2022-08-18 更新
Adversarial Relighting Against Face Recognition
Authors:Ruijun Gao, Qing Guo, Qian Zhang, Felix Juefei-Xu, Hongkai Yu, Wei Feng
Deep face recognition (FR) has achieved significantly high accuracy on several challenging datasets and fosters successful real-world applications, even showing high robustness to the illumination variation that is usually regarded as a main threat to the FR system. However, in the real world, illumination variation caused by diverse lighting conditions cannot be fully covered by the limited face dataset. In this paper, we study the threat of lighting against FR from a new angle, i.e., adversarial attack, and identify a new task, i.e., adversarial relighting. Given a face image, adversarial relighting aims to produce a naturally relighted counterpart while fooling the state-of-the-art deep FR methods. To this end, we first propose the physical model-based adversarial relighting attack (ARA) denoted as albedo-quotient-based adversarial relighting attack (AQ-ARA). It generates natural adversarial light under the physical lighting model and guidance of FR systems and synthesizes adversarially relighted face images. Moreover, we propose the auto-predictive adversarial relighting attack (AP-ARA) by training an adversarial relighting network (ARNet) to automatically predict the adversarial light in a one-step manner according to different input faces, allowing efficiency-sensitive applications. More importantly, we propose to transfer the above digital attacks to physical ARA (Phy-ARA) through a precise relighting device, making the estimated adversarial lighting condition reproducible in the real world. We validate our methods on three state-of-the-art deep FR methods, i.e., FaceNet, ArcFace, and CosFace, on two public datasets. The extensive and insightful results demonstrate our work can generate realistic adversarial relighted face images fooling FR easily, revealing the threat of specific light directions and strengths.
PDF
点此查看论文截图
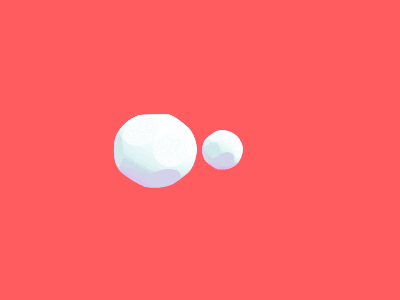
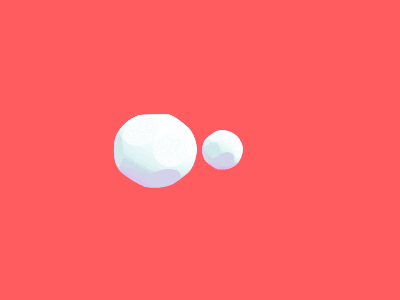
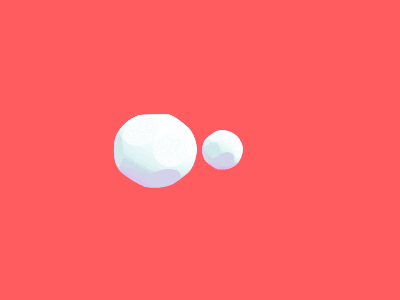
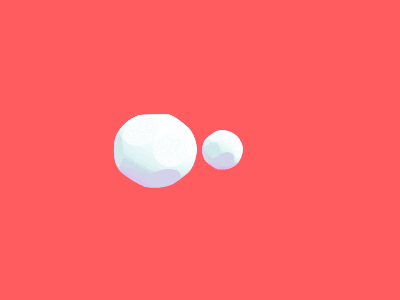
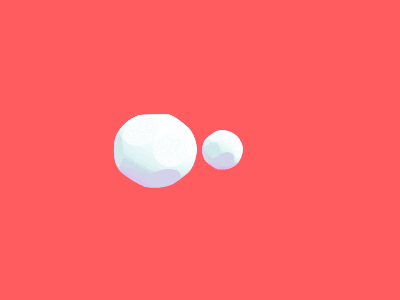
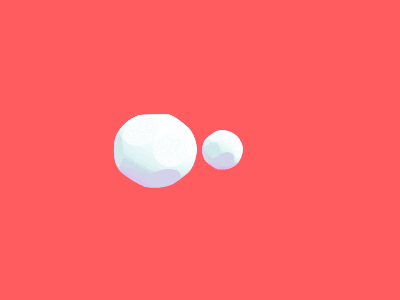
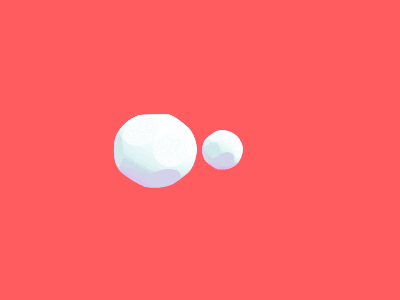
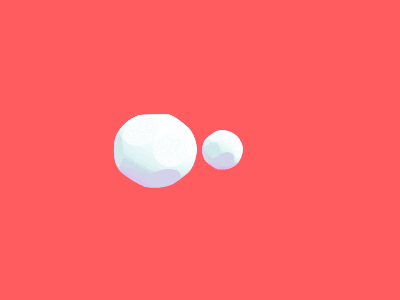
A Physical-World Adversarial Attack for 3D Face Recognition
Authors:Yanjie Li, Yiquan Li, Xuelong Dai, Songtao Guo, Bin Xiao
The 3D face recognition has long been considered secure for its resistance to current physical adversarial attacks, like adversarial patches. However, this paper shows that a 3D face recognition system can be easily attacked, leading to evading and impersonation attacks. We are the first to propose a physically realizable attack for the 3D face recognition system, named structured light imaging attack (SLIA), which exploits the weakness of structured-light-based 3D scanning devices. SLIA utilizes the projector in the structured light imaging system to create adversarial illuminations to contaminate the reconstructed point cloud. Firstly, we propose a 3D transform-invariant loss function (3D-TI) to generate adversarial perturbations that are more robust to head movements. Then we integrate the 3D imaging process into the attack optimization, which minimizes the total pixel shifting of fringe patterns. We realize both dodging and impersonation attacks on a real-world 3D face recognition system. Our methods need fewer modifications on projected patterns compared with Chamfer and Chamfer+kNN-based methods and achieve average attack success rates of 0.47 (impersonation) and 0.89 (dodging). This paper exposes the insecurity of present structured light imaging technology and sheds light on designing secure 3D face recognition authentication systems.
