2022-08-09 更新
Keys to Better Image Inpainting: Structure and Texture Go Hand in Hand
Authors:Jitesh Jain, Yuqian Zhou, Ning Yu, Humphrey Shi
Deep image inpainting has made impressive progress with recent advances in image generation and processing algorithms. We claim that the performance of inpainting algorithms can be better judged by the generated structures and textures. Structures refer to the generated object boundary or novel geometric structures within the hole, while texture refers to high-frequency details, especially man-made repeating patterns filled inside the structural regions. We believe that better structures are usually obtained from a coarse-to-fine GAN-based generator network while repeating patterns nowadays can be better modeled using state-of-the-art high-frequency fast fourier convolutional layers. In this paper, we propose a novel inpainting network combining the advantages of the two designs. Therefore, our model achieves a remarkable visual quality to match state-of-the-art performance in both structure generation and repeating texture synthesis using a single network. Extensive experiments demonstrate the effectiveness of the method, and our conclusions further highlight the two critical factors of image inpainting quality, structures, and textures, as the future design directions of inpainting networks.
PDF Project page at https://praeclarumjj3.github.io/fcf-inpainting/
点此查看论文截图
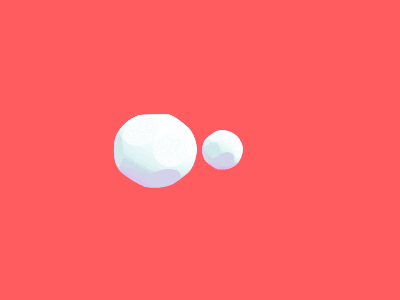
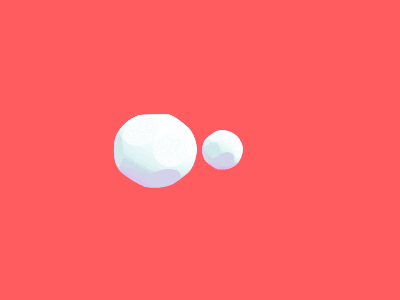
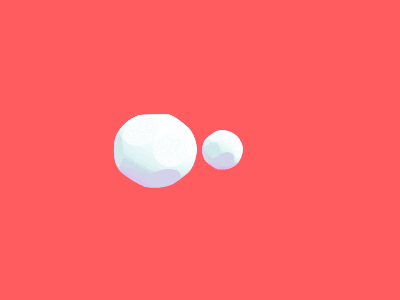
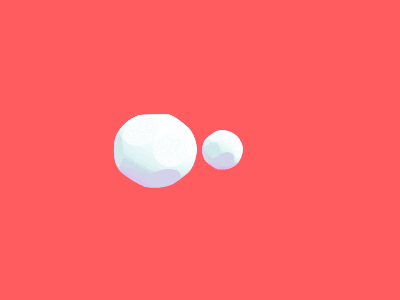
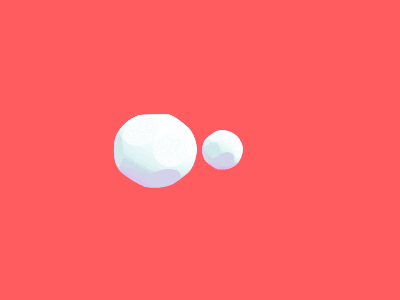
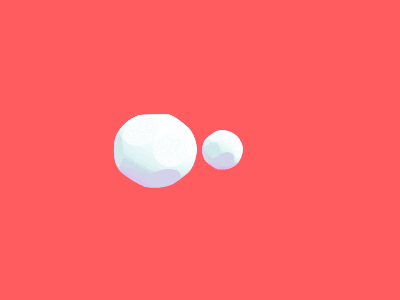
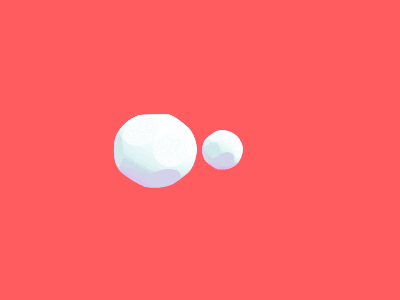
Towards Vivid and Diverse Image Colorization with Generative Color Prior
Authors:Yanze Wu, Xintao Wang, Yu Li, Honglun Zhang, Xun Zhao, Ying Shan
