2022-08-16 更新
AVisT: A Benchmark for Visual Object Tracking in Adverse Visibility
Authors:Mubashir Noman, Wafa Al Ghallabi, Daniya Najiha, Christoph Mayer, Akshay Dudhane, Martin Danelljan, Hisham Cholakkal, Salman Khan, Luc Van Gool, Fahad Shahbaz Khan
One of the key factors behind the recent success in visual tracking is the availability of dedicated benchmarks. While being greatly benefiting to the tracking research, existing benchmarks do not pose the same difficulty as before with recent trackers achieving higher performance mainly due to (i) the introduction of more sophisticated transformers-based methods and (ii) the lack of diverse scenarios with adverse visibility such as, severe weather conditions, camouflage and imaging effects. We introduce AVisT, a dedicated benchmark for visual tracking in diverse scenarios with adverse visibility. AVisT comprises 120 challenging sequences with 80k annotated frames, spanning 18 diverse scenarios broadly grouped into five attributes with 42 object categories. The key contribution of AVisT is diverse and challenging scenarios covering severe weather conditions such as, dense fog, heavy rain and sandstorm; obstruction effects including, fire, sun glare and splashing water; adverse imaging effects such as, low-light; target effects including, small targets and distractor objects along with camouflage. We further benchmark 17 popular and recent trackers on AVisT with detailed analysis of their tracking performance across attributes, demonstrating a big room for improvement in performance. We believe that AVisT can greatly benefit the tracking community by complementing the existing benchmarks, in developing new creative tracking solutions in order to continue pushing the boundaries of the state-of-the-art. Our dataset along with the complete tracking performance evaluation is available at: https://github.com/visionml/pytracking
PDF
点此查看论文截图
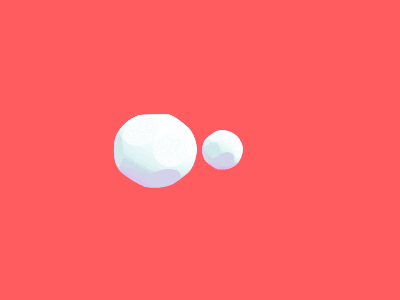
