2022-08-02 更新
Development of a face mask detection pipeline for mask-wearing monitoring in the era of the COVID-19 pandemic: A modular approach
Authors:Benjaphan Sommana, Ukrit Watchareeruetai, Ankush Ganguly, Samuel W. F. Earp, Taya Kitiyakara, Suparee Boonmanunt, Ratchainant Thammasudjarit
During the SARS-Cov-2 pandemic, mask-wearing became an effective tool to prevent spreading and contracting the virus. The ability to monitor the mask-wearing rate in the population would be useful for determining public health strategies against the virus. However, artificial intelligence technologies for detecting face masks have not been deployed at a large scale in real-life to measure the mask-wearing rate in public. In this paper, we present a two-step face mask detection approach consisting of two separate modules: 1) face detection and alignment and 2) face mask classification. This approach allowed us to experiment with different combinations of face detection and face mask classification modules. More specifically, we experimented with PyramidKey and RetinaFace as face detectors while maintaining a lightweight backbone for the face mask classification module. Moreover, we also provide a relabeled annotation of the test set of the AIZOO dataset, where we rectified the incorrect labels for some face images. The evaluation results on the AIZOO and Moxa 3K datasets showed that the proposed face mask detection pipeline surpassed the state-of-the-art methods. The proposed pipeline also yielded a higher mAP on the relabeled test set of the AIZOO dataset than the original test set. Since we trained the proposed model using in-the-wild face images, we can successfully deploy our model to monitor the mask-wearing rate using public CCTV images.
PDF Accepted at the 19th International Joint Conference on Computer Science and Software Engineering (JCSSE 2022)
点此查看论文截图
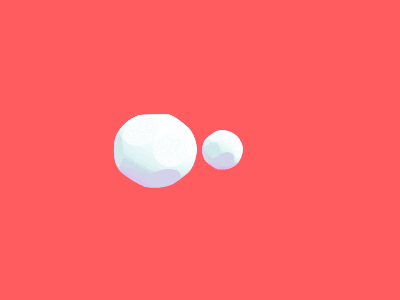
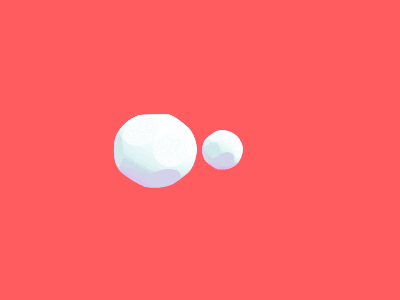
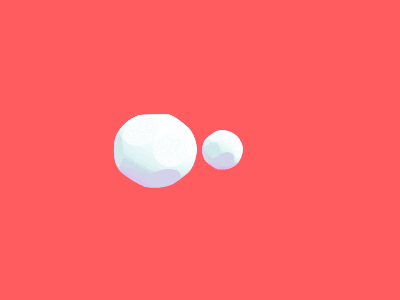
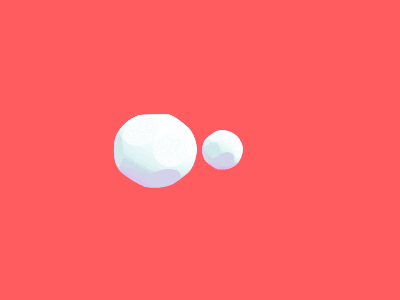
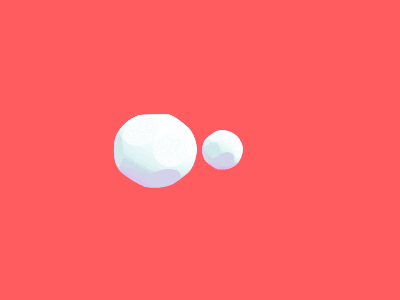
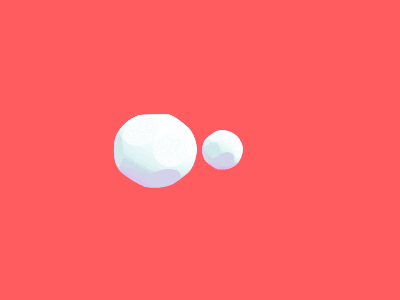