Colorization has attracted increasing interest in recent years. Classic reference-based methods usually rely on external color images for plausible results. A large image database or online search engine is inevitably required for retrieving such exemplars. Recent deep-learning-based methods could automatically colorize images at a low cost. However, unsatisfactory artifacts and incoherent colors are always accompanied. In this work, we propose GCP-Colorization that leverages the rich and diverse color priors encapsulated in a pretrained Generative Adversarial Networks (GAN) for automatic colorization. Specifically, we first “retrieve” matched features (similar to exemplars) via a GAN encoder and then incorporate these features into the colorization process with feature modulations. Thanks to the powerful generative color prior (GCP) and delicate designs, our GCP-Colorization could produce vivid colors with a single forward pass. Moreover, it is highly convenient to obtain diverse results by modifying GAN latent codes. GCP-Colorization also inherits the merit of interpretable controls of GANs and could attain controllable and smooth transitions by walking through GAN latent space. Extensive experiments and user studies demonstrate that GCP-Colorization achieves superior performance than previous works. Codes are available at https://github.com/ToTheBeginning/GCP-Colorization.
PDF ICCV 2021. Codes are available at https://github.com/ToTheBeginning/GCP-Colorization
点此查看论文截图
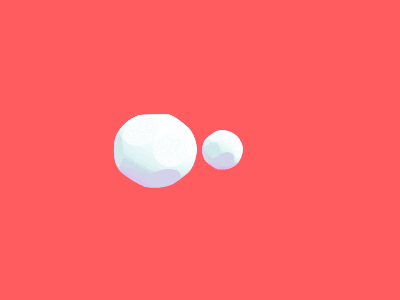
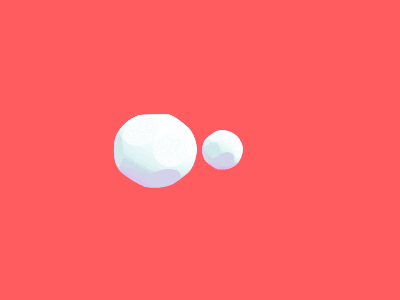
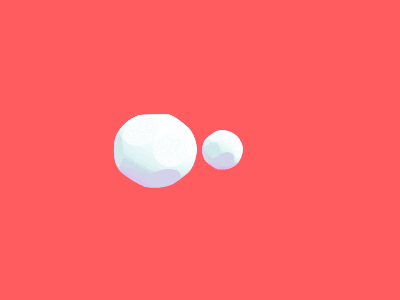
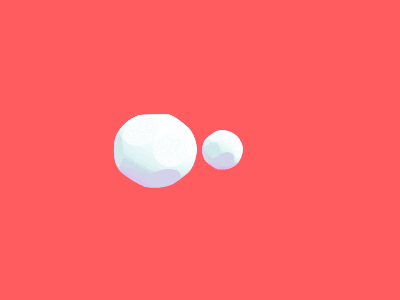
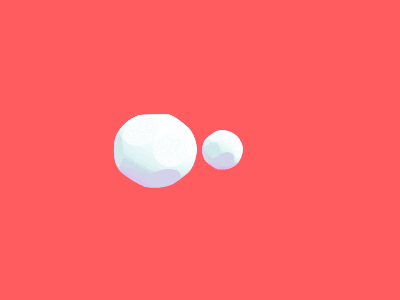
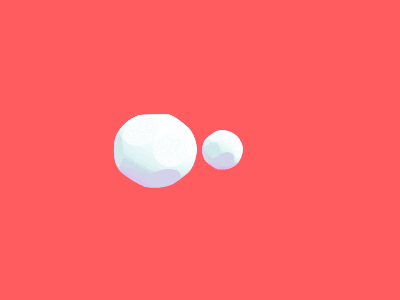
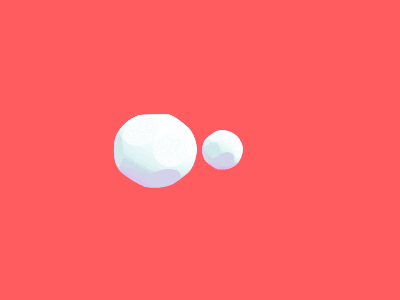
Hierarchical Semantic Regularization of Latent Spaces in StyleGANs
Authors:Tejan Karmali, Rishubh Parihar, Susmit Agrawal, Harsh Rangwani, Varun Jampani, Maneesh Singh, R. Venkatesh Babu