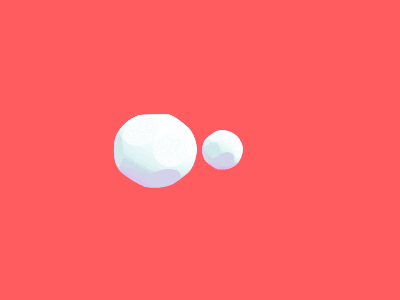
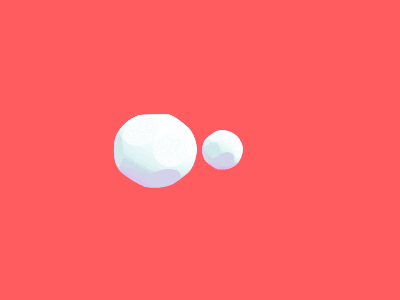
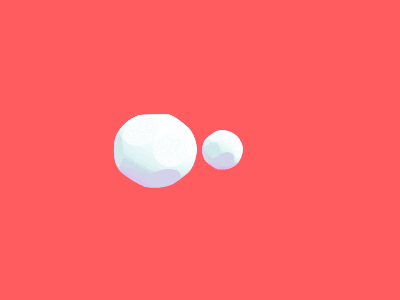
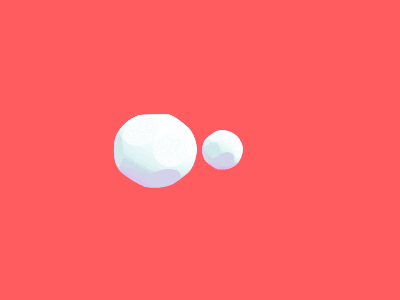
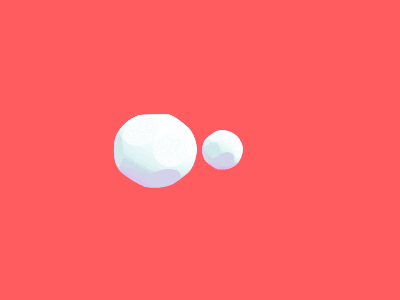
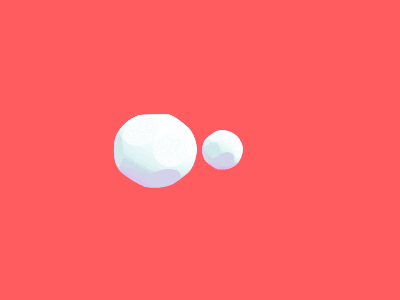
Surrogate-assisted Multi-objective Neural Architecture Search for Real-time Semantic Segmentation
Authors:Zhichao Lu, Ran Cheng, Shihua Huang, Haoming Zhang, Changxiao Qiu, Fan Yang
The architectural advancements in deep neural networks have led to remarkable leap-forwards across a broad array of computer vision tasks. Instead of relying on human expertise, neural architecture search (NAS) has emerged as a promising avenue toward automating the design of architectures. While recent achievements in image classification have suggested opportunities, the promises of NAS have yet to be thoroughly assessed on more challenging tasks of semantic segmentation. The main challenges of applying NAS to semantic segmentation arise from two aspects: (i) high-resolution images to be processed; (ii) additional requirement of real-time inference speed (i.e., real-time semantic segmentation) for applications such as autonomous driving. To meet such challenges, we propose a surrogate-assisted multi-objective method in this paper. Through a series of customized prediction models, our method effectively transforms the original NAS task into an ordinary multi-objective optimization problem. Followed by a hierarchical pre-screening criterion for in-fill selection, our method progressively achieves a set of efficient architectures trading-off between segmentation accuracy and inference speed. Empirical evaluations on three benchmark datasets together with an application using Huawei Atlas 200 DK suggest that our method can identify architectures significantly outperforming existing state-of-the-art architectures designed both manually by human experts and automatically by other NAS methods.
PDF
