2022-08-16 更新
Self-supervised Matting-specific Portrait Enhancement and Generation
Authors:Yangyang Xu Zeyang Zhou, Shengfeng He
We resolve the ill-posed alpha matting problem from a completely different perspective. Given an input portrait image, instead of estimating the corresponding alpha matte, we focus on the other end, to subtly enhance this input so that the alpha matte can be easily estimated by any existing matting models. This is accomplished by exploring the latent space of GAN models. It is demonstrated that interpretable directions can be found in the latent space and they correspond to semantic image transformations. We further explore this property in alpha matting. Particularly, we invert an input portrait into the latent code of StyleGAN, and our aim is to discover whether there is an enhanced version in the latent space which is more compatible with a reference matting model. We optimize multi-scale latent vectors in the latent spaces under four tailored losses, ensuring matting-specificity and subtle modifications on the portrait. We demonstrate that the proposed method can refine real portrait images for arbitrary matting models, boosting the performance of automatic alpha matting by a large margin. In addition, we leverage the generative property of StyleGAN, and propose to generate enhanced portrait data which can be treated as the pseudo GT. It addresses the problem of expensive alpha matte annotation, further augmenting the matting performance of existing models. Code is available at~\url{https://github.com/cnnlstm/StyleGAN_Matting}.
PDF
点此查看论文截图
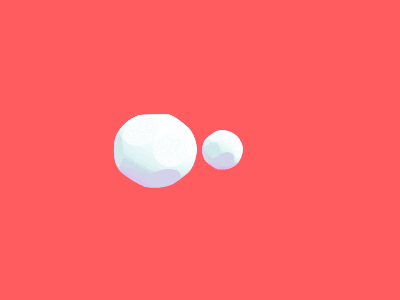
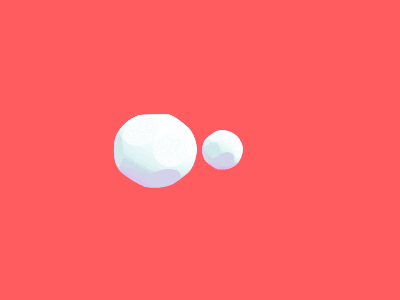
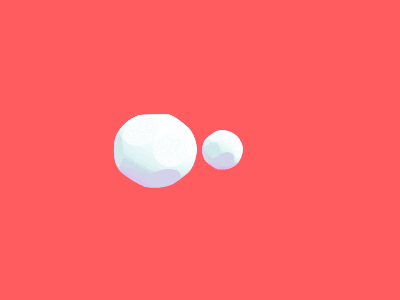
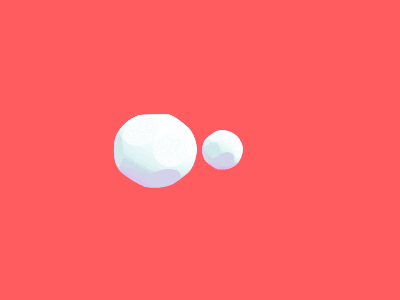
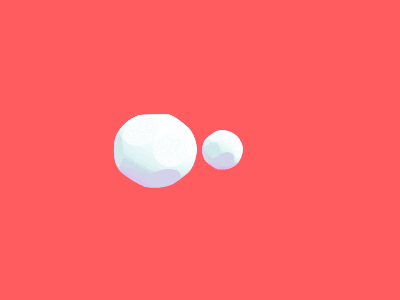
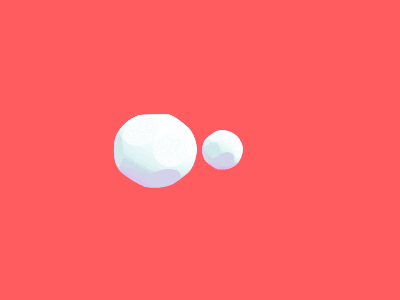
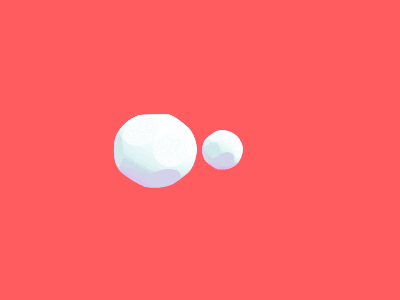
Do Inpainting Yourself: Generative Facial Inpainting Guided by Exemplars
Authors:Wanglong Lu, Hanli Zhao, Xianta Jiang, Xiaogang Jin, Yongliang Yang, Min Wang, Jiankai Lyu, Kaijie Shi
We present EXE-GAN, a novel exemplar-guided facial inpainting framework using generative adversarial networks. Our approach can not only preserve the quality of the input facial image but also complete the image with exemplar-like facial attributes. We achieve this by simultaneously leveraging the global style of the input image, the stochastic style generated from the random latent code, and the exemplar style of exemplar image. We introduce a novel attribute similarity metric to encourage networks to learn the style of facial attributes from the exemplar in a self-supervised way. To guarantee the natural transition across the boundaries of inpainted regions, we introduce a novel spatial variant gradient backpropagation technique to adjust the loss gradients based on the spatial location. Extensive evaluations and practical applications on public CelebA-HQ and FFHQ datasets validate the superiority of EXE-GAN in terms of the visual quality in facial inpainting.