Progress in GANs has enabled the generation of high-resolution photorealistic images of astonishing quality. StyleGANs allow for compelling attribute modification on such images via mathematical operations on the latent style vectors in the W/W+ space that effectively modulate the rich hierarchical representations of the generator. Such operations have recently been generalized beyond mere attribute swapping in the original StyleGAN paper to include interpolations. In spite of many significant improvements in StyleGANs, they are still seen to generate unnatural images. The quality of the generated images is predicated on two assumptions; (a) The richness of the hierarchical representations learnt by the generator, and, (b) The linearity and smoothness of the style spaces. In this work, we propose a Hierarchical Semantic Regularizer (HSR) which aligns the hierarchical representations learnt by the generator to corresponding powerful features learnt by pretrained networks on large amounts of data. HSR is shown to not only improve generator representations but also the linearity and smoothness of the latent style spaces, leading to the generation of more natural-looking style-edited images. To demonstrate improved linearity, we propose a novel metric - Attribute Linearity Score (ALS). A significant reduction in the generation of unnatural images is corroborated by improvement in the Perceptual Path Length (PPL) metric by 16.19% averaged across different standard datasets while simultaneously improving the linearity of attribute-change in the attribute editing tasks.
PDF ECCV 2022. Project page: https://sites.google.com/view/hsr-eccv22/
点此查看论文截图
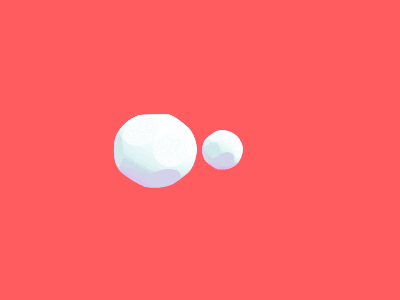
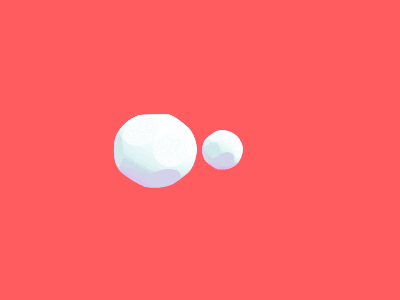
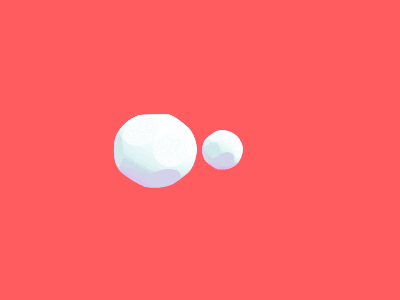
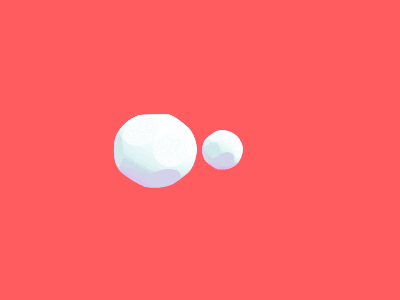