点此查看论文截图
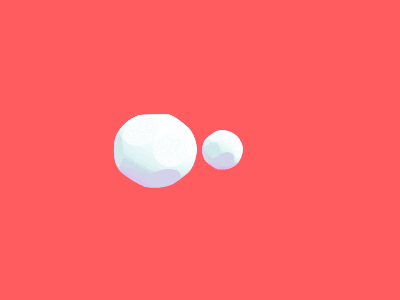
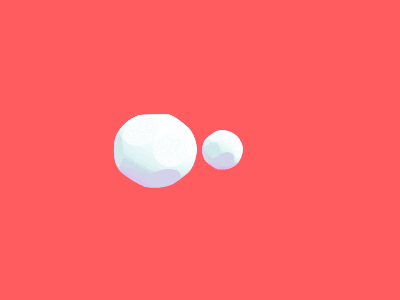
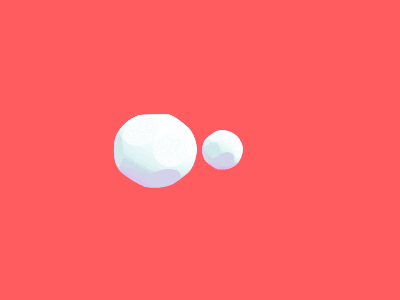
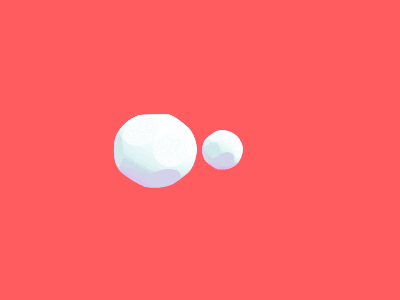
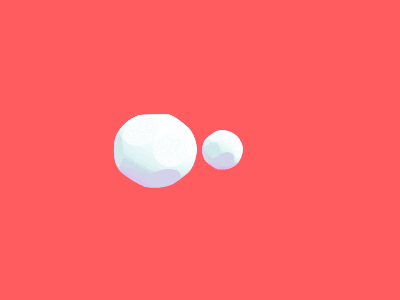
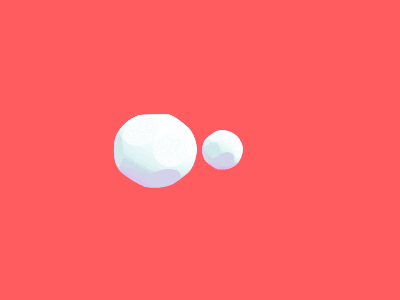
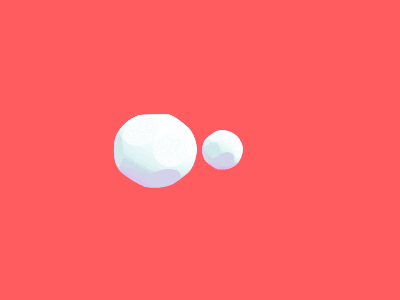
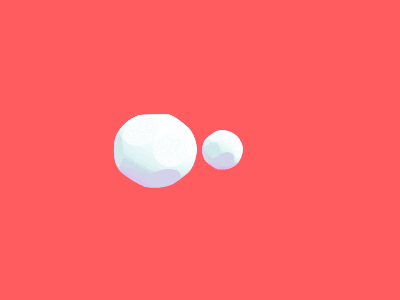
Hierarchical Attention Network for Few-Shot Object Detection via Meta-Contrastive Learning
Authors:Dongwoo Park, Jongmin Lee
Few-shot object detection (FSOD) aims to classify and detect few images of novel categories. Existing meta-learning methods insufficiently exploit features between support and query images owing to structural limitations. We propose a hierarchical attention network with sequentially large receptive fields to fully exploit the query and support images. In addition, meta-learning does not distinguish the categories well because it determines whether the support and query images match. In other words, metric-based learning for classification is ineffective because it does not work directly. Thus, we propose a contrastive learning method called meta-contrastive learning, which directly helps achieve the purpose of the meta-learning strategy. Finally, we establish a new state-of-the-art network, by realizing significant margins. Our method brings 2.3, 1.0, 1.3, 3.4 and 2.4\% AP improvements for 1-30 shots object detection on COCO dataset. Our code is available at: https://github.com/infinity7428/hANMCL
PDF
点此查看论文截图
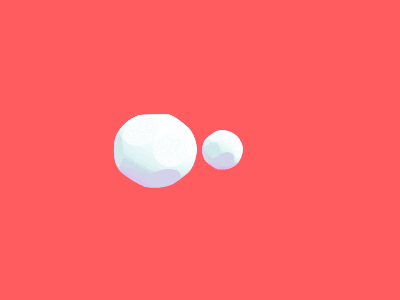
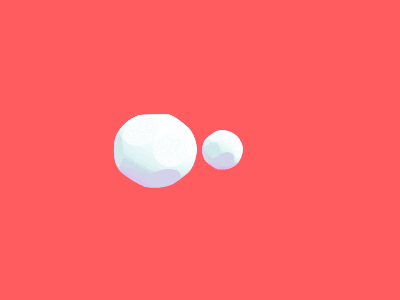
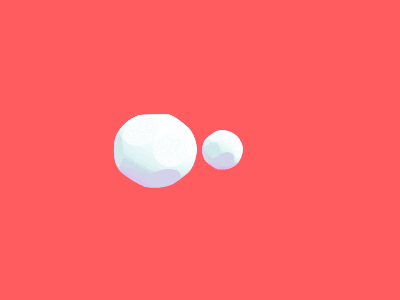
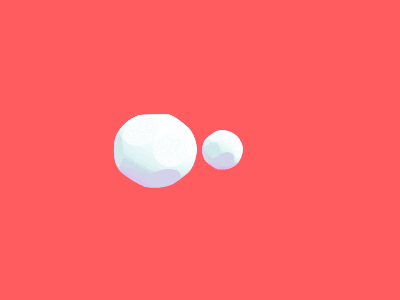
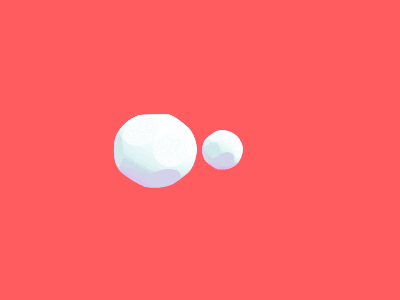
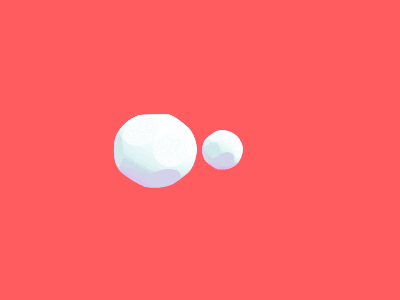
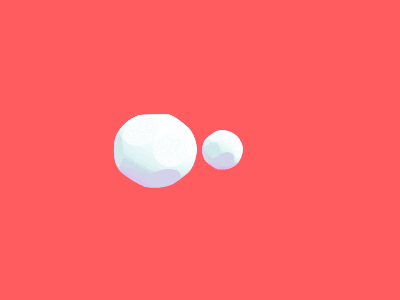
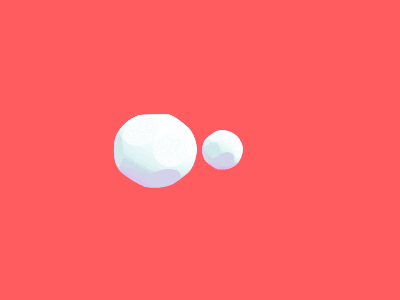
Continual Unsupervised Domain Adaptation for Semantic Segmentation using a Class-Specific Transfer
Authors:Robert A. Marsden, Felix Wiewel, Mario Döbler, Yang Yang, Bin Yang
