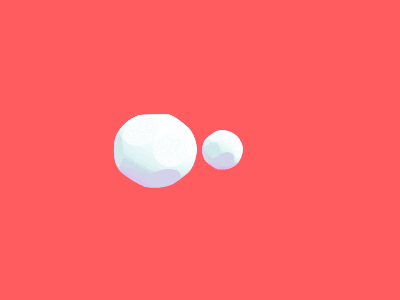
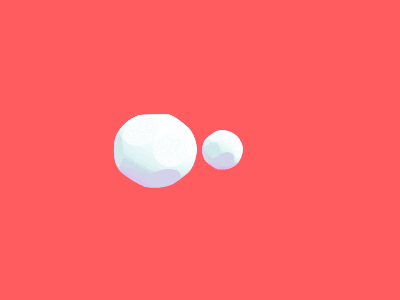
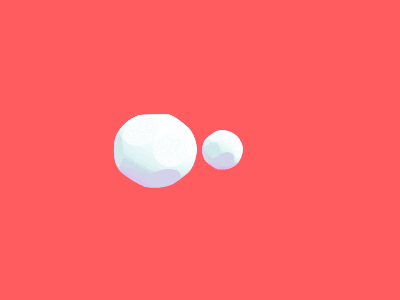
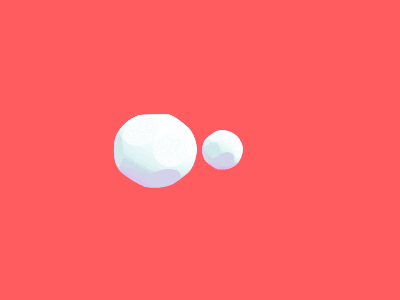
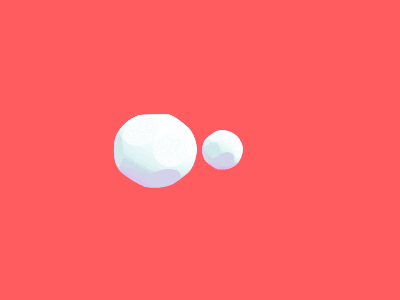
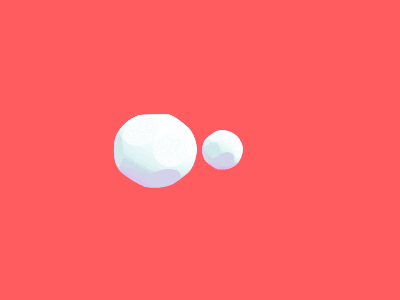
Text-to-Image Generation via Implicit Visual Guidance and Hypernetwork
Authors:Xin Yuan, Zhe Lin, Jason Kuen, Jianming Zhang, John Collomosse
We develop an approach for text-to-image generation that embraces additional retrieval images, driven by a combination of implicit visual guidance loss and generative objectives. Unlike most existing text-to-image generation methods which merely take the text as input, our method dynamically feeds cross-modal search results into a unified training stage, hence improving the quality, controllability and diversity of generation results. We propose a novel hypernetwork modulated visual-text encoding scheme to predict the weight update of the encoding layer, enabling effective transfer from visual information (e.g. layout, content) into the corresponding latent domain. Experimental results show that our model guided with additional retrieval visual data outperforms existing GAN-based models. On COCO dataset, we achieve better FID of $9.13$ with up to $3.5 \times$ fewer generator parameters, compared with the state-of-the-art method.
PDF
点此查看论文截图
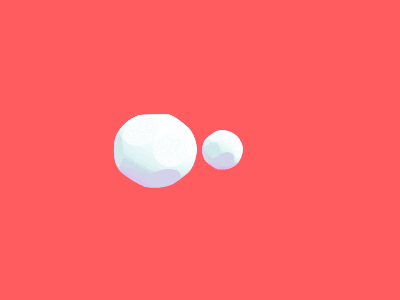
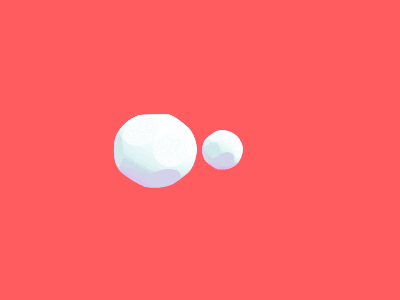
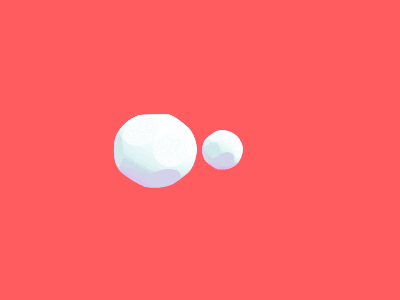
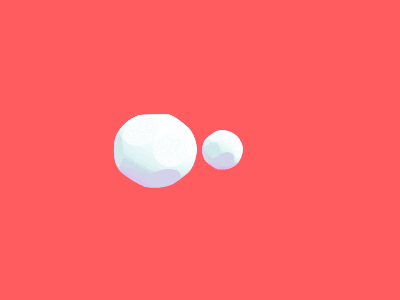
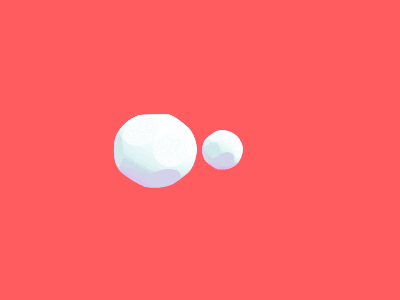
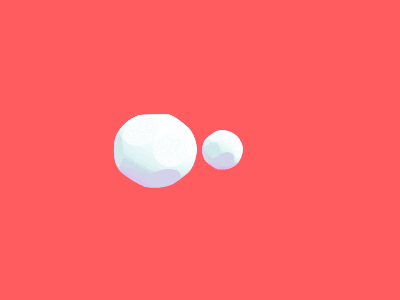
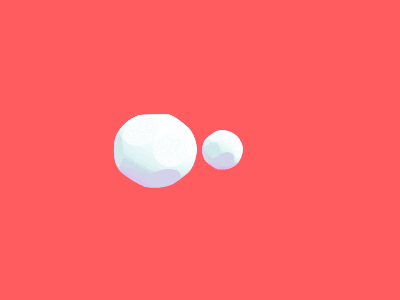
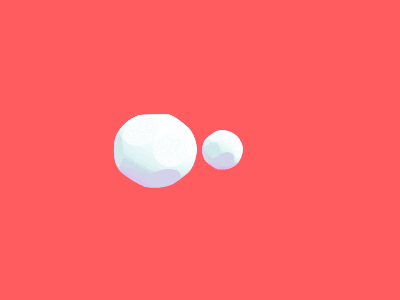
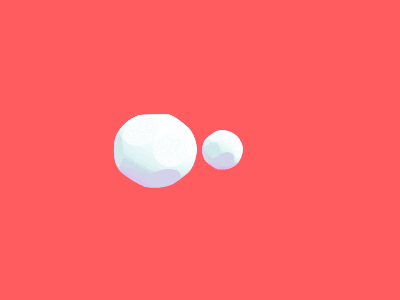