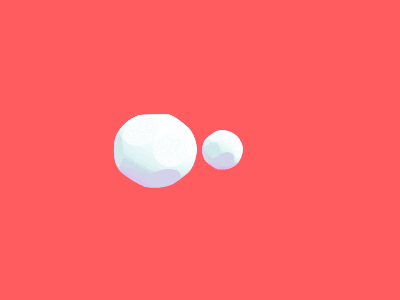
Perceptual Artifacts Localization for Inpainting
Authors:Lingzhi Zhang, Yuqian Zhou, Connelly Barnes, Sohrab Amirghodsi, Zhe Lin, Eli Shechtman, Jianbo Shi
Image inpainting is an essential task for multiple practical applications like object removal and image editing. Deep GAN-based models greatly improve the inpainting performance in structures and textures within the hole, but might also generate unexpected artifacts like broken structures or color blobs. Users perceive these artifacts to judge the effectiveness of inpainting models, and retouch these imperfect areas to inpaint again in a typical retouching workflow. Inspired by this workflow, we propose a new learning task of automatic segmentation of inpainting perceptual artifacts, and apply the model for inpainting model evaluation and iterative refinement. Specifically, we first construct a new inpainting artifacts dataset by manually annotating perceptual artifacts in the results of state-of-the-art inpainting models. Then we train advanced segmentation networks on this dataset to reliably localize inpainting artifacts within inpainted images. Second, we propose a new interpretable evaluation metric called Perceptual Artifact Ratio (PAR), which is the ratio of objectionable inpainted regions to the entire inpainted area. PAR demonstrates a strong correlation with real user preference. Finally, we further apply the generated masks for iterative image inpainting by combining our approach with multiple recent inpainting methods. Extensive experiments demonstrate the consistent decrease of artifact regions and inpainting quality improvement across the different methods.
PDF
点此查看论文截图
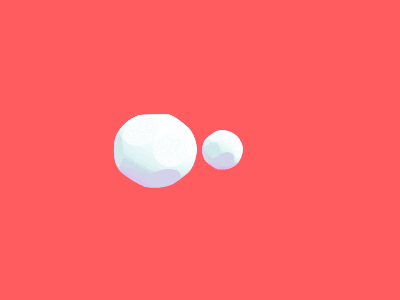
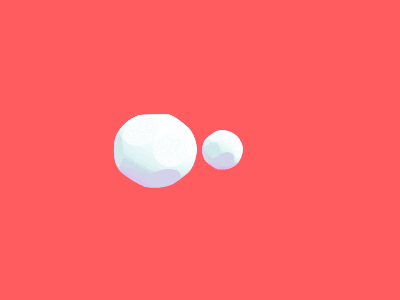
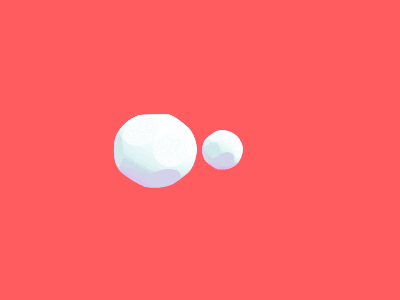
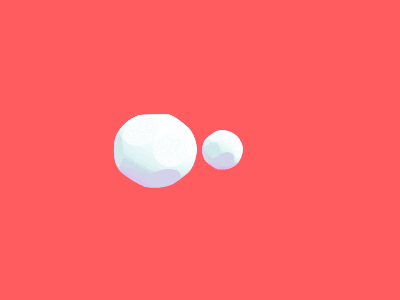
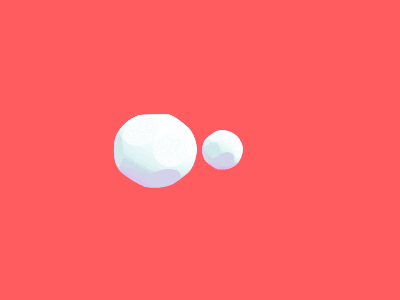