In recent years, there has been tremendous progress in the field of semantic segmentation. However, one remaining challenging problem is that segmentation models do not generalize to unseen domains. To overcome this problem, one either has to label lots of data covering the whole variety of domains, which is often infeasible in practice, or apply unsupervised domain adaptation (UDA), only requiring labeled source data. In this work, we focus on UDA and additionally address the case of adapting not only to a single domain, but to a sequence of target domains. This requires mechanisms preventing the model from forgetting its previously learned knowledge. To adapt a segmentation model to a target domain, we follow the idea of utilizing light-weight style transfer to convert the style of labeled source images into the style of the target domain, while retaining the source content. To mitigate the distributional shift between the source and the target domain, the model is fine-tuned on the transferred source images in a second step. Existing light-weight style transfer approaches relying on adaptive instance normalization (AdaIN) or Fourier transformation still lack performance and do not substantially improve upon common data augmentation, such as color jittering. The reason for this is that these methods do not focus on region- or class-specific differences, but mainly capture the most salient style. Therefore, we propose a simple and light-weight framework that incorporates two class-conditional AdaIN layers. To extract the class-specific target moments needed for the transfer layers, we use unfiltered pseudo-labels, which we show to be an effective approximation compared to real labels. We extensively validate our approach (CACE) on a synthetic sequence and further propose a challenging sequence consisting of real domains. CACE outperforms existing methods visually and quantitatively.