PDF There are 16 pages, 18 figures in this paper. Homepage: https://longlongaaago.github.io/EXE-GAN/
点此查看论文截图
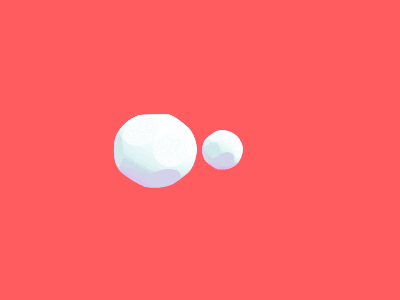
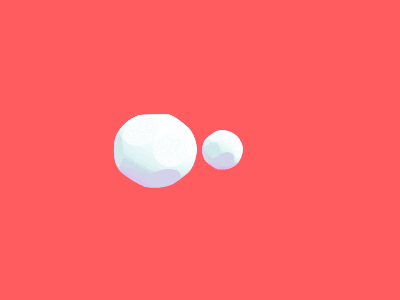
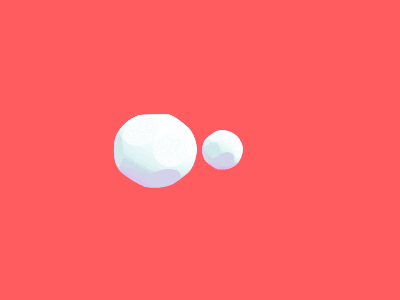
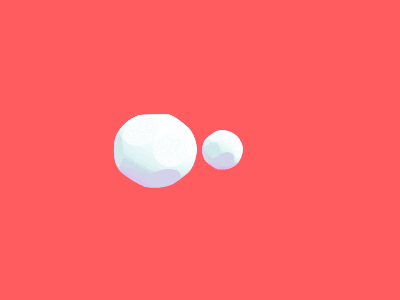
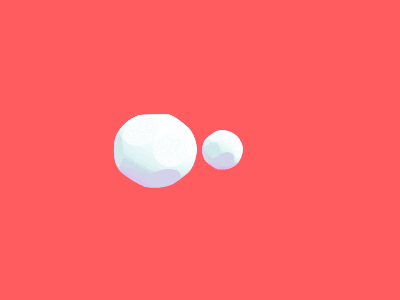
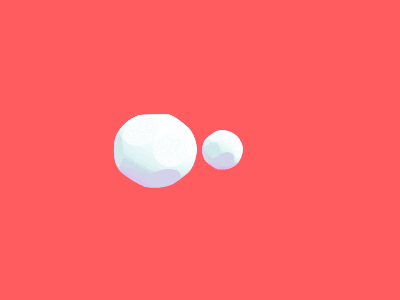
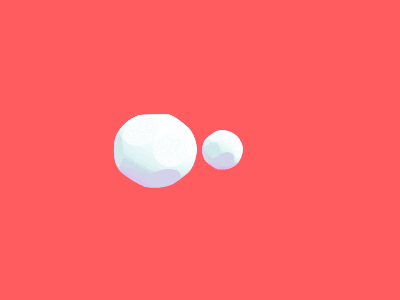
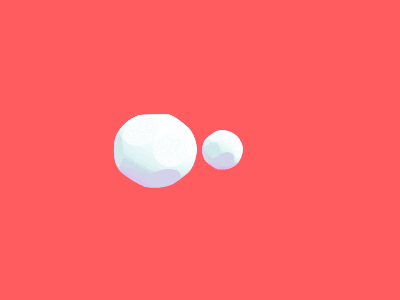