2022-07-30 更新
Bi-directional Contrastive Learning for Domain Adaptive Semantic Segmentation
Authors:Geon Lee, Chanho Eom, Wonkyung Lee, Hyekang Park, Bumsub Ham
We present a novel unsupervised domain adaptation method for semantic segmentation that generalizes a model trained with source images and corresponding ground-truth labels to a target domain. A key to domain adaptive semantic segmentation is to learn domain-invariant and discriminative features without target ground-truth labels. To this end, we propose a bi-directional pixel-prototype contrastive learning framework that minimizes intra-class variations of features for the same object class, while maximizing inter-class variations for different ones, regardless of domains. Specifically, our framework aligns pixel-level features and a prototype of the same object class in target and source images (i.e., positive pairs), respectively, sets them apart for different classes (i.e., negative pairs), and performs the alignment and separation processes toward the other direction with pixel-level features in the source image and a prototype in the target image. The cross-domain matching encourages domain-invariant feature representations, while the bidirectional pixel-prototype correspondences aggregate features for the same object class, providing discriminative features. To establish training pairs for contrastive learning, we propose to generate dynamic pseudo labels of target images using a non-parametric label transfer, that is, pixel-prototype correspondences across different domains. We also present a calibration method compensating class-wise domain biases of prototypes gradually during training.
PDF Accepted to ECCV 2022
点此查看论文截图
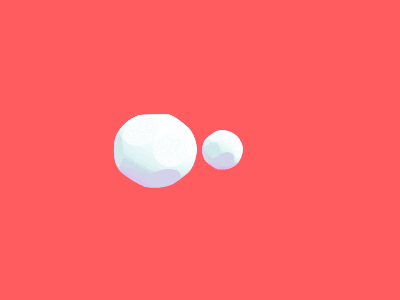
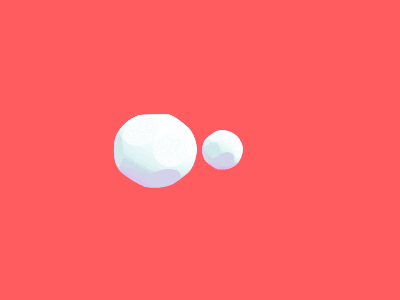
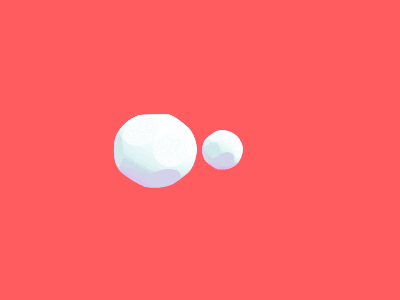
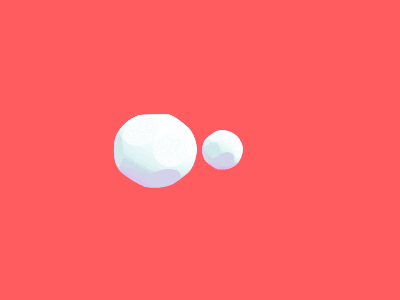
Optimizing transformations for contrastive learning in a differentiable framework
Authors:Camille Ruppli, Pietro Gori, Roberto Ardon, Isabelle Bloch
Current contrastive learning methods use random transformations sampled from a large list of transformations, with fixed hyperparameters, to learn invariance from an unannotated database. Following previous works that introduce a small amount of supervision, we propose a framework to find optimal transformations for contrastive learning using a differentiable transformation network. Our method increases performances at low annotated data regime both in supervision accuracy and in convergence speed. In contrast to previous work, no generative model is needed for transformation optimization. Transformed images keep relevant information to solve the supervised task, here classification. Experiments were performed on 34000 2D slices of brain Magnetic Resonance Images and 11200 chest X-ray images. On both datasets, with 10% of labeled data, our model achieves better performances than a fully supervised model with 100% labels.
PDF Accepted at MILLanD workshop (MICCAI)
点此查看论文截图
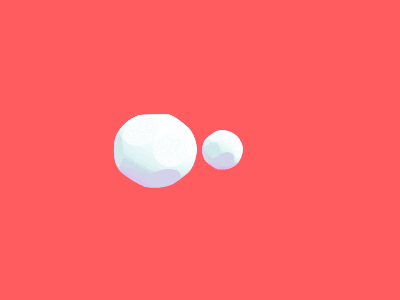
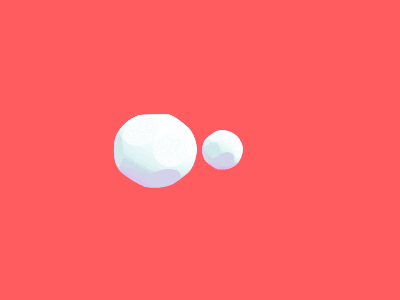
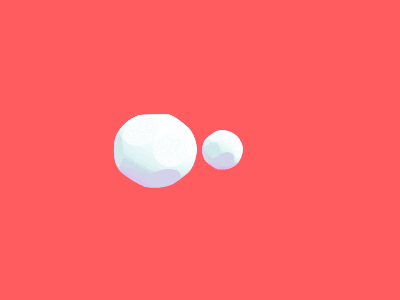
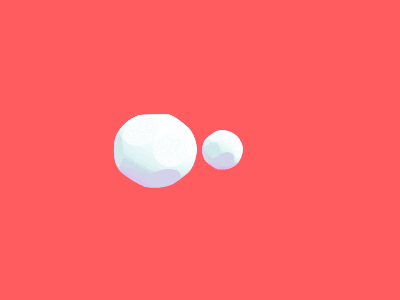
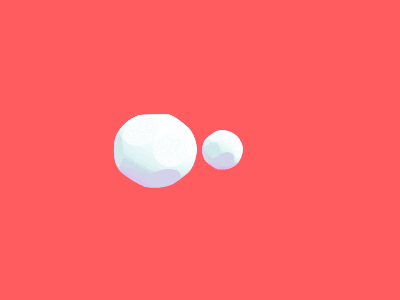
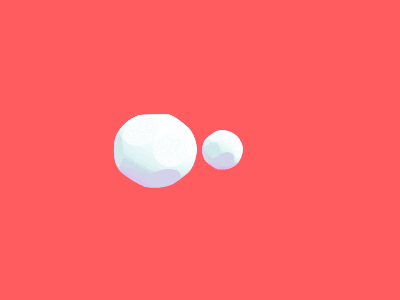