PDF Accepted at International Joint Conference on Neural Networks 2022 (IJCNN)
点此查看论文截图
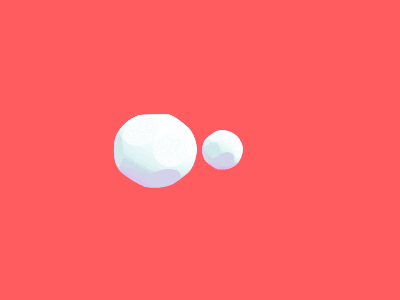
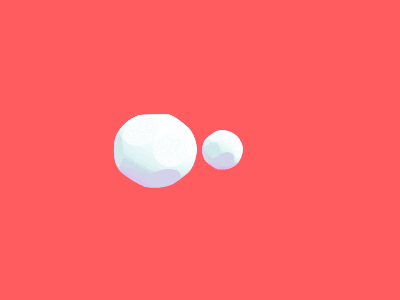
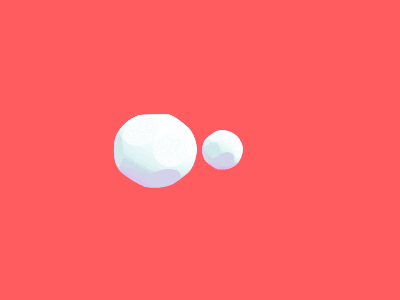
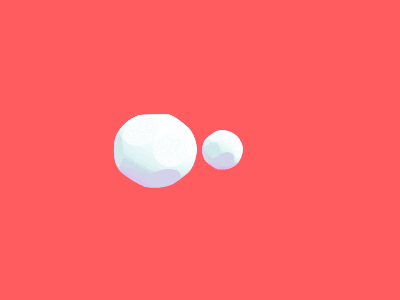
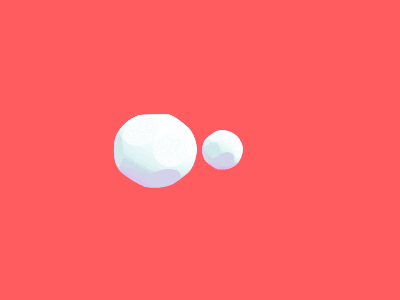
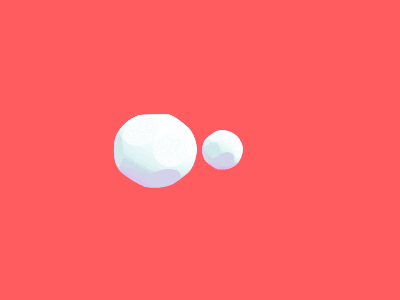
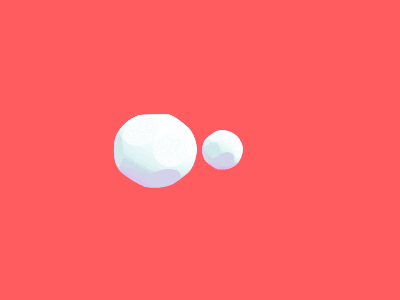
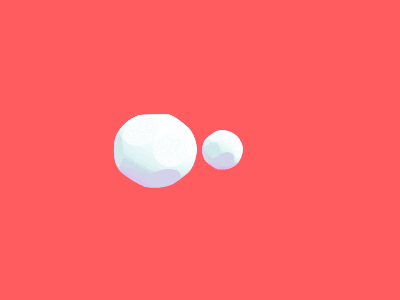
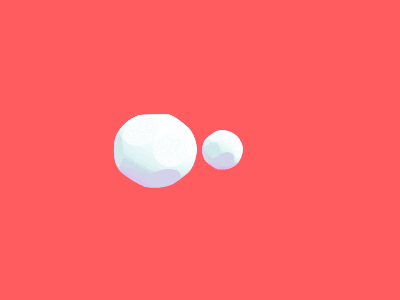