PDF 7 pages, 5 figures, Submit to AAAI 2023
点此查看论文截图
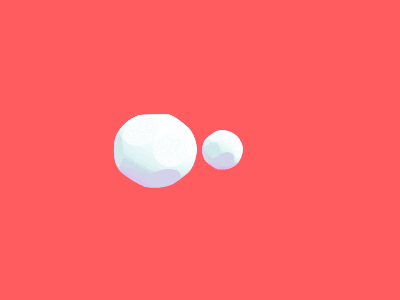
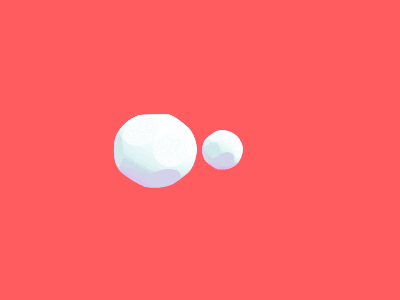
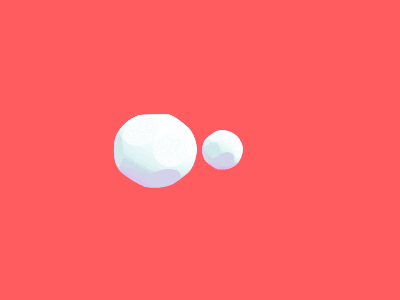
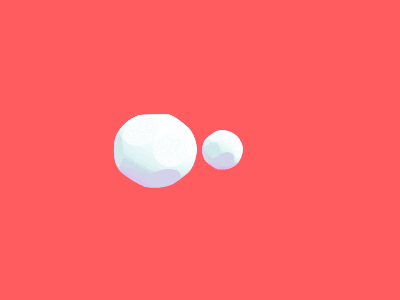
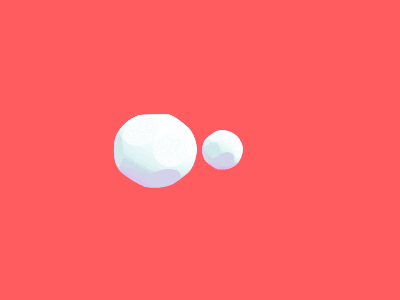
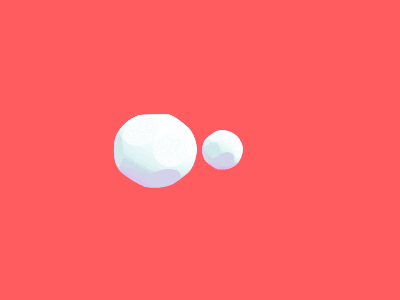
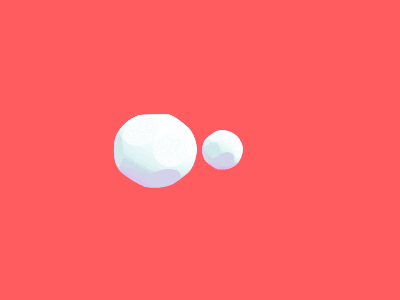
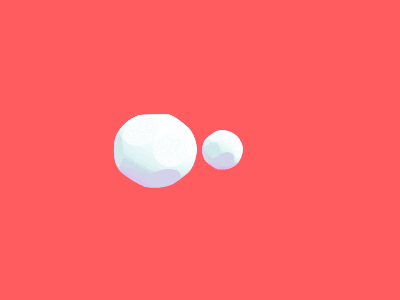
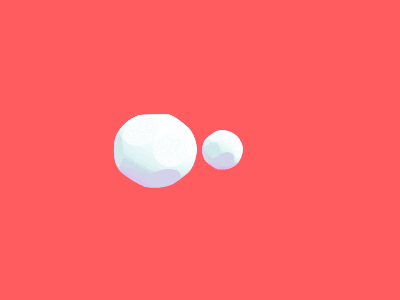